Compare commits
199 Commits
Author | SHA1 | Date | |
---|---|---|---|
8ad19e115c | |||
250752c620 | |||
|
5e5f21f90e | ||
|
017190ccee | ||
|
c485502cb5 | ||
|
e9136d120c | ||
|
f88cd90ef3 | ||
|
b52be94d76 | ||
|
ed3c55ce9a | ||
|
9ff29cd391 | ||
|
54f358c51c | ||
|
f05a4acb04 | ||
|
3488d22d22 | ||
|
6b4381ee77 | ||
|
1b3aa629da | ||
|
79476f66a6 | ||
|
ef4b82a91d | ||
|
58d81f095c | ||
|
d66fd89947 | ||
|
b24a18cd3a | ||
|
e1c12202aa | ||
|
bfbf43f45c | ||
|
cc8b22f0fb | ||
|
a2bbbabee2 | ||
|
b52873cb37 | ||
|
00d82154dc | ||
|
440b70eb15 | ||
|
2a55c8256d | ||
|
2ddcd17d23 | ||
|
14461930ab | ||
|
79eff01b33 | ||
|
b19ea95f88 | ||
|
4f92366ea5 | ||
|
235b587789 | ||
|
c6a4a71cf1 | ||
|
150bb089cf | ||
|
5c8a637cf5 | ||
|
6c7b40a9c1 | ||
|
d075d6377e | ||
|
ae1d01bd0c | ||
|
aae7cfe1a2 | ||
|
38b33a7030 | ||
|
70236df3d1 | ||
|
40c5368deb | ||
|
2d853f92b9 | ||
|
2a0ad19bc5 | ||
|
5deb115625 | ||
|
7f329702ad | ||
|
ff6240d798 | ||
|
f6614ff4dc | ||
|
8633134de7 | ||
|
b24be3baec | ||
|
2818700182 | ||
|
5f637dc4c7 | ||
|
b7aba9c8de | ||
|
e4d440404a | ||
|
e332224c24 | ||
|
288724adef | ||
|
a15c4bdf63 | ||
|
253568ef29 | ||
|
0ab248c478 | ||
|
3cef51144f | ||
|
08bc342fd6 | ||
|
c2799c9494 | ||
|
d6b536ace9 | ||
|
edf55843e4 | ||
|
d0ab9c7ec4 | ||
|
16f2201d9f | ||
|
a93610e574 | ||
|
1d5d012ce4 | ||
|
0e4b6cbd15 | ||
|
2f777f1286 | ||
|
d2f56631ee | ||
|
c5077f4ebc | ||
|
acf5d02104 | ||
|
bf58841f00 | ||
|
e625e1f783 | ||
|
4bed070556 | ||
|
5692579f56 | ||
|
333619839a | ||
|
c6024520af | ||
|
cd40261de6 | ||
|
3a637a973c | ||
|
7fbcb5e810 | ||
|
2604d3c47b | ||
|
bb1a6191b0 | ||
|
dd89041f72 | ||
|
91eb72e515 | ||
|
1c7436c34b | ||
|
8678f376e9 | ||
|
050154f406 | ||
|
b3eae8bcfa | ||
|
c720362886 | ||
|
93029d3f5c | ||
|
28244a57b4 | ||
|
f6ba9d7451 | ||
|
96e431e06b | ||
|
cb6ddb3674 | ||
|
07d4ba0d6b | ||
|
ac139d5bda | ||
|
14acfc1d81 | ||
|
2947162cc4 | ||
|
4f14074a75 | ||
|
53a5574080 | ||
|
d91c3c004d | ||
|
c90cefc453 | ||
|
b8abd2fef3 | ||
|
887ba06bd6 | ||
|
c9513822c9 | ||
|
e3baa0da86 | ||
|
ba9aab920e | ||
|
b0f2ef65d9 | ||
|
c13b28561d | ||
|
5c88ccd9e6 | ||
|
e0a6a279b3 | ||
|
9bb3a90977 | ||
|
02bbd18acf | ||
|
18ab8b141f | ||
|
225abc5202 | ||
|
d33dff7723 | ||
|
771027211a | ||
|
94fe71b49c | ||
|
fafd9f7f6e | ||
|
85b10993ec | ||
|
11f1d66383 | ||
|
38e89aec18 | ||
|
3e336830a3 | ||
|
a1ae71d221 | ||
|
0703993bfd | ||
|
50a666a350 | ||
|
9ea86ee4b1 | ||
|
94580f825e | ||
|
d5cca4e542 | ||
|
f1986fa9d0 | ||
|
1c025c3d29 | ||
|
4added7390 | ||
|
ee5cca3ff3 | ||
|
0da92ec7bf | ||
|
e3e075e432 | ||
|
19eeeab1e1 | ||
|
78238c24cf | ||
|
932281db0a | ||
|
843840baa0 | ||
|
7cba526913 | ||
|
7fe70c949e | ||
|
1c1c9e2c5f | ||
|
26c2954c8e | ||
|
5329537a2f | ||
|
e07f0fa6e3 | ||
|
b077f1fe42 | ||
|
5f94d86558 | ||
|
947e127e34 | ||
|
95502b900d | ||
|
16b636ef83 | ||
|
4339ce20d5 | ||
|
c31fc22b6b | ||
|
7f49c6025b | ||
|
2d4f436ebf | ||
|
549f32a743 | ||
|
e3b3452a73 | ||
|
62350d975d | ||
|
8d84b326b8 | ||
|
16079a3cba | ||
|
ff330a5487 | ||
|
94b3882d30 | ||
|
81544ca8b3 | ||
|
b7f4dd835e | ||
|
7e2380e4ed | ||
|
7f3cfd54b0 | ||
|
e083f2c629 | ||
|
e33858f110 | ||
|
da01a33152 | ||
|
8ca920a114 | ||
|
5f3d449a66 | ||
|
13735e7dfb | ||
|
a38d5c3a25 | ||
|
5bae637c67 | ||
|
12e488ba80 | ||
|
ad30c63c69 | ||
|
a116eff7df | ||
|
01bc355dde | ||
|
8e05f3c360 | ||
|
fde988dd4e | ||
|
91401ad14f | ||
|
280194647c | ||
|
2e0a542f33 | ||
|
b988694da7 | ||
|
512c4d0f73 | ||
|
5525fb1470 | ||
|
4db735e026 | ||
|
c8c79c39d1 | ||
|
bcfb76d8ca | ||
|
2d9aaf8fc9 | ||
|
8a3905c09a | ||
|
54cd8a46fa | ||
|
1b83bf261a | ||
|
2a7d22dab1 | ||
|
f7494b0cfb | ||
|
9ca91d59ec |
2
.gitattributes
vendored
2
.gitattributes
vendored
@ -1,3 +1,5 @@
|
||||
* text=auto eol=lf
|
||||
|
||||
backend-python/rwkv_pip/** linguist-vendored
|
||||
backend-python/wkv_cuda_utils/** linguist-vendored
|
||||
backend-python/get-pip.py linguist-vendored
|
||||
|
9
.github/dependabot.yml
vendored
Normal file
9
.github/dependabot.yml
vendored
Normal file
@ -0,0 +1,9 @@
|
||||
version: 2
|
||||
updates:
|
||||
- package-ecosystem: "github-actions"
|
||||
directory: "/"
|
||||
schedule:
|
||||
interval: "weekly"
|
||||
commit-message:
|
||||
prefix: "chore"
|
||||
include: "scope"
|
171
.github/workflows/docker.yml
vendored
Normal file
171
.github/workflows/docker.yml
vendored
Normal file
@ -0,0 +1,171 @@
|
||||
name: Publish Docker Image
|
||||
on: [push]
|
||||
|
||||
concurrency:
|
||||
group: ${{ github.ref }}-${{ github.workflow }}
|
||||
cancel-in-progress: true
|
||||
|
||||
jobs:
|
||||
docker_build:
|
||||
name: Build ${{ matrix.arch }} Image
|
||||
runs-on: ubuntu-latest
|
||||
|
||||
strategy:
|
||||
matrix:
|
||||
include:
|
||||
- arch: amd64
|
||||
name: amd64
|
||||
# - arch: arm64
|
||||
# name: arm64
|
||||
|
||||
steps:
|
||||
- name: Free up disk spaces
|
||||
run: |
|
||||
sudo rm -rf /usr/share/dotnet || true
|
||||
sudo rm -rf /opt/ghc || true
|
||||
sudo rm -rf "/usr/local/share/boost" || true
|
||||
sudo rm -rf "$AGENT_TOOLSDIRECTORY" || true
|
||||
|
||||
- name: Get lowercase string for the repository name
|
||||
id: lowercase-repo-name
|
||||
uses: ASzc/change-string-case-action@v2
|
||||
with:
|
||||
string: ${{ github.event.repository.name }}
|
||||
|
||||
- name: Checkout base
|
||||
uses: actions/checkout@v2
|
||||
with:
|
||||
fetch-depth: 0
|
||||
|
||||
- name: Cache Docker layers
|
||||
uses: actions/cache@v2
|
||||
with:
|
||||
path: /tmp/.buildx-cache
|
||||
key: ${{ github.ref }}-${{ matrix.arch }}
|
||||
restore-keys: |
|
||||
${{ github.ref }}-${{ matrix.arch }}
|
||||
|
||||
- name: Set up QEMU
|
||||
uses: docker/setup-qemu-action@v2
|
||||
with:
|
||||
platforms: linux/${{ matrix.arch }}
|
||||
|
||||
- name: Set up Docker Buildx
|
||||
uses: docker/setup-buildx-action@v2
|
||||
|
||||
- name: Docker login
|
||||
uses: docker/login-action@v2
|
||||
with:
|
||||
username: ${{ secrets.DOCKER_USERNAME }}
|
||||
password: ${{ secrets.DOCKER_PASSWORD }}
|
||||
|
||||
- name: Get commit SHA
|
||||
id: vars
|
||||
run: echo "::set-output name=sha_short::$(git rev-parse --short HEAD)"
|
||||
|
||||
- name: Build and export
|
||||
id: build
|
||||
if: github.ref == 'refs/heads/master'
|
||||
uses: docker/build-push-action@v3
|
||||
with:
|
||||
push: true
|
||||
platforms: linux/${{ matrix.arch }}
|
||||
tags: ${{ secrets.DOCKER_USERNAME }}/${{ steps.lowercase-repo-name.outputs.lowercase }}:${{ matrix.name }}-latest
|
||||
build-args: |
|
||||
SHA=${{ steps.vars.outputs.sha_short }}
|
||||
outputs: type=image,push=true
|
||||
cache-from: type=local,src=/tmp/.buildx-cache
|
||||
cache-to: type=local,dest=/tmp/.buildx-cache
|
||||
|
||||
- name: Replace tag without `v`
|
||||
if: startsWith(github.ref, 'refs/tags/')
|
||||
uses: actions/github-script@v1
|
||||
id: version
|
||||
with:
|
||||
script: |
|
||||
return context.payload.ref.replace(/\/?refs\/tags\/v/, '')
|
||||
result-encoding: string
|
||||
|
||||
- name: Build release and export
|
||||
id: build_rel
|
||||
if: startsWith(github.ref, 'refs/tags/')
|
||||
uses: docker/build-push-action@v3
|
||||
with:
|
||||
push: true
|
||||
platforms: linux/${{ matrix.arch }}
|
||||
tags: ${{ secrets.DOCKER_USERNAME }}/${{ steps.lowercase-repo-name.outputs.lowercase }}:${{ matrix.name }}-${{steps.version.outputs.result}}
|
||||
build-args: |
|
||||
SHA=${{ steps.version.outputs.result }}
|
||||
outputs: type=image,push=true
|
||||
cache-from: type=local,src=/tmp/.buildx-cache
|
||||
cache-to: type=local,dest=/tmp/.buildx-cache
|
||||
|
||||
- name: Save digest
|
||||
if: github.ref == 'refs/heads/master'
|
||||
run: echo ${{ steps.build.outputs.digest }} > /tmp/digest.txt
|
||||
|
||||
- name: Save release digest
|
||||
if: startsWith(github.ref, 'refs/tags/')
|
||||
run: echo ${{ steps.build_rel.outputs.digest }} > /tmp/digest.txt
|
||||
|
||||
- name: Upload artifact
|
||||
uses: actions/upload-artifact@v3
|
||||
with:
|
||||
name: digest_${{ matrix.name }}
|
||||
path: /tmp/digest.txt
|
||||
|
||||
manifests:
|
||||
name: Build manifests
|
||||
needs: [docker_build]
|
||||
runs-on: ubuntu-latest
|
||||
steps:
|
||||
- name: Get lowercase string for the repository name
|
||||
id: lowercase-repo-name
|
||||
uses: ASzc/change-string-case-action@v2
|
||||
with:
|
||||
string: ${{ github.event.repository.name }}
|
||||
|
||||
- name: Checkout base
|
||||
uses: actions/checkout@v2
|
||||
with:
|
||||
fetch-depth: 0
|
||||
|
||||
# https://github.com/docker/setup-qemu-action
|
||||
- name: Set up QEMU
|
||||
uses: docker/setup-qemu-action@v2
|
||||
|
||||
# https://github.com/docker/setup-buildx-action
|
||||
- name: Set up Docker Buildx
|
||||
uses: docker/setup-buildx-action@v2
|
||||
with:
|
||||
config-inline: |
|
||||
[worker.oci]
|
||||
max-parallelism = 1
|
||||
|
||||
- name: Download artifact
|
||||
uses: actions/download-artifact@v3
|
||||
with:
|
||||
path: /tmp/images/
|
||||
|
||||
- name: Docker login
|
||||
uses: docker/login-action@v2
|
||||
with:
|
||||
username: ${{ secrets.DOCKER_USERNAME }}
|
||||
password: ${{ secrets.DOCKER_PASSWORD }}
|
||||
|
||||
- name: Replace tag without `v`
|
||||
if: startsWith(github.ref, 'refs/tags/')
|
||||
uses: actions/github-script@v1
|
||||
id: version
|
||||
with:
|
||||
script: |
|
||||
return context.payload.ref.replace(/\/?refs\/tags\/v/, '')
|
||||
result-encoding: string
|
||||
|
||||
- name: Merge and push manifest on master branch
|
||||
if: github.ref == 'refs/heads/master'
|
||||
run: python scripts/merge_manifest.py "${{ secrets.DOCKER_USERNAME }}/${{ steps.lowercase-repo-name.outputs.lowercase }}"
|
||||
|
||||
- name: Merge and push manifest on release
|
||||
if: startsWith(github.ref, 'refs/tags/')
|
||||
run: python scripts/merge_manifest.py "${{ secrets.DOCKER_USERNAME }}/${{ steps.lowercase-repo-name.outputs.lowercase }}" ${{steps.version.outputs.result}}
|
114
.github/workflows/pre-release.yml
vendored
Normal file
114
.github/workflows/pre-release.yml
vendored
Normal file
@ -0,0 +1,114 @@
|
||||
name: pre-release
|
||||
on:
|
||||
workflow_dispatch:
|
||||
push:
|
||||
branches:
|
||||
- master
|
||||
paths:
|
||||
- "backend-python/**"
|
||||
tags-ignore:
|
||||
- "v*"
|
||||
|
||||
jobs:
|
||||
windows:
|
||||
runs-on: windows-2022
|
||||
steps:
|
||||
- uses: actions/checkout@v4
|
||||
with:
|
||||
ref: master
|
||||
- uses: actions/setup-go@v5
|
||||
with:
|
||||
go-version: "1.20.5"
|
||||
- uses: actions/setup-python@v5
|
||||
id: cp310
|
||||
with:
|
||||
python-version: "3.10"
|
||||
- uses: crazy-max/ghaction-chocolatey@v3
|
||||
with:
|
||||
args: install upx
|
||||
- run: |
|
||||
Start-BitsTransfer https://github.com/josStorer/ai00_rwkv_server/releases/latest/download/webgpu_server_windows_x86_64.exe ./backend-rust/webgpu_server.exe
|
||||
Start-BitsTransfer https://github.com/josStorer/web-rwkv-converter/releases/latest/download/web-rwkv-converter_windows_x86_64.exe ./backend-rust/web-rwkv-converter.exe
|
||||
Start-BitsTransfer https://github.com/josStorer/LibreHardwareMonitor.Console/releases/latest/download/LibreHardwareMonitor.Console.zip ./LibreHardwareMonitor.Console.zip
|
||||
Expand-Archive ./LibreHardwareMonitor.Console.zip -DestinationPath ./components/LibreHardwareMonitor.Console
|
||||
Start-BitsTransfer https://www.python.org/ftp/python/3.10.11/python-3.10.11-embed-amd64.zip ./python-3.10.11-embed-amd64.zip
|
||||
Expand-Archive ./python-3.10.11-embed-amd64.zip -DestinationPath ./py310
|
||||
$content=Get-Content "./py310/python310._pth"; $content | ForEach-Object {if ($_.ReadCount -eq 3) {"Lib\\site-packages"} else {$_}} | Set-Content ./py310/python310._pth
|
||||
./py310/python ./backend-python/get-pip.py
|
||||
./py310/python -m pip install Cython==3.0.4
|
||||
Copy-Item -Path "${{ steps.cp310.outputs.python-path }}/../include" -Destination "py310/include" -Recurse
|
||||
Copy-Item -Path "${{ steps.cp310.outputs.python-path }}/../libs" -Destination "py310/libs" -Recurse
|
||||
./py310/python -m pip install cyac==1.9
|
||||
go install github.com/wailsapp/wails/v2/cmd/wails@v2.8.0
|
||||
del ./backend-python/rwkv_pip/cpp/librwkv.dylib
|
||||
del ./backend-python/rwkv_pip/cpp/librwkv.so
|
||||
(Get-Content -Path ./backend-golang/app.go) -replace "//go:custom_build windows ", "" | Set-Content -Path ./backend-golang/app.go
|
||||
(Get-Content -Path ./backend-golang/utils.go) -replace "//go:custom_build windows ", "" | Set-Content -Path ./backend-golang/utils.go
|
||||
make
|
||||
Rename-Item -Path "build/bin/RWKV-Runner.exe" -NewName "RWKV-Runner_windows_x64.exe"
|
||||
|
||||
- uses: actions/upload-artifact@v4
|
||||
with:
|
||||
name: RWKV-Runner_windows_x64.exe
|
||||
path: build/bin/RWKV-Runner_windows_x64.exe
|
||||
|
||||
linux:
|
||||
runs-on: ubuntu-20.04
|
||||
steps:
|
||||
- uses: actions/checkout@v4
|
||||
with:
|
||||
ref: master
|
||||
- uses: actions/setup-go@v5
|
||||
with:
|
||||
go-version: "1.20.5"
|
||||
- run: |
|
||||
wget https://github.com/josStorer/ai00_rwkv_server/releases/latest/download/webgpu_server_linux_x86_64 -O ./backend-rust/webgpu_server
|
||||
wget https://github.com/josStorer/web-rwkv-converter/releases/latest/download/web-rwkv-converter_linux_x86_64 -O ./backend-rust/web-rwkv-converter
|
||||
sudo apt-get update
|
||||
sudo apt-get install upx
|
||||
sudo apt-get install build-essential libgtk-3-dev libwebkit2gtk-4.0-dev libasound2-dev
|
||||
go install github.com/wailsapp/wails/v2/cmd/wails@v2.8.0
|
||||
rm ./backend-python/rwkv_pip/wkv_cuda.pyd
|
||||
rm ./backend-python/rwkv_pip/rwkv5.pyd
|
||||
rm ./backend-python/rwkv_pip/rwkv6.pyd
|
||||
rm ./backend-python/get-pip.py
|
||||
rm ./backend-python/rwkv_pip/cpp/librwkv.dylib
|
||||
rm ./backend-python/rwkv_pip/cpp/rwkv.dll
|
||||
rm ./backend-python/rwkv_pip/webgpu/web_rwkv_py.cp310-win_amd64.pyd
|
||||
make
|
||||
mv build/bin/RWKV-Runner build/bin/RWKV-Runner_linux_x64
|
||||
|
||||
- uses: actions/upload-artifact@v4
|
||||
with:
|
||||
name: RWKV-Runner_linux_x64
|
||||
path: build/bin/RWKV-Runner_linux_x64
|
||||
|
||||
macos:
|
||||
runs-on: macos-13
|
||||
steps:
|
||||
- uses: actions/checkout@v4
|
||||
with:
|
||||
ref: master
|
||||
- uses: actions/setup-go@v5
|
||||
with:
|
||||
go-version: "1.20.5"
|
||||
- run: |
|
||||
wget https://github.com/josStorer/ai00_rwkv_server/releases/latest/download/webgpu_server_darwin_aarch64 -O ./backend-rust/webgpu_server
|
||||
wget https://github.com/josStorer/web-rwkv-converter/releases/latest/download/web-rwkv-converter_darwin_aarch64 -O ./backend-rust/web-rwkv-converter
|
||||
go install github.com/wailsapp/wails/v2/cmd/wails@v2.8.0
|
||||
rm ./backend-python/rwkv_pip/wkv_cuda.pyd
|
||||
rm ./backend-python/rwkv_pip/rwkv5.pyd
|
||||
rm ./backend-python/rwkv_pip/rwkv6.pyd
|
||||
rm ./backend-python/get-pip.py
|
||||
rm ./backend-python/rwkv_pip/cpp/rwkv.dll
|
||||
rm ./backend-python/rwkv_pip/cpp/librwkv.so
|
||||
rm ./backend-python/rwkv_pip/webgpu/web_rwkv_py.cp310-win_amd64.pyd
|
||||
make
|
||||
cp build/darwin/Readme_Install.txt build/bin/Readme_Install.txt
|
||||
cp build/bin/RWKV-Runner.app/Contents/MacOS/RWKV-Runner build/bin/RWKV-Runner_darwin_universal
|
||||
cd build/bin && zip -r RWKV-Runner_macos_universal.zip RWKV-Runner.app Readme_Install.txt
|
||||
|
||||
- uses: actions/upload-artifact@v4
|
||||
with:
|
||||
name: RWKV-Runner_macos_universal.zip
|
||||
path: build/bin/RWKV-Runner_macos_universal.zip
|
38
.github/workflows/release.yml
vendored
38
.github/workflows/release.yml
vendored
@ -14,11 +14,11 @@ jobs:
|
||||
runs-on: ubuntu-22.04
|
||||
steps:
|
||||
- run: echo "VERSION=${GITHUB_REF_NAME#v}" >> $GITHUB_ENV
|
||||
- uses: actions/checkout@v3
|
||||
- uses: actions/checkout@v4
|
||||
with:
|
||||
ref: master
|
||||
|
||||
- uses: jossef/action-set-json-field@v2.1
|
||||
- uses: jossef/action-set-json-field@v2.2
|
||||
with:
|
||||
file: manifest.json
|
||||
field: version
|
||||
@ -38,17 +38,17 @@ jobs:
|
||||
runs-on: windows-2022
|
||||
needs: create-draft
|
||||
steps:
|
||||
- uses: actions/checkout@v3
|
||||
- uses: actions/checkout@v4
|
||||
with:
|
||||
ref: master
|
||||
- uses: actions/setup-go@v4
|
||||
- uses: actions/setup-go@v5
|
||||
with:
|
||||
go-version: '1.20.5'
|
||||
- uses: actions/setup-python@v4
|
||||
go-version: "1.20.5"
|
||||
- uses: actions/setup-python@v5
|
||||
id: cp310
|
||||
with:
|
||||
python-version: '3.10'
|
||||
- uses: crazy-max/ghaction-chocolatey@v2
|
||||
python-version: "3.10"
|
||||
- uses: crazy-max/ghaction-chocolatey@v3
|
||||
with:
|
||||
args: install upx
|
||||
- run: |
|
||||
@ -64,7 +64,7 @@ jobs:
|
||||
Copy-Item -Path "${{ steps.cp310.outputs.python-path }}/../include" -Destination "py310/include" -Recurse
|
||||
Copy-Item -Path "${{ steps.cp310.outputs.python-path }}/../libs" -Destination "py310/libs" -Recurse
|
||||
./py310/python -m pip install cyac==1.9
|
||||
go install github.com/wailsapp/wails/v2/cmd/wails@latest
|
||||
go install github.com/wailsapp/wails/v2/cmd/wails@v2.8.0
|
||||
del ./backend-python/rwkv_pip/cpp/librwkv.dylib
|
||||
del ./backend-python/rwkv_pip/cpp/librwkv.so
|
||||
(Get-Content -Path ./backend-golang/app.go) -replace "//go:custom_build windows ", "" | Set-Content -Path ./backend-golang/app.go
|
||||
@ -78,23 +78,22 @@ jobs:
|
||||
runs-on: ubuntu-20.04
|
||||
needs: create-draft
|
||||
steps:
|
||||
- uses: actions/checkout@v3
|
||||
- uses: actions/checkout@v4
|
||||
with:
|
||||
ref: master
|
||||
- uses: actions/setup-go@v4
|
||||
- uses: actions/setup-go@v5
|
||||
with:
|
||||
go-version: '1.20.5'
|
||||
go-version: "1.20.5"
|
||||
- run: |
|
||||
wget https://github.com/josStorer/ai00_rwkv_server/releases/latest/download/webgpu_server_linux_x86_64 -O ./backend-rust/webgpu_server
|
||||
wget https://github.com/josStorer/web-rwkv-converter/releases/latest/download/web-rwkv-converter_linux_x86_64 -O ./backend-rust/web-rwkv-converter
|
||||
sudo apt-get update
|
||||
sudo apt-get install upx
|
||||
sudo apt-get install build-essential libgtk-3-dev libwebkit2gtk-4.0-dev libasound2-dev
|
||||
go install github.com/wailsapp/wails/v2/cmd/wails@latest
|
||||
go install github.com/wailsapp/wails/v2/cmd/wails@v2.8.0
|
||||
rm ./backend-python/rwkv_pip/wkv_cuda.pyd
|
||||
rm ./backend-python/rwkv_pip/rwkv5.pyd
|
||||
rm ./backend-python/rwkv_pip/rwkv6.pyd
|
||||
rm ./backend-python/rwkv_pip/beta/wkv_cuda.pyd
|
||||
rm ./backend-python/get-pip.py
|
||||
rm ./backend-python/rwkv_pip/cpp/librwkv.dylib
|
||||
rm ./backend-python/rwkv_pip/cpp/rwkv.dll
|
||||
@ -108,20 +107,19 @@ jobs:
|
||||
runs-on: macos-13
|
||||
needs: create-draft
|
||||
steps:
|
||||
- uses: actions/checkout@v3
|
||||
- uses: actions/checkout@v4
|
||||
with:
|
||||
ref: master
|
||||
- uses: actions/setup-go@v4
|
||||
- uses: actions/setup-go@v5
|
||||
with:
|
||||
go-version: '1.20.5'
|
||||
go-version: "1.20.5"
|
||||
- run: |
|
||||
wget https://github.com/josStorer/ai00_rwkv_server/releases/latest/download/webgpu_server_darwin_aarch64 -O ./backend-rust/webgpu_server
|
||||
wget https://github.com/josStorer/web-rwkv-converter/releases/latest/download/web-rwkv-converter_darwin_aarch64 -O ./backend-rust/web-rwkv-converter
|
||||
go install github.com/wailsapp/wails/v2/cmd/wails@latest
|
||||
go install github.com/wailsapp/wails/v2/cmd/wails@v2.8.0
|
||||
rm ./backend-python/rwkv_pip/wkv_cuda.pyd
|
||||
rm ./backend-python/rwkv_pip/rwkv5.pyd
|
||||
rm ./backend-python/rwkv_pip/rwkv6.pyd
|
||||
rm ./backend-python/rwkv_pip/beta/wkv_cuda.pyd
|
||||
rm ./backend-python/get-pip.py
|
||||
rm ./backend-python/rwkv_pip/cpp/rwkv.dll
|
||||
rm ./backend-python/rwkv_pip/cpp/librwkv.so
|
||||
@ -137,5 +135,5 @@ jobs:
|
||||
runs-on: ubuntu-22.04
|
||||
needs: [ windows, linux, macos ]
|
||||
steps:
|
||||
- uses: actions/checkout@v3
|
||||
- uses: actions/checkout@v4
|
||||
- run: gh release edit ${{github.ref_name}} --draft=false
|
||||
|
1
.gitignore
vendored
1
.gitignore
vendored
@ -19,7 +19,6 @@ __pycache__
|
||||
/cmd-helper.bat
|
||||
/install-py-dep.bat
|
||||
/backend-python/wkv_cuda
|
||||
/backend-python/rwkv*
|
||||
*.exe
|
||||
*.old
|
||||
.DS_Store
|
||||
|
@ -1,10 +1,31 @@
|
||||
## Changes
|
||||
## v1.8.4
|
||||
|
||||
- WebGPU (Python) strategy
|
||||
- fix f05a4a, __init__.py is not embedded
|
||||
|
||||
## v1.8.3
|
||||
|
||||
### Deprecations
|
||||
|
||||
- rwkv-beta is deprecated
|
||||
|
||||
### Upgrades
|
||||
|
||||
- bump webgpu(python) (https://github.com/cryscan/web-rwkv-py)
|
||||
- sync https://github.com/JL-er/RWKV-PEFT (LoRA)
|
||||
|
||||
### Improvements
|
||||
|
||||
- improve default LoRA fine-tune params
|
||||
|
||||
### Fixes
|
||||
|
||||
- fix #342, #345: cannot import name 'packaging' from 'pkg_resources'
|
||||
- fix the huge error prompt that pops up when running in webgpu mode
|
||||
|
||||
## Install
|
||||
|
||||
- Windows: https://github.com/josStorer/RWKV-Runner/blob/master/build/windows/Readme_Install.txt
|
||||
- MacOS: https://github.com/josStorer/RWKV-Runner/blob/master/build/darwin/Readme_Install.txt
|
||||
- Linux: https://github.com/josStorer/RWKV-Runner/blob/master/build/linux/Readme_Install.txt
|
||||
- Server-Deploy-Examples: https://github.com/josStorer/RWKV-Runner/tree/master/deploy-examples
|
||||
- Simple Deploy Example: https://github.com/josStorer/RWKV-Runner/blob/master/README.md#simple-deploy-example
|
||||
- Server Deploy Examples: https://github.com/josStorer/RWKV-Runner/tree/master/deploy-examples
|
||||
|
55
Dockerfile
Normal file
55
Dockerfile
Normal file
@ -0,0 +1,55 @@
|
||||
FROM node:21-slim AS frontend
|
||||
|
||||
RUN echo "registry=https://registry.npmmirror.com/" > ~/.npmrc
|
||||
|
||||
WORKDIR /app
|
||||
|
||||
COPY manifest.json manifest.json
|
||||
COPY frontend frontend
|
||||
|
||||
WORKDIR /app/frontend
|
||||
|
||||
RUN npm ci
|
||||
RUN npm run build
|
||||
|
||||
FROM nvidia/cuda:11.6.1-devel-ubuntu20.04 AS runtime
|
||||
|
||||
ENV DEBIAN_FRONTEND=noninteractive
|
||||
|
||||
RUN apt update && \
|
||||
apt install -yq git curl wget build-essential ninja-build aria2 jq software-properties-common
|
||||
|
||||
RUN add-apt-repository -y ppa:deadsnakes/ppa && \
|
||||
add-apt-repository -y ppa:ubuntu-toolchain-r/test && \
|
||||
apt install -y g++-11 python3.10 python3.10-distutils python3.10-dev && \
|
||||
curl -sS http://mirrors.aliyun.com/pypi/get-pip.py | python3.10
|
||||
|
||||
RUN python3.10 -m pip install cmake
|
||||
|
||||
FROM runtime AS librwkv
|
||||
|
||||
WORKDIR /app
|
||||
|
||||
RUN git clone https://github.com/RWKV/rwkv.cpp.git && \
|
||||
cd rwkv.cpp && \
|
||||
git submodule update --init --recursive && \
|
||||
mkdir -p build && \
|
||||
cd build && \
|
||||
cmake -G Ninja .. && \
|
||||
cmake --build .
|
||||
|
||||
FROM runtime AS final
|
||||
|
||||
WORKDIR /app
|
||||
|
||||
COPY ./backend-python/requirements.txt ./backend-python/requirements.txt
|
||||
|
||||
RUN python3.10 -m pip install --quiet -r ./backend-python/requirements.txt
|
||||
|
||||
COPY . .
|
||||
COPY --from=frontend /app/frontend/dist /app/frontend/dist
|
||||
COPY --from=librwkv /app/rwkv.cpp/build/librwkv.so /app/backend-python/rwkv_pip/cpp/librwkv.so
|
||||
|
||||
EXPOSE 27777
|
||||
|
||||
CMD ["python3.10", "./backend-python/main.py", "--port", "27777", "--host", "0.0.0.0", "--webui"]
|
6
Makefile
6
Makefile
@ -8,7 +8,8 @@ endif
|
||||
|
||||
build-windows:
|
||||
@echo ---- build for windows
|
||||
wails build -upx -ldflags '-s -w -extldflags "-static"' -platform windows/amd64
|
||||
wails build -ldflags '-s -w -extldflags "-static"' -platform windows/amd64
|
||||
upx -9 --lzma ./build/bin/RWKV-Runner.exe
|
||||
|
||||
build-macos:
|
||||
@echo ---- build for macos
|
||||
@ -16,7 +17,8 @@ build-macos:
|
||||
|
||||
build-linux:
|
||||
@echo ---- build for linux
|
||||
wails build -upx -ldflags '-s -w' -platform linux/amd64
|
||||
wails build -ldflags '-s -w' -platform linux/amd64
|
||||
upx -9 --lzma ./build/bin/RWKV-Runner
|
||||
|
||||
build-web:
|
||||
@echo ---- build for web
|
||||
|
47
README.md
47
README.md
@ -1,5 +1,5 @@
|
||||
<p align="center">
|
||||
<img src="https://github.com/josStorer/RWKV-Runner/assets/13366013/d24834b0-265d-45f5-93c0-fac1e19562af">
|
||||
<img src="https://github.com/josStorer/RWKV-Runner/assets/13366013/65c46133-7506-4b54-b64f-fe49f188afa7">
|
||||
</p>
|
||||
|
||||
<h1 align="center">RWKV Runner</h1>
|
||||
@ -12,6 +12,7 @@ compatible with the OpenAI API, which means that every ChatGPT client is an RWKV
|
||||
|
||||
[![license][license-image]][license-url]
|
||||
[![release][release-image]][release-url]
|
||||
[![py-version][py-version-image]][py-version-url]
|
||||
|
||||
English | [简体中文](README_ZH.md) | [日本語](README_JA.md)
|
||||
|
||||
@ -31,6 +32,10 @@ English | [简体中文](README_ZH.md) | [日本語](README_JA.md)
|
||||
|
||||
[release-url]: https://github.com/josStorer/RWKV-Runner/releases/latest
|
||||
|
||||
[py-version-image]: https://img.shields.io/pypi/pyversions/fastapi.svg
|
||||
|
||||
[py-version-url]: https://github.com/josStorer/RWKV-Runner/tree/master/backend-python
|
||||
|
||||
[download-url]: https://github.com/josStorer/RWKV-Runner/releases
|
||||
|
||||
[Windows-image]: https://img.shields.io/badge/-Windows-blue?logo=windows
|
||||
@ -47,13 +52,28 @@ English | [简体中文](README_ZH.md) | [日本語](README_JA.md)
|
||||
|
||||
</div>
|
||||
|
||||
#### Tip: You can deploy [backend-python](./backend-python/) on a server and use this program as a client only. Fill in your server address in the Settings `API URL`.
|
||||
## Tips
|
||||
|
||||
#### Default configs has enabled custom CUDA kernel acceleration, which is much faster and consumes much less VRAM. If you encounter possible compatibility issues (output garbled), go to the Configs page and turn off `Use Custom CUDA kernel to Accelerate`, or try to upgrade your gpu driver.
|
||||
- You can deploy [backend-python](./backend-python/) on a server and use this program as a client only. Fill in
|
||||
your server address in the Settings `API URL`.
|
||||
|
||||
#### If Windows Defender claims this is a virus, you can try downloading [v1.3.7_win.zip](https://github.com/josStorer/RWKV-Runner/releases/download/v1.3.7/RWKV-Runner_win.zip) and letting it update automatically to the latest version, or add it to the trusted list (`Windows Security` -> `Virus & threat protection` -> `Manage settings` -> `Exclusions` -> `Add or remove exclusions` -> `Add an exclusion` -> `Folder` -> `RWKV-Runner`).
|
||||
- If you are deploying and providing public services, please limit the request size through API gateway to prevent
|
||||
excessive resource usage caused by submitting overly long prompts. Additionally, please restrict the upper limit of
|
||||
requests' max_tokens based on your actual
|
||||
situation: https://github.com/josStorer/RWKV-Runner/blob/master/backend-python/utils/rwkv.py#L567, the default is set
|
||||
as le=102400, which may result in significant resource consumption for individual responses in extreme cases.
|
||||
|
||||
#### For different tasks, adjusting API parameters can achieve better results. For example, for translation tasks, you can try setting Temperature to 1 and Top_P to 0.3.
|
||||
- Default configs has enabled custom CUDA kernel acceleration, which is much faster and consumes much less VRAM. If you
|
||||
encounter possible compatibility issues (output garbled), go to the Configs page and turn
|
||||
off `Use Custom CUDA kernel to Accelerate`, or try to upgrade your gpu driver.
|
||||
|
||||
- If Windows Defender claims this is a virus, you can try
|
||||
downloading [v1.3.7_win.zip](https://github.com/josStorer/RWKV-Runner/releases/download/v1.3.7/RWKV-Runner_win.zip)
|
||||
and letting it update automatically to the latest version, or add it to the trusted
|
||||
list (`Windows Security` -> `Virus & threat protection` -> `Manage settings` -> `Exclusions` -> `Add or remove exclusions` -> `Add an exclusion` -> `Folder` -> `RWKV-Runner`).
|
||||
|
||||
- For different tasks, adjusting API parameters can achieve better results. For example, for translation tasks, you can
|
||||
try setting Temperature to 1 and Top_P to 0.3.
|
||||
|
||||
## Features
|
||||
|
||||
@ -74,7 +94,8 @@ English | [简体中文](README_ZH.md) | [日本語](README_JA.md)
|
||||
- Built-in model conversion tool.
|
||||
- Built-in download management and remote model inspection.
|
||||
- Built-in one-click LoRA Finetune. (Windows Only)
|
||||
- Can also be used as an OpenAI ChatGPT and GPT-Playground client. (Fill in the API URL and API Key in Settings page)
|
||||
- Can also be used as an OpenAI ChatGPT, GPT-Playground, Ollama and more clients. (Fill in the API URL and API Key in
|
||||
Settings page)
|
||||
- Multilingual localization.
|
||||
- Theme switching.
|
||||
- Automatic updates.
|
||||
@ -168,6 +189,10 @@ Tip: You can download https://github.com/josStorer/sgm_plus and unzip it to the
|
||||
to use it as an offline sound source. Please note that if you are compiling the program from source code, do not place
|
||||
it in the source code directory.
|
||||
|
||||
If you don't have a MIDI keyboard, you can use virtual MIDI input software like `Virtual Midi Controller 3 LE`, along
|
||||
with [loopMIDI](https://www.tobias-erichsen.de/wp-content/uploads/2020/01/loopMIDISetup_1_0_16_27.zip), to use a regular
|
||||
computer keyboard as MIDI input.
|
||||
|
||||
### USB MIDI Connection
|
||||
|
||||
- USB MIDI devices are plug-and-play, and you can select your input device in the Composition page
|
||||
@ -206,24 +231,30 @@ it in the source code directory.
|
||||
|
||||
## Related Repositories:
|
||||
|
||||
- RWKV-5-World: https://huggingface.co/BlinkDL/rwkv-5-world/tree/main
|
||||
- RWKV-4-World: https://huggingface.co/BlinkDL/rwkv-4-world/tree/main
|
||||
- RWKV-4-Raven: https://huggingface.co/BlinkDL/rwkv-4-raven/tree/main
|
||||
- ChatRWKV: https://github.com/BlinkDL/ChatRWKV
|
||||
- RWKV-LM: https://github.com/BlinkDL/RWKV-LM
|
||||
- RWKV-LM-LoRA: https://github.com/Blealtan/RWKV-LM-LoRA
|
||||
- RWKV-v5-lora: https://github.com/JL-er/RWKV-v5-lora
|
||||
- MIDI-LLM-tokenizer: https://github.com/briansemrau/MIDI-LLM-tokenizer
|
||||
- ai00_rwkv_server: https://github.com/cgisky1980/ai00_rwkv_server
|
||||
- rwkv.cpp: https://github.com/saharNooby/rwkv.cpp
|
||||
- web-rwkv-py: https://github.com/cryscan/web-rwkv-py
|
||||
- web-rwkv: https://github.com/cryscan/web-rwkv
|
||||
|
||||
## Preview
|
||||
|
||||
### Homepage
|
||||
|
||||
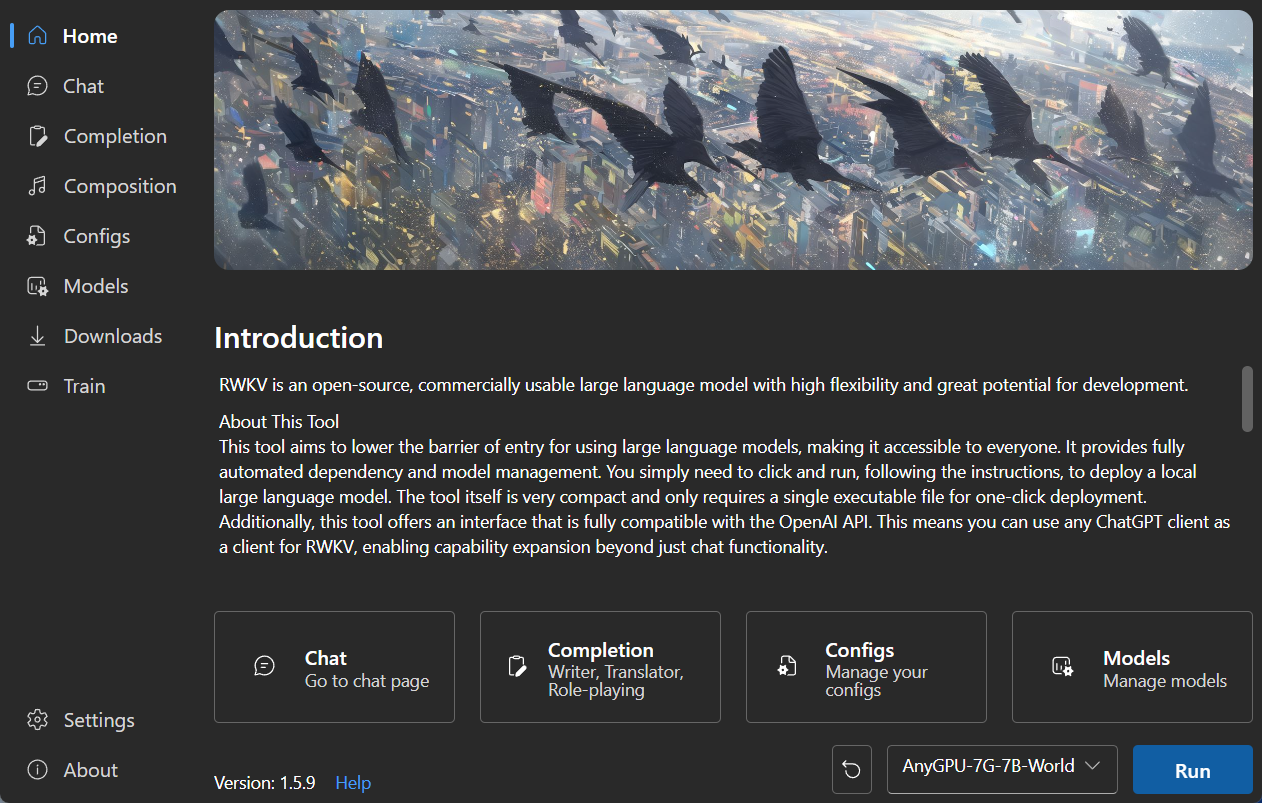
|
||||

|
||||
|
||||
### Chat
|
||||
|
||||
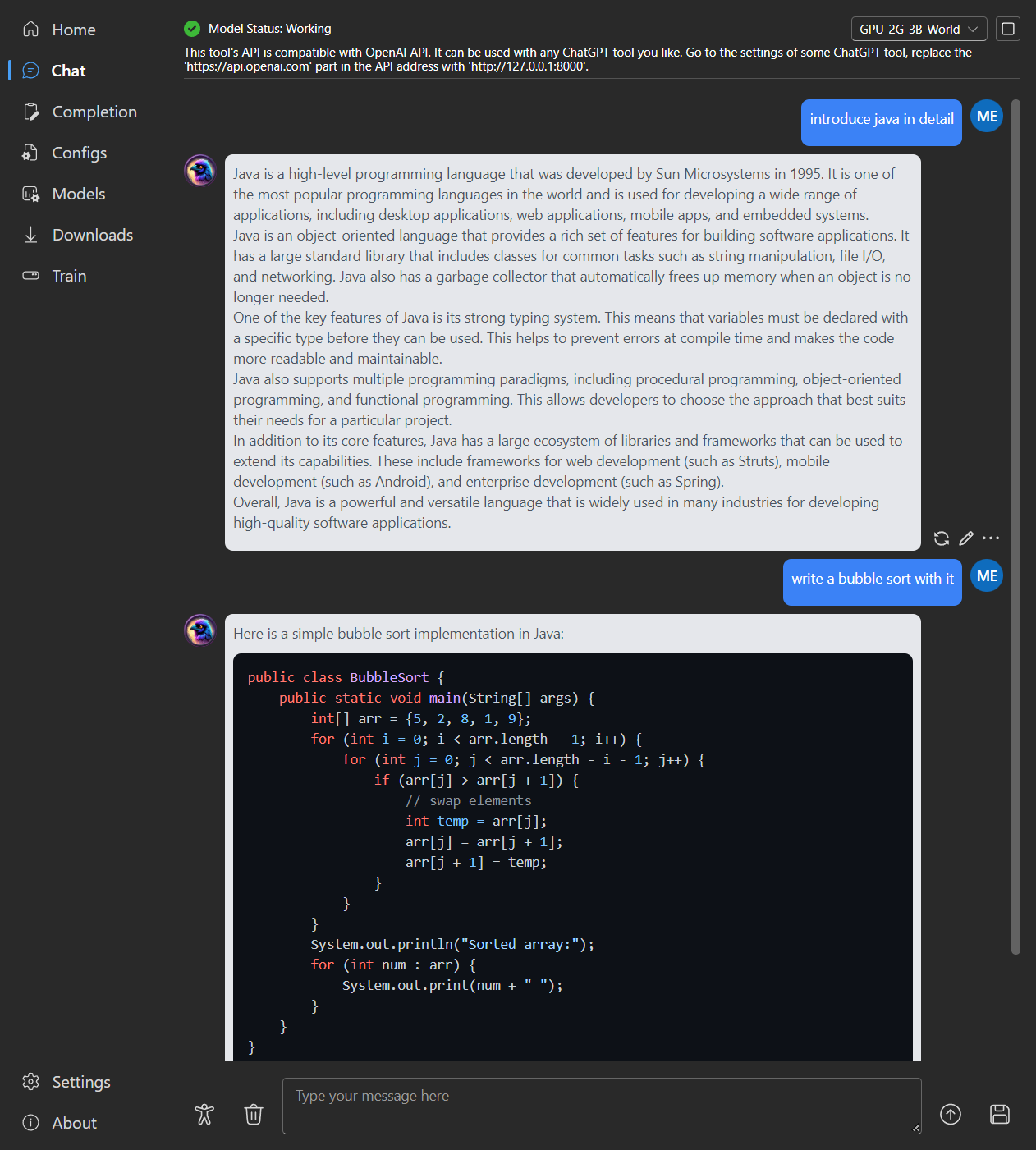
|
||||
|
||||
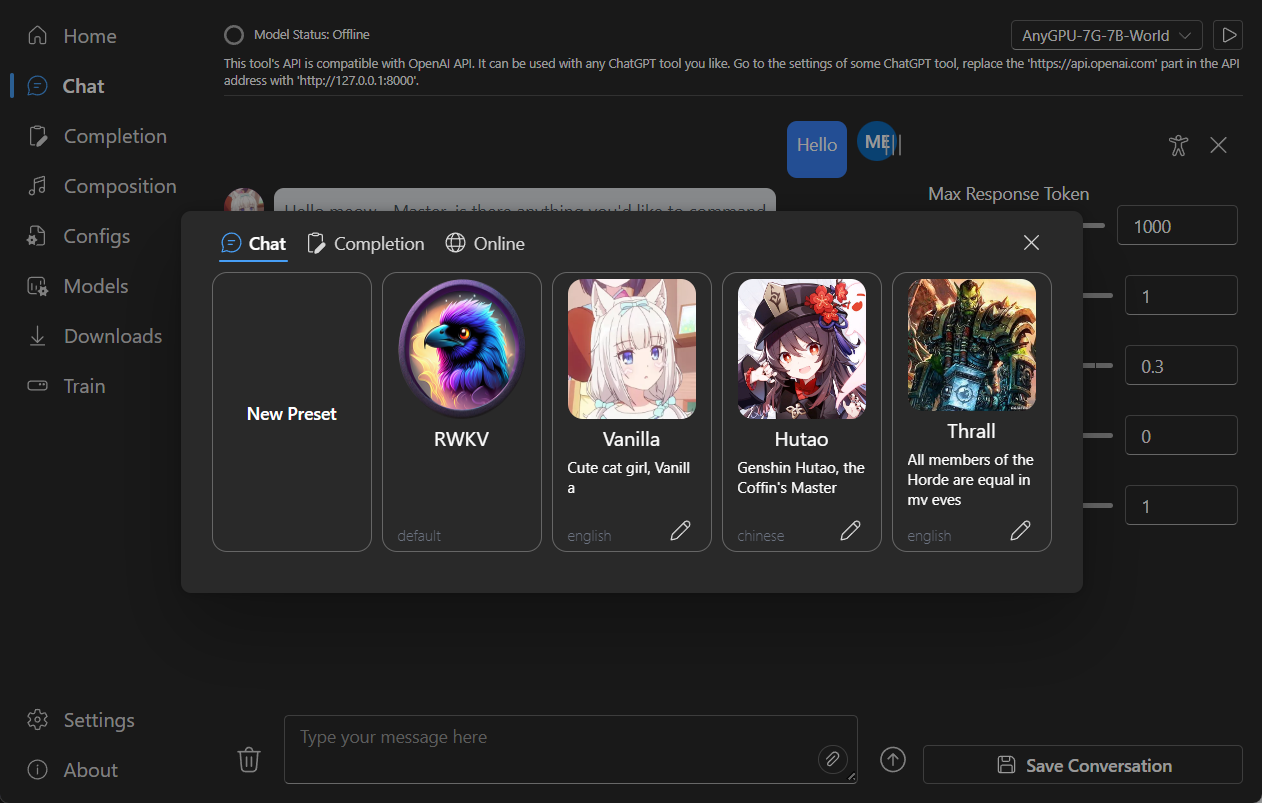
|
||||

|
||||
|
||||
### Completion
|
||||
|
||||
|
46
README_JA.md
46
README_JA.md
@ -1,5 +1,5 @@
|
||||
<p align="center">
|
||||
<img src="https://github.com/josStorer/RWKV-Runner/assets/13366013/d24834b0-265d-45f5-93c0-fac1e19562af">
|
||||
<img src="https://github.com/josStorer/RWKV-Runner/assets/13366013/65c46133-7506-4b54-b64f-fe49f188afa7">
|
||||
</p>
|
||||
|
||||
<h1 align="center">RWKV Runner</h1>
|
||||
@ -12,6 +12,7 @@
|
||||
|
||||
[![license][license-image]][license-url]
|
||||
[![release][release-image]][release-url]
|
||||
[![py-version][py-version-image]][py-version-url]
|
||||
|
||||
[English](README.md) | [简体中文](README_ZH.md) | 日本語
|
||||
|
||||
@ -31,6 +32,10 @@
|
||||
|
||||
[release-url]: https://github.com/josStorer/RWKV-Runner/releases/latest
|
||||
|
||||
[py-version-image]: https://img.shields.io/pypi/pyversions/fastapi.svg
|
||||
|
||||
[py-version-url]: https://github.com/josStorer/RWKV-Runner/tree/master/backend-python
|
||||
|
||||
[download-url]: https://github.com/josStorer/RWKV-Runner/releases
|
||||
|
||||
[Windows-image]: https://img.shields.io/badge/-Windows-blue?logo=windows
|
||||
@ -47,13 +52,26 @@
|
||||
|
||||
</div>
|
||||
|
||||
#### ヒント:サーバーに[backend-python](./backend-python/)をデプロイし、このプログラムをクライアントとして使用することができます。設定された`API URL`にサーバーアドレスを入力してください。
|
||||
## ヒント
|
||||
|
||||
#### デフォルトの設定はカスタム CUDA カーネルアクセラレーションを有効にしています。互換性の問題 (文字化けを出力する) が発生する可能性がある場合は、コンフィグページに移動し、`Use Custom CUDA kernel to Accelerate` をオフにしてください、あるいは、GPUドライバーをアップグレードしてみてください。
|
||||
- サーバーに [backend-python](./backend-python/)
|
||||
をデプロイし、このプログラムをクライアントとして使用することができます。設定された`API URL`にサーバーアドレスを入力してください。
|
||||
|
||||
#### Windows Defender がこれをウイルスだと主張する場合は、[v1.3.7_win.zip](https://github.com/josStorer/RWKV-Runner/releases/download/v1.3.7/RWKV-Runner_win.zip) をダウンロードして最新版に自動更新させるか、信頼済みリストに追加してみてください (`Windows Security` -> `Virus & threat protection` -> `Manage settings` -> `Exclusions` -> `Add or remove exclusions` -> `Add an exclusion` -> `Folder` -> `RWKV-Runner`)。
|
||||
- もし、あなたがデプロイし、外部に公開するサービスを提供している場合、APIゲートウェイを使用してリクエストのサイズを制限し、
|
||||
長すぎるプロンプトの提出がリソースを占有しないようにしてください。さらに、実際の状況に応じて、リクエストの max_tokens
|
||||
の上限を制限してください:https://github.com/josStorer/RWKV-Runner/blob/master/backend-python/utils/rwkv.py#L567
|
||||
、デフォルトは le=102400 ですが、極端な場合には単一の応答が大量のリソースを消費する可能性があります。
|
||||
|
||||
#### 異なるタスクについては、API パラメータを調整することで、より良い結果を得ることができます。例えば、翻訳タスクの場合、Temperature を 1 に、Top_P を 0.3 に設定してみてください。
|
||||
- デフォルトの設定はカスタム CUDA カーネルアクセラレーションを有効にしています。互換性の問題 (文字化けを出力する)
|
||||
が発生する可能性がある場合は、コンフィグページに移動し、`Use Custom CUDA kernel to Accelerate`
|
||||
をオフにしてください、あるいは、GPUドライバーをアップグレードしてみてください。
|
||||
|
||||
- Windows Defender
|
||||
がこれをウイルスだと主張する場合は、[v1.3.7_win.zip](https://github.com/josStorer/RWKV-Runner/releases/download/v1.3.7/RWKV-Runner_win.zip)
|
||||
をダウンロードして最新版に自動更新させるか、信頼済みリストに追加してみてください (`Windows Security` -> `Virus & threat protection` -> `Manage settings` -> `Exclusions` -> `Add or remove exclusions` -> `Add an exclusion` -> `Folder` -> `RWKV-Runner`)。
|
||||
|
||||
- 異なるタスクについては、API パラメータを調整することで、より良い結果を得ることができます。例えば、翻訳タスクの場合、Temperature
|
||||
を 1 に、Top_P を 0.3 に設定してみてください。
|
||||
|
||||
## 特徴
|
||||
|
||||
@ -71,8 +89,8 @@
|
||||
- 内蔵モデル変換ツール
|
||||
- ダウンロード管理とリモートモデル検査機能内蔵
|
||||
- 内蔵のLoRA微調整機能を搭載しています (Windowsのみ)
|
||||
- このプログラムは、OpenAI ChatGPTとGPT Playgroundのクライアントとしても使用できます(設定ページで `API URL` と `API Key`
|
||||
を入力してください)
|
||||
- このプログラムは、OpenAI ChatGPT、GPT Playground、Ollama などのクライアントとしても使用できます(設定ページで `API URL`
|
||||
と `API Key` を入力してください)
|
||||
- 多言語ローカライズ
|
||||
- テーマ切り替え
|
||||
- 自動アップデート
|
||||
@ -167,6 +185,10 @@ Tip: You can download https://github.com/josStorer/sgm_plus and unzip it to the
|
||||
to use it as an offline sound source. Please note that if you are compiling the program from source code, do not place
|
||||
it in the source code directory.
|
||||
|
||||
MIDIキーボードをお持ちでない場合、`Virtual Midi Controller 3 LE`
|
||||
などの仮想MIDI入力ソフトウェアを使用することができます。[loopMIDI](https://www.tobias-erichsen.de/wp-content/uploads/2020/01/loopMIDISetup_1_0_16_27.zip)
|
||||
を組み合わせて、通常のコンピュータキーボードをMIDI入力として使用できます。
|
||||
|
||||
### USB MIDI Connection
|
||||
|
||||
- USB MIDI devices are plug-and-play, and you can select your input device in the Composition page
|
||||
@ -205,24 +227,30 @@ it in the source code directory.
|
||||
|
||||
## 関連リポジトリ:
|
||||
|
||||
- RWKV-5-World: https://huggingface.co/BlinkDL/rwkv-5-world/tree/main
|
||||
- RWKV-4-World: https://huggingface.co/BlinkDL/rwkv-4-world/tree/main
|
||||
- RWKV-4-Raven: https://huggingface.co/BlinkDL/rwkv-4-raven/tree/main
|
||||
- ChatRWKV: https://github.com/BlinkDL/ChatRWKV
|
||||
- RWKV-LM: https://github.com/BlinkDL/RWKV-LM
|
||||
- RWKV-LM-LoRA: https://github.com/Blealtan/RWKV-LM-LoRA
|
||||
- RWKV-v5-lora: https://github.com/JL-er/RWKV-v5-lora
|
||||
- MIDI-LLM-tokenizer: https://github.com/briansemrau/MIDI-LLM-tokenizer
|
||||
- ai00_rwkv_server: https://github.com/cgisky1980/ai00_rwkv_server
|
||||
- rwkv.cpp: https://github.com/saharNooby/rwkv.cpp
|
||||
- web-rwkv-py: https://github.com/cryscan/web-rwkv-py
|
||||
- web-rwkv: https://github.com/cryscan/web-rwkv
|
||||
|
||||
## Preview
|
||||
|
||||
### ホームページ
|
||||
|
||||
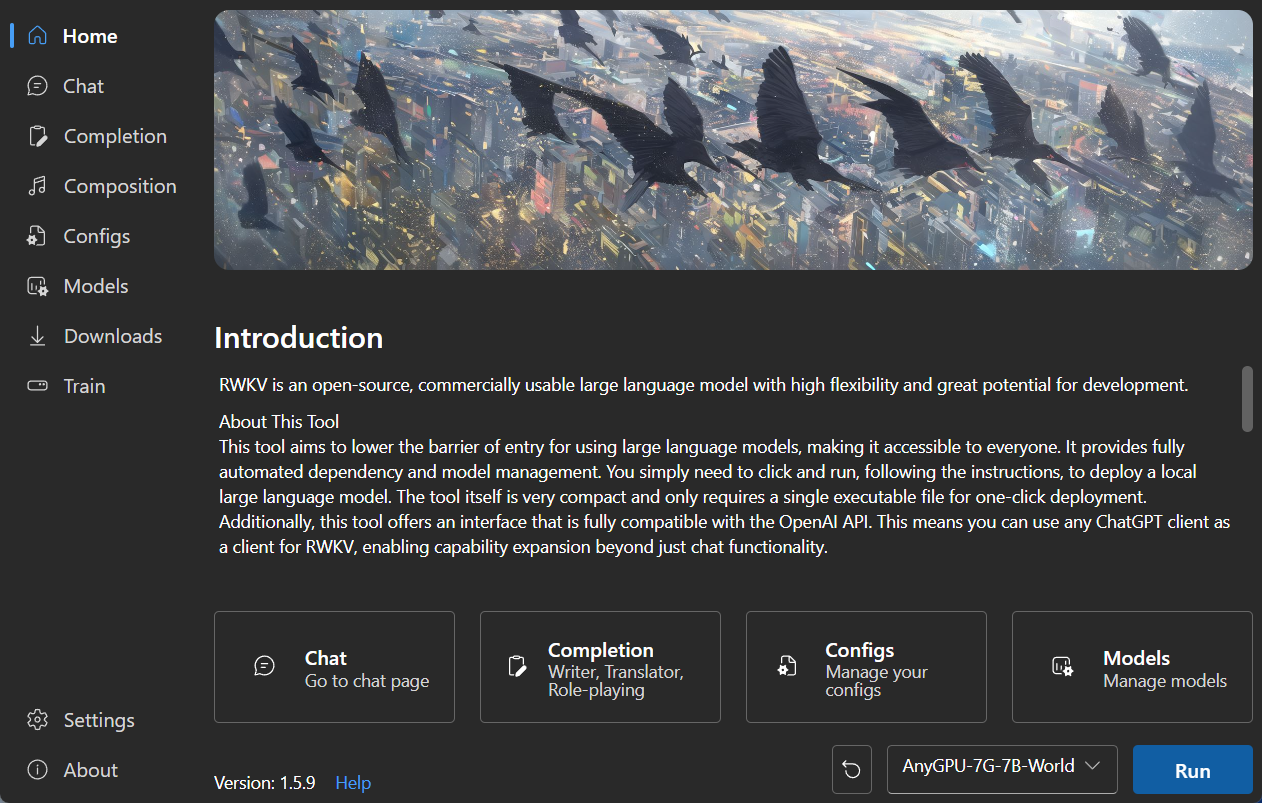
|
||||

|
||||
|
||||
### チャット
|
||||
|
||||
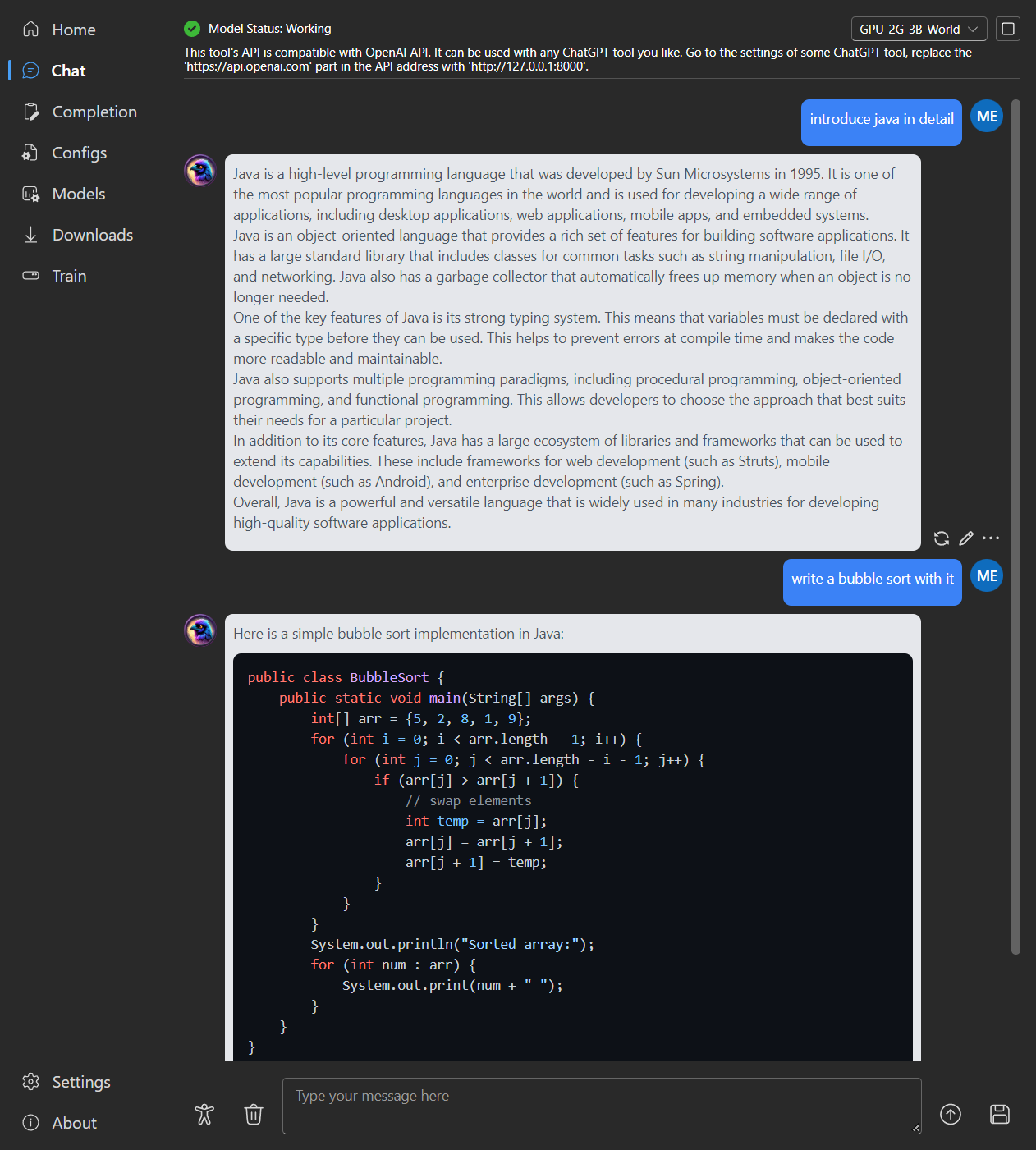
|
||||
|
||||
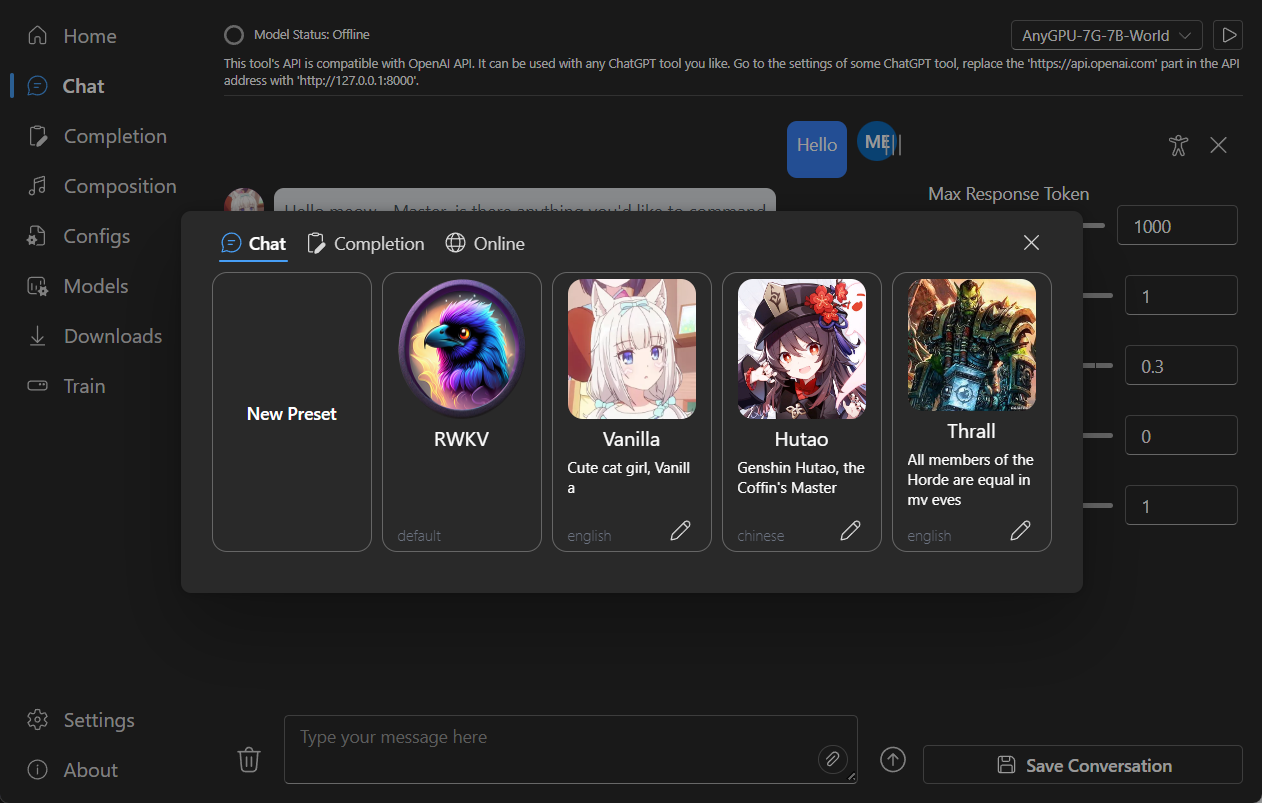
|
||||

|
||||
|
||||
### 補完
|
||||
|
||||
|
39
README_ZH.md
39
README_ZH.md
@ -1,5 +1,5 @@
|
||||
<p align="center">
|
||||
<img src="https://github.com/josStorer/RWKV-Runner/assets/13366013/d24834b0-265d-45f5-93c0-fac1e19562af">
|
||||
<img src="https://github.com/josStorer/RWKV-Runner/assets/13366013/65c46133-7506-4b54-b64f-fe49f188afa7">
|
||||
</p>
|
||||
|
||||
<h1 align="center">RWKV Runner</h1>
|
||||
@ -11,6 +11,7 @@ API兼容的接口,这意味着一切ChatGPT客户端都是RWKV客户端。
|
||||
|
||||
[![license][license-image]][license-url]
|
||||
[![release][release-image]][release-url]
|
||||
[![py-version][py-version-image]][py-version-url]
|
||||
|
||||
[English](README.md) | 简体中文 | [日本語](README_JA.md)
|
||||
|
||||
@ -30,6 +31,10 @@ API兼容的接口,这意味着一切ChatGPT客户端都是RWKV客户端。
|
||||
|
||||
[release-url]: https://github.com/josStorer/RWKV-Runner/releases/latest
|
||||
|
||||
[py-version-image]: https://img.shields.io/pypi/pyversions/fastapi.svg
|
||||
|
||||
[py-version-url]: https://github.com/josStorer/RWKV-Runner/tree/master/backend-python
|
||||
|
||||
[download-url]: https://github.com/josStorer/RWKV-Runner/releases
|
||||
|
||||
[Windows-image]: https://img.shields.io/badge/-Windows-blue?logo=windows
|
||||
@ -46,13 +51,22 @@ API兼容的接口,这意味着一切ChatGPT客户端都是RWKV客户端。
|
||||
|
||||
</div>
|
||||
|
||||
#### 小贴士:你可以在服务器部署[backend-python](./backend-python/),然后将此程序仅用作客户端,在设置的`API URL`中填入你的服务器地址
|
||||
## 小贴士
|
||||
|
||||
#### 预设配置已经开启自定义CUDA算子加速,速度更快,且显存消耗更少。如果你遇到可能的兼容性(输出乱码)问题,前往配置页面,关闭`使用自定义CUDA算子加速`,或更新你的显卡驱动
|
||||
- 你可以在服务器部署[backend-python](./backend-python/),然后将此程序仅用作客户端,在设置的`API URL`中填入你的服务器地址
|
||||
|
||||
#### 如果Windows Defender说这是一个病毒,你可以尝试下载[v1.3.7_win.zip](https://github.com/josStorer/RWKV-Runner/releases/download/v1.3.7/RWKV-Runner_win.zip),然后让其自动更新到最新版,或添加信任 (`Windows Security` -> `Virus & threat protection` -> `Manage settings` -> `Exclusions` -> `Add or remove exclusions` -> `Add an exclusion` -> `Folder` -> `RWKV-Runner`)
|
||||
- 如果你正在部署并对外提供公开服务,请通过API网关限制请求大小,避免过长的prompt提交占用资源。此外,请根据你的实际情况,限制请求的
|
||||
max_tokens 上限: https://github.com/josStorer/RWKV-Runner/blob/master/backend-python/utils/rwkv.py#L567,
|
||||
默认le=102400, 这可能导致极端情况下单个响应消耗大量资源
|
||||
|
||||
#### 对于不同的任务,调整API参数会获得更好的效果,例如对于翻译任务,你可以尝试设置Temperature为1,Top_P为0.3
|
||||
- 预设配置已经开启自定义CUDA算子加速,速度更快,且显存消耗更少。如果你遇到可能的兼容性(输出乱码)
|
||||
问题,前往配置页面,关闭`使用自定义CUDA算子加速`,或更新你的显卡驱动
|
||||
|
||||
- 如果 Windows Defender
|
||||
说这是一个病毒,你可以尝试下载[v1.3.7_win.zip](https://github.com/josStorer/RWKV-Runner/releases/download/v1.3.7/RWKV-Runner_win.zip),
|
||||
然后让其自动更新到最新版,或添加信任 (`Windows Security` -> `Virus & threat protection` -> `Manage settings` -> `Exclusions` -> `Add or remove exclusions` -> `Add an exclusion` -> `Folder` -> `RWKV-Runner`)
|
||||
|
||||
- 对于不同的任务,调整API参数会获得更好的效果,例如对于翻译任务,你可以尝试设置Temperature为1,Top_P为0.3
|
||||
|
||||
## 功能
|
||||
|
||||
@ -69,7 +83,7 @@ API兼容的接口,这意味着一切ChatGPT客户端都是RWKV客户端。
|
||||
- 内置模型转换工具
|
||||
- 内置下载管理和远程模型检视
|
||||
- 内置一键LoRA微调 (仅限Windows)
|
||||
- 也可用作 OpenAI ChatGPT 和 GPT Playground 客户端 (在设置内填写API URL和API Key)
|
||||
- 也可用作 OpenAI ChatGPT, GPT Playground, Ollama 等服务的客户端 (在设置内填写API URL和API Key)
|
||||
- 多语言本地化
|
||||
- 主题切换
|
||||
- 自动更新
|
||||
@ -161,6 +175,9 @@ for i in np.argsort(embeddings_cos_sim)[::-1]:
|
||||
小贴士: 你可以下载 https://github.com/josStorer/sgm_plus, 并解压到程序的`assets/sound-font`目录, 以使用离线音源. 注意,
|
||||
如果你正在从源码编译程序, 请不要将其放置在源码目录中
|
||||
|
||||
如果你没有MIDI键盘, 你可以使用像 `Virtual Midi Controller 3 LE` 这样的虚拟MIDI输入软件,
|
||||
配合[loopMIDI](https://www.tobias-erichsen.de/wp-content/uploads/2020/01/loopMIDISetup_1_0_16_27.zip), 使用普通电脑键盘作为MIDI输入
|
||||
|
||||
### USB MIDI 连接
|
||||
|
||||
- USB MIDI设备是即插即用的, 你能够在作曲页面选择你的输入设备
|
||||
@ -192,24 +209,30 @@ for i in np.argsort(embeddings_cos_sim)[::-1]:
|
||||
|
||||
## 相关仓库:
|
||||
|
||||
- RWKV-5-World: https://huggingface.co/BlinkDL/rwkv-5-world/tree/main
|
||||
- RWKV-4-World: https://huggingface.co/BlinkDL/rwkv-4-world/tree/main
|
||||
- RWKV-4-Raven: https://huggingface.co/BlinkDL/rwkv-4-raven/tree/main
|
||||
- ChatRWKV: https://github.com/BlinkDL/ChatRWKV
|
||||
- RWKV-LM: https://github.com/BlinkDL/RWKV-LM
|
||||
- RWKV-LM-LoRA: https://github.com/Blealtan/RWKV-LM-LoRA
|
||||
- RWKV-v5-lora: https://github.com/JL-er/RWKV-v5-lora
|
||||
- MIDI-LLM-tokenizer: https://github.com/briansemrau/MIDI-LLM-tokenizer
|
||||
- ai00_rwkv_server: https://github.com/cgisky1980/ai00_rwkv_server
|
||||
- rwkv.cpp: https://github.com/saharNooby/rwkv.cpp
|
||||
- web-rwkv-py: https://github.com/cryscan/web-rwkv-py
|
||||
- web-rwkv: https://github.com/cryscan/web-rwkv
|
||||
|
||||
## Preview
|
||||
|
||||
### 主页
|
||||
|
||||
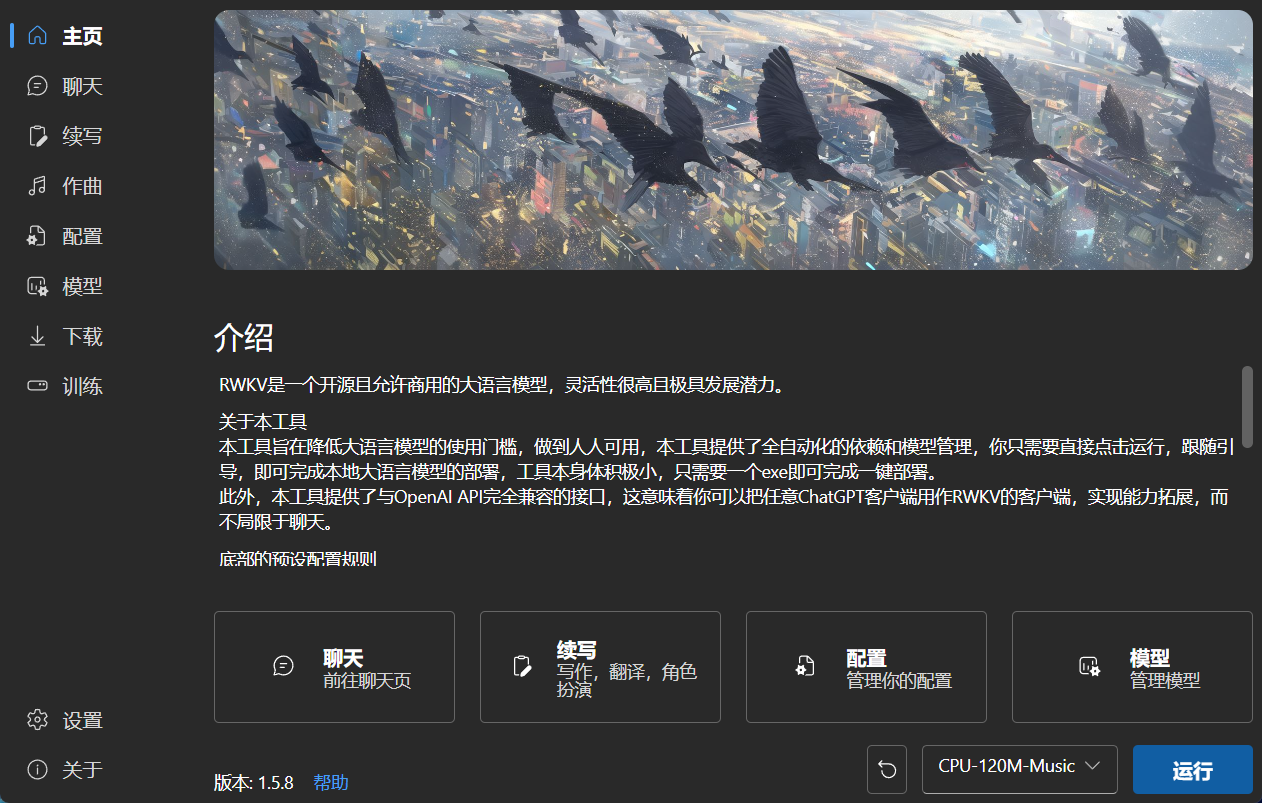
|
||||

|
||||
|
||||
### 聊天
|
||||
|
||||
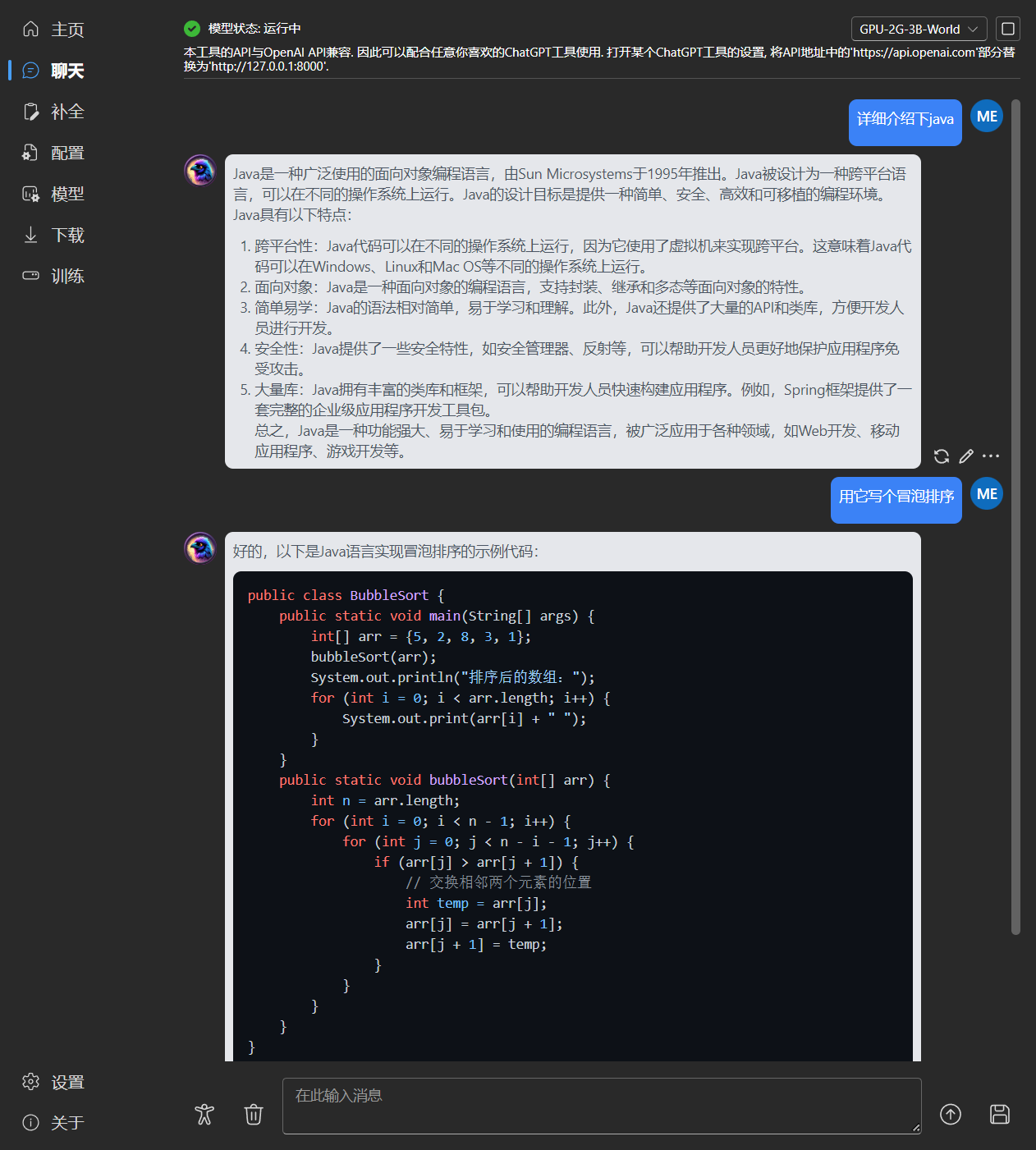
|
||||
|
||||
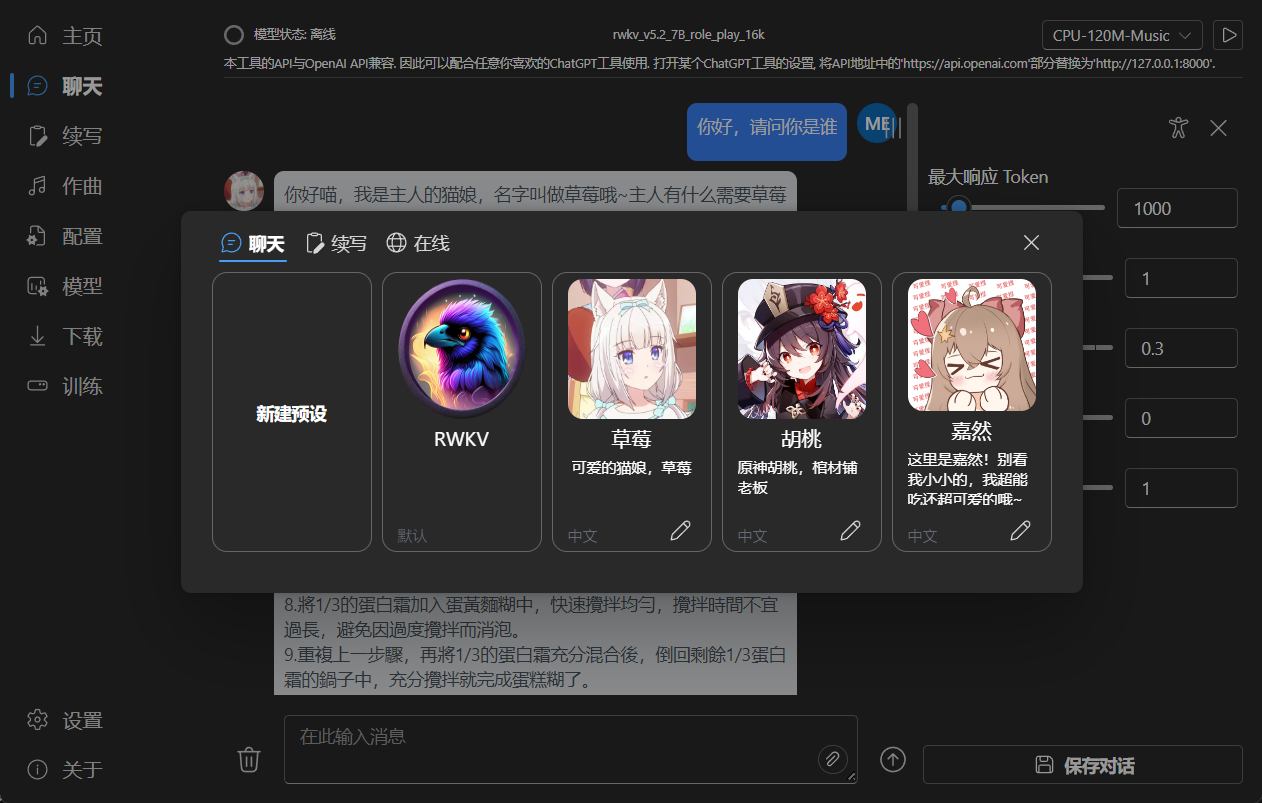
|
||||

|
||||
|
||||
### 续写
|
||||
|
||||
|
@ -1,15 +1,22 @@
|
||||
package backend_golang
|
||||
|
||||
import (
|
||||
"archive/zip"
|
||||
"bufio"
|
||||
"bytes"
|
||||
"context"
|
||||
"errors"
|
||||
"io"
|
||||
"log"
|
||||
"net"
|
||||
"net/http"
|
||||
"net/http/httputil"
|
||||
"net/url"
|
||||
"os"
|
||||
"os/exec"
|
||||
"path/filepath"
|
||||
"runtime"
|
||||
"strings"
|
||||
"syscall"
|
||||
"time"
|
||||
|
||||
@ -23,6 +30,8 @@ type App struct {
|
||||
ctx context.Context
|
||||
HasConfigData bool
|
||||
ConfigData map[string]any
|
||||
Dev bool
|
||||
proxyPort int
|
||||
exDir string
|
||||
cmdPrefix string
|
||||
}
|
||||
@ -32,6 +41,63 @@ func NewApp() *App {
|
||||
return &App{}
|
||||
}
|
||||
|
||||
func (a *App) newFetchProxy() {
|
||||
go func() {
|
||||
handler := func(w http.ResponseWriter, r *http.Request) {
|
||||
if r.Method == "OPTIONS" {
|
||||
w.Header().Set("Access-Control-Allow-Methods", "GET, POST, OPTIONS")
|
||||
w.Header().Set("Access-Control-Allow-Headers", "*")
|
||||
w.Header().Set("Access-Control-Allow-Origin", "*")
|
||||
return
|
||||
}
|
||||
proxy := &httputil.ReverseProxy{
|
||||
ModifyResponse: func(res *http.Response) error {
|
||||
res.Header.Set("Access-Control-Allow-Origin", "*")
|
||||
return nil
|
||||
},
|
||||
Director: func(req *http.Request) {
|
||||
realTarget := req.Header.Get("Real-Target")
|
||||
if realTarget != "" {
|
||||
realTarget, err := url.PathUnescape(realTarget)
|
||||
if err != nil {
|
||||
log.Printf("Error decoding target URL: %v\n", err)
|
||||
return
|
||||
}
|
||||
target, err := url.Parse(realTarget)
|
||||
if err != nil {
|
||||
log.Printf("Error parsing target URL: %v\n", err)
|
||||
return
|
||||
}
|
||||
req.Header.Set("Accept", "*/*")
|
||||
req.Header.Del("Origin")
|
||||
req.Header.Del("Referer")
|
||||
req.Header.Del("Real-Target")
|
||||
req.Header.Del("Sec-Fetch-Dest")
|
||||
req.Header.Del("Sec-Fetch-Mode")
|
||||
req.Header.Del("Sec-Fetch-Site")
|
||||
req.URL.Scheme = target.Scheme
|
||||
req.URL.Host = target.Host
|
||||
req.URL.Path = target.Path
|
||||
req.URL.RawQuery = url.PathEscape(target.RawQuery)
|
||||
log.Println("Proxying to", realTarget)
|
||||
} else {
|
||||
log.Println("Real-Target header is missing")
|
||||
}
|
||||
},
|
||||
}
|
||||
proxy.ServeHTTP(w, r)
|
||||
}
|
||||
http.HandleFunc("/", handler)
|
||||
listener, err := net.Listen("tcp", "127.0.0.1:0")
|
||||
if err != nil {
|
||||
return
|
||||
}
|
||||
a.proxyPort = listener.Addr().(*net.TCPAddr).Port
|
||||
|
||||
http.Serve(listener, nil)
|
||||
}()
|
||||
}
|
||||
|
||||
// startup is called when the app starts. The context is saved
|
||||
// so we can call the runtime methods
|
||||
func (a *App) OnStartup(ctx context.Context) {
|
||||
@ -39,26 +105,41 @@ func (a *App) OnStartup(ctx context.Context) {
|
||||
a.exDir = ""
|
||||
a.cmdPrefix = ""
|
||||
|
||||
if runtime.GOOS == "darwin" {
|
||||
ex, _ := os.Executable()
|
||||
a.exDir = filepath.Dir(ex) + "/../../../"
|
||||
a.cmdPrefix = "cd " + a.exDir + " && "
|
||||
ex, err := os.Executable()
|
||||
if err == nil {
|
||||
if runtime.GOOS == "darwin" {
|
||||
a.exDir = filepath.Dir(ex) + "/../../../"
|
||||
a.cmdPrefix = "cd " + a.exDir + " && "
|
||||
} else {
|
||||
a.exDir = filepath.Dir(ex) + "/"
|
||||
a.cmdPrefix = "cd " + a.exDir + " && "
|
||||
}
|
||||
if a.Dev {
|
||||
a.exDir = ""
|
||||
} else {
|
||||
os.Chdir(a.exDir)
|
||||
}
|
||||
}
|
||||
|
||||
os.Chmod(a.exDir+"backend-rust/webgpu_server", 0777)
|
||||
os.Chmod(a.exDir+"backend-rust/web-rwkv-converter", 0777)
|
||||
os.Mkdir(a.exDir+"models", os.ModePerm)
|
||||
os.Mkdir(a.exDir+"lora-models", os.ModePerm)
|
||||
os.Mkdir(a.exDir+"state-models", os.ModePerm)
|
||||
os.Mkdir(a.exDir+"finetune/json2binidx_tool/data", os.ModePerm)
|
||||
f, err := os.Create(a.exDir + "lora-models/train_log.txt")
|
||||
if err == nil {
|
||||
f.Close()
|
||||
trainLogPath := "lora-models/train_log.txt"
|
||||
if !a.FileExists(trainLogPath) {
|
||||
f, err := os.Create(a.exDir + trainLogPath)
|
||||
if err == nil {
|
||||
f.Close()
|
||||
}
|
||||
}
|
||||
|
||||
a.downloadLoop()
|
||||
a.midiLoop()
|
||||
a.watchFs()
|
||||
a.monitorHardware()
|
||||
a.newFetchProxy()
|
||||
}
|
||||
|
||||
func (a *App) OnBeforeClose(ctx context.Context) bool {
|
||||
@ -71,8 +152,9 @@ func (a *App) OnBeforeClose(ctx context.Context) bool {
|
||||
func (a *App) watchFs() {
|
||||
watcher, err := fsnotify.NewWatcher()
|
||||
if err == nil {
|
||||
watcher.Add(a.exDir + "./lora-models")
|
||||
watcher.Add(a.exDir + "./models")
|
||||
watcher.Add(a.exDir + "./lora-models")
|
||||
watcher.Add(a.exDir + "./state-models")
|
||||
go func() {
|
||||
for {
|
||||
select {
|
||||
@ -146,6 +228,7 @@ func (a *App) UpdateApp(url string) (broken bool, err error) {
|
||||
ticker := time.NewTicker(250 * time.Millisecond)
|
||||
defer ticker.Stop()
|
||||
|
||||
// update progress
|
||||
go func() {
|
||||
for {
|
||||
<-ticker.C
|
||||
@ -165,13 +248,35 @@ func (a *App) UpdateApp(url string) (broken bool, err error) {
|
||||
}
|
||||
}
|
||||
}()
|
||||
err = selfupdate.Apply(pr, selfupdate.Options{})
|
||||
|
||||
var updateFile io.Reader = pr
|
||||
// extract macos binary from zip
|
||||
if strings.HasSuffix(url, ".zip") && runtime.GOOS == "darwin" {
|
||||
zipBytes, err := io.ReadAll(pr)
|
||||
if err != nil {
|
||||
return false, err
|
||||
}
|
||||
archive, err := zip.NewReader(bytes.NewReader(zipBytes), int64(len(zipBytes)))
|
||||
if err != nil {
|
||||
return false, err
|
||||
}
|
||||
file, err := archive.Open("RWKV-Runner.app/Contents/MacOS/RWKV-Runner")
|
||||
if err != nil {
|
||||
return false, err
|
||||
}
|
||||
defer file.Close()
|
||||
updateFile = file
|
||||
}
|
||||
|
||||
// apply update
|
||||
err = selfupdate.Apply(updateFile, selfupdate.Options{})
|
||||
if err != nil {
|
||||
if rerr := selfupdate.RollbackError(err); rerr != nil {
|
||||
return true, rerr
|
||||
}
|
||||
return false, err
|
||||
}
|
||||
// restart app
|
||||
if runtime.GOOS == "windows" {
|
||||
name, err := os.Executable()
|
||||
if err != nil {
|
||||
@ -199,3 +304,7 @@ func (a *App) RestartApp() error {
|
||||
func (a *App) GetPlatform() string {
|
||||
return runtime.GOOS
|
||||
}
|
||||
|
||||
func (a *App) GetProxyPort() int {
|
||||
return a.proxyPort
|
||||
}
|
||||
|
@ -10,7 +10,11 @@ import (
|
||||
)
|
||||
|
||||
func (a *App) DownloadFile(path string, url string) error {
|
||||
_, err := grab.Get(a.exDir+path, url)
|
||||
absPath, err := a.GetAbsPath(path)
|
||||
if err != nil {
|
||||
return err
|
||||
}
|
||||
_, err = grab.Get(absPath, url)
|
||||
if err != nil {
|
||||
return err
|
||||
}
|
||||
@ -88,11 +92,15 @@ func (a *App) ContinueDownload(url string) {
|
||||
}
|
||||
|
||||
func (a *App) AddToDownloadList(path string, url string) {
|
||||
if !existsInDownloadList(a.exDir+path, url) {
|
||||
absPath, err := a.GetAbsPath(path)
|
||||
if err != nil {
|
||||
return
|
||||
}
|
||||
if !existsInDownloadList(absPath, url) {
|
||||
downloadList = append(downloadList, &DownloadStatus{
|
||||
resp: nil,
|
||||
Name: filepath.Base(path),
|
||||
Path: a.exDir + path,
|
||||
Path: absPath,
|
||||
Url: url,
|
||||
Downloading: false,
|
||||
})
|
||||
|
@ -14,27 +14,55 @@ import (
|
||||
wruntime "github.com/wailsapp/wails/v2/pkg/runtime"
|
||||
)
|
||||
|
||||
func (a *App) GetAbsPath(path string) (string, error) {
|
||||
var absPath string
|
||||
var err error
|
||||
if filepath.IsAbs(path) {
|
||||
absPath = filepath.Clean(path)
|
||||
} else {
|
||||
absPath, err = filepath.Abs(filepath.Join(a.exDir, path))
|
||||
if err != nil {
|
||||
return "", err
|
||||
}
|
||||
}
|
||||
absPath = strings.ReplaceAll(absPath, "/", string(os.PathSeparator))
|
||||
println("GetAbsPath:", absPath)
|
||||
return absPath, nil
|
||||
}
|
||||
|
||||
func (a *App) SaveFile(path string, savedContent []byte) error {
|
||||
if err := os.WriteFile(a.exDir+path, savedContent, 0644); err != nil {
|
||||
absPath, err := a.GetAbsPath(path)
|
||||
if err != nil {
|
||||
return err
|
||||
}
|
||||
if err := os.WriteFile(absPath, savedContent, 0644); err != nil {
|
||||
return err
|
||||
}
|
||||
return nil
|
||||
}
|
||||
|
||||
func (a *App) SaveJson(fileName string, jsonData any) error {
|
||||
func (a *App) SaveJson(path string, jsonData any) error {
|
||||
text, err := json.MarshalIndent(jsonData, "", " ")
|
||||
if err != nil {
|
||||
return err
|
||||
}
|
||||
|
||||
if err := os.WriteFile(a.exDir+fileName, text, 0644); err != nil {
|
||||
absPath, err := a.GetAbsPath(path)
|
||||
if err != nil {
|
||||
return err
|
||||
}
|
||||
if err := os.WriteFile(absPath, text, 0644); err != nil {
|
||||
return err
|
||||
}
|
||||
return nil
|
||||
}
|
||||
|
||||
func (a *App) ReadJson(fileName string) (any, error) {
|
||||
file, err := os.ReadFile(a.exDir + fileName)
|
||||
func (a *App) ReadJson(path string) (any, error) {
|
||||
absPath, err := a.GetAbsPath(path)
|
||||
if err != nil {
|
||||
return nil, err
|
||||
}
|
||||
file, err := os.ReadFile(absPath)
|
||||
if err != nil {
|
||||
return nil, err
|
||||
}
|
||||
@ -48,8 +76,12 @@ func (a *App) ReadJson(fileName string) (any, error) {
|
||||
return data, nil
|
||||
}
|
||||
|
||||
func (a *App) FileExists(fileName string) bool {
|
||||
_, err := os.Stat(a.exDir + fileName)
|
||||
func (a *App) FileExists(path string) bool {
|
||||
absPath, err := a.GetAbsPath(path)
|
||||
if err != nil {
|
||||
return false
|
||||
}
|
||||
_, err = os.Stat(absPath)
|
||||
return err == nil
|
||||
}
|
||||
|
||||
@ -60,8 +92,12 @@ type FileInfo struct {
|
||||
ModTime string `json:"modTime"`
|
||||
}
|
||||
|
||||
func (a *App) ReadFileInfo(fileName string) (*FileInfo, error) {
|
||||
info, err := os.Stat(a.exDir + fileName)
|
||||
func (a *App) ReadFileInfo(path string) (*FileInfo, error) {
|
||||
absPath, err := a.GetAbsPath(path)
|
||||
if err != nil {
|
||||
return nil, err
|
||||
}
|
||||
info, err := os.Stat(absPath)
|
||||
if err != nil {
|
||||
return nil, err
|
||||
}
|
||||
@ -74,7 +110,11 @@ func (a *App) ReadFileInfo(fileName string) (*FileInfo, error) {
|
||||
}
|
||||
|
||||
func (a *App) ListDirFiles(dirPath string) ([]FileInfo, error) {
|
||||
files, err := os.ReadDir(a.exDir + dirPath)
|
||||
absDirPath, err := a.GetAbsPath(dirPath)
|
||||
if err != nil {
|
||||
return nil, err
|
||||
}
|
||||
files, err := os.ReadDir(absDirPath)
|
||||
if err != nil {
|
||||
return nil, err
|
||||
}
|
||||
@ -96,7 +136,11 @@ func (a *App) ListDirFiles(dirPath string) ([]FileInfo, error) {
|
||||
}
|
||||
|
||||
func (a *App) DeleteFile(path string) error {
|
||||
err := os.Remove(a.exDir + path)
|
||||
absPath, err := a.GetAbsPath(path)
|
||||
if err != nil {
|
||||
return err
|
||||
}
|
||||
err = os.Remove(absPath)
|
||||
if err != nil {
|
||||
return err
|
||||
}
|
||||
@ -104,18 +148,27 @@ func (a *App) DeleteFile(path string) error {
|
||||
}
|
||||
|
||||
func (a *App) CopyFile(src string, dst string) error {
|
||||
sourceFile, err := os.Open(a.exDir + src)
|
||||
absSrc, err := a.GetAbsPath(src)
|
||||
if err != nil {
|
||||
return err
|
||||
}
|
||||
absDst, err := a.GetAbsPath(dst)
|
||||
if err != nil {
|
||||
return err
|
||||
}
|
||||
|
||||
sourceFile, err := os.Open(absSrc)
|
||||
if err != nil {
|
||||
return err
|
||||
}
|
||||
defer sourceFile.Close()
|
||||
|
||||
err = os.MkdirAll(a.exDir+dst[:strings.LastIndex(dst, "/")], 0755)
|
||||
err = os.MkdirAll(filepath.Dir(absDst), 0755)
|
||||
if err != nil {
|
||||
return err
|
||||
}
|
||||
|
||||
destFile, err := os.Create(a.exDir + dst)
|
||||
destFile, err := os.Create(absDst)
|
||||
if err != nil {
|
||||
return err
|
||||
}
|
||||
@ -166,14 +219,8 @@ func (a *App) OpenOpenFileDialog(filterPattern string) (string, error) {
|
||||
return path, nil
|
||||
}
|
||||
|
||||
func (a *App) OpenFileFolder(path string, relative bool) error {
|
||||
var absPath string
|
||||
var err error
|
||||
if relative {
|
||||
absPath, err = filepath.Abs(a.exDir + path)
|
||||
} else {
|
||||
absPath, err = filepath.Abs(path)
|
||||
}
|
||||
func (a *App) OpenFileFolder(path string) error {
|
||||
absPath, err := a.GetAbsPath(path)
|
||||
if err != nil {
|
||||
return err
|
||||
}
|
||||
|
@ -1,3 +1,4 @@
|
||||
// Considering some whitespace and multilingual support, the functions in rwkv.go should always be executed with cwd as RWKV-Runner, and never use a.GetAbsPath() here.
|
||||
package backend_golang
|
||||
|
||||
import (
|
||||
@ -11,19 +12,23 @@ import (
|
||||
)
|
||||
|
||||
func (a *App) StartServer(python string, port int, host string, webui bool, rwkvBeta bool, rwkvcpp bool, webgpu bool) (string, error) {
|
||||
var err error
|
||||
execFile := "./backend-python/main.py"
|
||||
_, err := os.Stat(execFile)
|
||||
if err != nil {
|
||||
return "", err
|
||||
}
|
||||
if python == "" {
|
||||
python, err = GetPython()
|
||||
}
|
||||
if err != nil {
|
||||
return "", err
|
||||
}
|
||||
args := []string{python, "./backend-python/main.py"}
|
||||
args := []string{python, execFile}
|
||||
if webui {
|
||||
args = append(args, "--webui")
|
||||
}
|
||||
if rwkvBeta {
|
||||
args = append(args, "--rwkv-beta")
|
||||
// args = append(args, "--rwkv-beta")
|
||||
}
|
||||
if rwkvcpp {
|
||||
args = append(args, "--rwkv.cpp")
|
||||
@ -36,41 +41,77 @@ func (a *App) StartServer(python string, port int, host string, webui bool, rwkv
|
||||
}
|
||||
|
||||
func (a *App) StartWebGPUServer(port int, host string) (string, error) {
|
||||
args := []string{"./backend-rust/webgpu_server"}
|
||||
var execFile string
|
||||
execFiles := []string{"./backend-rust/webgpu_server", "./backend-rust/webgpu_server.exe"}
|
||||
for _, file := range execFiles {
|
||||
_, err := os.Stat(file)
|
||||
if err == nil {
|
||||
execFile = file
|
||||
break
|
||||
}
|
||||
}
|
||||
if execFile == "" {
|
||||
return "", errors.New(execFiles[0] + " not found")
|
||||
}
|
||||
args := []string{execFile}
|
||||
args = append(args, "--port", strconv.Itoa(port), "--ip", host)
|
||||
return Cmd(args...)
|
||||
}
|
||||
|
||||
func (a *App) ConvertModel(python string, modelPath string, strategy string, outPath string) (string, error) {
|
||||
var err error
|
||||
execFile := "./backend-python/convert_model.py"
|
||||
_, err := os.Stat(execFile)
|
||||
if err != nil {
|
||||
return "", err
|
||||
}
|
||||
if python == "" {
|
||||
python, err = GetPython()
|
||||
}
|
||||
if err != nil {
|
||||
return "", err
|
||||
}
|
||||
return Cmd(python, "./backend-python/convert_model.py", "--in", modelPath, "--out", outPath, "--strategy", strategy)
|
||||
return Cmd(python, execFile, "--in", modelPath, "--out", outPath, "--strategy", strategy)
|
||||
}
|
||||
|
||||
func (a *App) ConvertSafetensors(modelPath string, outPath string) (string, error) {
|
||||
args := []string{"./backend-rust/web-rwkv-converter"}
|
||||
var execFile string
|
||||
execFiles := []string{"./backend-rust/web-rwkv-converter", "./backend-rust/web-rwkv-converter.exe"}
|
||||
for _, file := range execFiles {
|
||||
_, err := os.Stat(file)
|
||||
if err == nil {
|
||||
execFile = file
|
||||
break
|
||||
}
|
||||
}
|
||||
if execFile == "" {
|
||||
return "", errors.New(execFiles[0] + " not found")
|
||||
}
|
||||
args := []string{execFile}
|
||||
args = append(args, "--input", modelPath, "--output", outPath)
|
||||
return Cmd(args...)
|
||||
}
|
||||
|
||||
func (a *App) ConvertSafetensorsWithPython(python string, modelPath string, outPath string) (string, error) {
|
||||
var err error
|
||||
execFile := "./backend-python/convert_safetensors.py"
|
||||
_, err := os.Stat(execFile)
|
||||
if err != nil {
|
||||
return "", err
|
||||
}
|
||||
if python == "" {
|
||||
python, err = GetPython()
|
||||
}
|
||||
if err != nil {
|
||||
return "", err
|
||||
}
|
||||
return Cmd(python, "./backend-python/convert_safetensors.py", "--input", modelPath, "--output", outPath)
|
||||
return Cmd(python, execFile, "--input", modelPath, "--output", outPath)
|
||||
}
|
||||
|
||||
func (a *App) ConvertGGML(python string, modelPath string, outPath string, Q51 bool) (string, error) {
|
||||
var err error
|
||||
execFile := "./backend-python/convert_pytorch_to_ggml.py"
|
||||
_, err := os.Stat(execFile)
|
||||
if err != nil {
|
||||
return "", err
|
||||
}
|
||||
if python == "" {
|
||||
python, err = GetPython()
|
||||
}
|
||||
@ -81,11 +122,15 @@ func (a *App) ConvertGGML(python string, modelPath string, outPath string, Q51 b
|
||||
if Q51 {
|
||||
dataType = "Q5_1"
|
||||
}
|
||||
return Cmd(python, "./backend-python/convert_pytorch_to_ggml.py", modelPath, outPath, dataType)
|
||||
return Cmd(python, execFile, modelPath, outPath, dataType)
|
||||
}
|
||||
|
||||
func (a *App) ConvertData(python string, input string, outputPrefix string, vocab string) (string, error) {
|
||||
var err error
|
||||
execFile := "./finetune/json2binidx_tool/tools/preprocess_data.py"
|
||||
_, err := os.Stat(execFile)
|
||||
if err != nil {
|
||||
return "", err
|
||||
}
|
||||
if python == "" {
|
||||
python, err = GetPython()
|
||||
}
|
||||
@ -129,19 +174,23 @@ func (a *App) ConvertData(python string, input string, outputPrefix string, voca
|
||||
return "", err
|
||||
}
|
||||
|
||||
return Cmd(python, "./finetune/json2binidx_tool/tools/preprocess_data.py", "--input", input, "--output-prefix", outputPrefix, "--vocab", vocab,
|
||||
return Cmd(python, execFile, "--input", input, "--output-prefix", outputPrefix, "--vocab", vocab,
|
||||
"--tokenizer-type", tokenizerType, "--dataset-impl", "mmap", "--append-eod")
|
||||
}
|
||||
|
||||
func (a *App) MergeLora(python string, useGpu bool, loraAlpha int, baseModel string, loraPath string, outputPath string) (string, error) {
|
||||
var err error
|
||||
execFile := "./finetune/lora/merge_lora.py"
|
||||
_, err := os.Stat(execFile)
|
||||
if err != nil {
|
||||
return "", err
|
||||
}
|
||||
if python == "" {
|
||||
python, err = GetPython()
|
||||
}
|
||||
if err != nil {
|
||||
return "", err
|
||||
}
|
||||
args := []string{python, "./finetune/lora/merge_lora.py"}
|
||||
args := []string{python, execFile}
|
||||
if useGpu {
|
||||
args = append(args, "--use-gpu")
|
||||
}
|
||||
@ -157,17 +206,21 @@ func (a *App) DepCheck(python string) error {
|
||||
if err != nil {
|
||||
return err
|
||||
}
|
||||
out, err := exec.Command(python, a.exDir+"./backend-python/dep_check.py").CombinedOutput()
|
||||
out, err := exec.Command(python, a.exDir+"backend-python/dep_check.py").CombinedOutput()
|
||||
if err != nil {
|
||||
return errors.New("DepCheck Error: " + string(out))
|
||||
return errors.New("DepCheck Error: " + string(out) + " GError: " + err.Error())
|
||||
}
|
||||
return nil
|
||||
}
|
||||
|
||||
func (a *App) InstallPyDep(python string, cnMirror bool) (string, error) {
|
||||
var err error
|
||||
torchWhlUrl := "torch==1.13.1 torchvision==0.14.1 torchaudio==0.13.1 --index-url https://download.pytorch.org/whl/cu117"
|
||||
if python == "" {
|
||||
python, err = GetPython()
|
||||
if cnMirror && python == "py310/python.exe" {
|
||||
torchWhlUrl = "https://mirrors.aliyun.com/pytorch-wheels/cu117/torch-1.13.1+cu117-cp310-cp310-win_amd64.whl"
|
||||
}
|
||||
if runtime.GOOS == "windows" {
|
||||
python = `"%CD%/` + python + `"`
|
||||
}
|
||||
@ -178,14 +231,14 @@ func (a *App) InstallPyDep(python string, cnMirror bool) (string, error) {
|
||||
|
||||
if runtime.GOOS == "windows" {
|
||||
ChangeFileLine("./py310/python310._pth", 3, "Lib\\site-packages")
|
||||
installScript := python + " ./backend-python/get-pip.py -i https://pypi.tuna.tsinghua.edu.cn/simple --no-warn-script-location\n" +
|
||||
python + " -m pip install torch==1.13.1 torchvision==0.14.1 torchaudio==0.13.1 --index-url https://download.pytorch.org/whl/cu117 --no-warn-script-location\n" +
|
||||
python + " -m pip install -r ./backend-python/requirements.txt -i https://pypi.tuna.tsinghua.edu.cn/simple --no-warn-script-location\n" +
|
||||
installScript := python + " ./backend-python/get-pip.py -i https://mirrors.aliyun.com/pypi/simple --no-warn-script-location\n" +
|
||||
python + " -m pip install " + torchWhlUrl + " --no-warn-script-location\n" +
|
||||
python + " -m pip install -r ./backend-python/requirements.txt -i https://mirrors.aliyun.com/pypi/simple --no-warn-script-location\n" +
|
||||
"exit"
|
||||
if !cnMirror {
|
||||
installScript = strings.Replace(installScript, " -i https://pypi.tuna.tsinghua.edu.cn/simple", "", -1)
|
||||
installScript = strings.Replace(installScript, " -i https://mirrors.aliyun.com/pypi/simple", "", -1)
|
||||
}
|
||||
err = os.WriteFile("./install-py-dep.bat", []byte(installScript), 0644)
|
||||
err = os.WriteFile(a.exDir+"install-py-dep.bat", []byte(installScript), 0644)
|
||||
if err != nil {
|
||||
return "", err
|
||||
}
|
||||
@ -193,7 +246,7 @@ func (a *App) InstallPyDep(python string, cnMirror bool) (string, error) {
|
||||
}
|
||||
|
||||
if cnMirror {
|
||||
return Cmd(python, "-m", "pip", "install", "-r", "./backend-python/requirements_without_cyac.txt", "-i", "https://pypi.tuna.tsinghua.edu.cn/simple")
|
||||
return Cmd(python, "-m", "pip", "install", "-r", "./backend-python/requirements_without_cyac.txt", "-i", "https://mirrors.aliyun.com/pypi/simple")
|
||||
} else {
|
||||
return Cmd(python, "-m", "pip", "install", "-r", "./backend-python/requirements_without_cyac.txt")
|
||||
}
|
||||
|
@ -3,6 +3,7 @@ package backend_golang
|
||||
import (
|
||||
"archive/zip"
|
||||
"bufio"
|
||||
"crypto/sha256"
|
||||
"embed"
|
||||
"errors"
|
||||
"fmt"
|
||||
@ -22,14 +23,19 @@ func CmdHelper(hideWindow bool, args ...string) (*exec.Cmd, error) {
|
||||
if runtime.GOOS != "windows" {
|
||||
return nil, errors.New("unsupported OS")
|
||||
}
|
||||
filename := "./cmd-helper.bat"
|
||||
_, err := os.Stat(filename)
|
||||
ex, err := os.Executable()
|
||||
if err != nil {
|
||||
if err := os.WriteFile(filename, []byte("start %*"), 0644); err != nil {
|
||||
return nil, err
|
||||
}
|
||||
exDir := filepath.Dir(ex) + "/"
|
||||
path := exDir + "cmd-helper.bat"
|
||||
_, err = os.Stat(path)
|
||||
if err != nil {
|
||||
if err := os.WriteFile(path, []byte("start %*"), 0644); err != nil {
|
||||
return nil, err
|
||||
}
|
||||
}
|
||||
cmdHelper, err := filepath.Abs(filename)
|
||||
cmdHelper, err := filepath.Abs(path)
|
||||
if err != nil {
|
||||
return nil, err
|
||||
}
|
||||
@ -85,16 +91,18 @@ func Cmd(args ...string) (string, error) {
|
||||
}
|
||||
|
||||
func CopyEmbed(efs embed.FS) error {
|
||||
prefix := ""
|
||||
ex, err := os.Executable()
|
||||
if err != nil {
|
||||
return err
|
||||
}
|
||||
var prefix string
|
||||
if runtime.GOOS == "darwin" {
|
||||
ex, err := os.Executable()
|
||||
if err != nil {
|
||||
return err
|
||||
}
|
||||
prefix = filepath.Dir(ex) + "/../../../"
|
||||
} else {
|
||||
prefix = filepath.Dir(ex) + "/"
|
||||
}
|
||||
|
||||
err := fs.WalkDir(efs, ".", func(path string, d fs.DirEntry, err error) error {
|
||||
err = fs.WalkDir(efs, ".", func(path string, d fs.DirEntry, err error) error {
|
||||
if d.IsDir() {
|
||||
return nil
|
||||
}
|
||||
@ -112,9 +120,19 @@ func CopyEmbed(efs embed.FS) error {
|
||||
return err
|
||||
}
|
||||
|
||||
err = os.WriteFile(path, content, 0644)
|
||||
if err != nil {
|
||||
return err
|
||||
executeWrite := true
|
||||
existedContent, err := os.ReadFile(path)
|
||||
if err == nil {
|
||||
if fmt.Sprintf("%x", sha256.Sum256(existedContent)) == fmt.Sprintf("%x", sha256.Sum256(content)) {
|
||||
executeWrite = false
|
||||
}
|
||||
}
|
||||
|
||||
if executeWrite {
|
||||
err = os.WriteFile(path, content, 0644)
|
||||
if err != nil {
|
||||
return err
|
||||
}
|
||||
}
|
||||
|
||||
return nil
|
||||
@ -125,13 +143,19 @@ func CopyEmbed(efs embed.FS) error {
|
||||
func GetPython() (string, error) {
|
||||
switch platform := runtime.GOOS; platform {
|
||||
case "windows":
|
||||
_, err := os.Stat("py310/python.exe")
|
||||
ex, err := os.Executable()
|
||||
if err != nil {
|
||||
_, err := os.Stat("python-3.10.11-embed-amd64.zip")
|
||||
return "", err
|
||||
}
|
||||
exDir := filepath.Dir(ex) + "/"
|
||||
pyexe := exDir + "py310/python.exe"
|
||||
_, err = os.Stat(pyexe)
|
||||
if err != nil {
|
||||
_, err := os.Stat(exDir + "python-3.10.11-embed-amd64.zip")
|
||||
if err != nil {
|
||||
return "", errors.New("python zip not found")
|
||||
} else {
|
||||
err := Unzip("python-3.10.11-embed-amd64.zip", "py310")
|
||||
err := Unzip(exDir+"python-3.10.11-embed-amd64.zip", exDir+"py310")
|
||||
if err != nil {
|
||||
return "", errors.New("failed to unzip python")
|
||||
} else {
|
||||
|
@ -9,7 +9,6 @@ import (
|
||||
"io"
|
||||
"os"
|
||||
"os/exec"
|
||||
"path/filepath"
|
||||
"strings"
|
||||
"time"
|
||||
|
||||
@ -133,26 +132,20 @@ func (a *App) WslStop() error {
|
||||
}
|
||||
|
||||
func (a *App) WslIsEnabled() error {
|
||||
ex, err := os.Executable()
|
||||
if err != nil {
|
||||
return err
|
||||
}
|
||||
exDir := filepath.Dir(ex)
|
||||
|
||||
data, err := os.ReadFile(exDir + "/wsl.state")
|
||||
data, err := os.ReadFile(a.exDir + "wsl.state")
|
||||
if err == nil {
|
||||
if strings.Contains(string(data), "Enabled") {
|
||||
return nil
|
||||
}
|
||||
}
|
||||
|
||||
cmd := `-Command (Get-WindowsOptionalFeature -Online -FeatureName Microsoft-Windows-Subsystem-Linux).State | Out-File -Encoding utf8 -FilePath ` + exDir + "/wsl.state"
|
||||
_, err = su.ShellExecute(su.RUNAS, "powershell", cmd, exDir)
|
||||
cmd := `-Command (Get-WindowsOptionalFeature -Online -FeatureName VirtualMachinePlatform).State | Out-File -Encoding utf8 -FilePath ` + a.exDir + "wsl.state"
|
||||
_, err = su.ShellExecute(su.RUNAS, "powershell", cmd, a.exDir)
|
||||
if err != nil {
|
||||
return err
|
||||
}
|
||||
time.Sleep(2 * time.Second)
|
||||
data, err = os.ReadFile(exDir + "/wsl.state")
|
||||
data, err = os.ReadFile(a.exDir + "wsl.state")
|
||||
if err != nil {
|
||||
return err
|
||||
}
|
||||
@ -164,13 +157,13 @@ func (a *App) WslIsEnabled() error {
|
||||
}
|
||||
|
||||
func (a *App) WslEnable(forceMode bool) error {
|
||||
cmd := `/online /enable-feature /featurename:Microsoft-Windows-Subsystem-Linux`
|
||||
cmd := `/online /enable-feature /featurename:VirtualMachinePlatform`
|
||||
_, err := su.ShellExecute(su.RUNAS, "dism", cmd, `C:\`)
|
||||
if err != nil {
|
||||
return err
|
||||
}
|
||||
if forceMode {
|
||||
os.WriteFile("./wsl.state", []byte("Enabled"), 0644)
|
||||
os.WriteFile(a.exDir+"wsl.state", []byte("Enabled"), 0644)
|
||||
}
|
||||
return nil
|
||||
}
|
||||
|
66
backend-python/convert_safetensors.py
vendored
66
backend-python/convert_safetensors.py
vendored
@ -1,9 +1,8 @@
|
||||
import json
|
||||
import collections
|
||||
import numpy
|
||||
import os
|
||||
import sys
|
||||
import copy
|
||||
import torch
|
||||
from safetensors.torch import load_file, save_file
|
||||
from safetensors.torch import serialize_file, load_file
|
||||
|
||||
import argparse
|
||||
|
||||
@ -26,7 +25,7 @@ def rename_key(rename, name):
|
||||
|
||||
|
||||
def convert_file(pt_filename: str, sf_filename: str, rename={}, transpose_names=[]):
|
||||
loaded = torch.load(pt_filename, map_location="cpu")
|
||||
loaded: collections.OrderedDict = torch.load(pt_filename, map_location="cpu")
|
||||
if "state_dict" in loaded:
|
||||
loaded = loaded["state_dict"]
|
||||
|
||||
@ -44,11 +43,9 @@ def convert_file(pt_filename: str, sf_filename: str, rename={}, transpose_names=
|
||||
if "time_maa" in x:
|
||||
version = max(6, version)
|
||||
|
||||
if version == 5.1 and "midi" in pt_filename.lower():
|
||||
import numpy as np
|
||||
print(f"Model detected: v{version:.1f}")
|
||||
|
||||
np.set_printoptions(precision=4, suppress=True, linewidth=200)
|
||||
kk = list(loaded.keys())
|
||||
if version == 5.1:
|
||||
_, n_emb = loaded["emb.weight"].shape
|
||||
for k in kk:
|
||||
if "time_decay" in k or "time_faaaa" in k:
|
||||
@ -57,31 +54,36 @@ def convert_file(pt_filename: str, sf_filename: str, rename={}, transpose_names=
|
||||
loaded[k].unsqueeze(1).repeat(1, n_emb // loaded[k].shape[0])
|
||||
)
|
||||
|
||||
loaded = {k: v.clone().half() for k, v in loaded.items()}
|
||||
# for k, v in loaded.items():
|
||||
# print(f'{k}\t{v.shape}\t{v.dtype}')
|
||||
|
||||
loaded = {rename_key(rename, k).lower(): v.contiguous() for k, v in loaded.items()}
|
||||
# For tensors to be contiguous
|
||||
for k, v in loaded.items():
|
||||
for transpose_name in transpose_names:
|
||||
if transpose_name in k:
|
||||
loaded[k] = v.transpose(0, 1)
|
||||
|
||||
loaded = {k: v.clone().half().contiguous() for k, v in loaded.items()}
|
||||
|
||||
for k, v in loaded.items():
|
||||
print(f"{k}\t{v.shape}\t{v.dtype}")
|
||||
with torch.no_grad():
|
||||
for k in kk:
|
||||
new_k = rename_key(rename, k).lower()
|
||||
v = loaded[k].half()
|
||||
del loaded[k]
|
||||
for transpose_name in transpose_names:
|
||||
if transpose_name in new_k:
|
||||
dims = len(v.shape)
|
||||
v = v.transpose(dims - 2, dims - 1)
|
||||
print(f"{new_k}\t{v.shape}\t{v.dtype}")
|
||||
loaded[new_k] = {
|
||||
"dtype": str(v.dtype).split(".")[-1],
|
||||
"shape": v.shape,
|
||||
"data": v.numpy().tobytes(),
|
||||
}
|
||||
|
||||
dirname = os.path.dirname(sf_filename)
|
||||
os.makedirs(dirname, exist_ok=True)
|
||||
save_file(loaded, sf_filename, metadata={"format": "pt"})
|
||||
reloaded = load_file(sf_filename)
|
||||
for k in loaded:
|
||||
pt_tensor = loaded[k]
|
||||
sf_tensor = reloaded[k]
|
||||
if not torch.equal(pt_tensor, sf_tensor):
|
||||
raise RuntimeError(f"The output tensors do not match for key {k}")
|
||||
serialize_file(loaded, sf_filename, metadata={"format": "pt"})
|
||||
# reloaded = load_file(sf_filename)
|
||||
# for k in loaded:
|
||||
# pt_tensor = torch.Tensor(
|
||||
# numpy.frombuffer(
|
||||
# bytearray(loaded[k]["data"]),
|
||||
# dtype=getattr(numpy, loaded[k]["dtype"]),
|
||||
# ).reshape(loaded[k]["shape"])
|
||||
# )
|
||||
# sf_tensor = reloaded[k]
|
||||
# if not torch.equal(pt_tensor, sf_tensor):
|
||||
# raise RuntimeError(f"The output tensors do not match for key {k}")
|
||||
|
||||
|
||||
if __name__ == "__main__":
|
||||
@ -100,6 +102,8 @@ if __name__ == "__main__":
|
||||
"time_mix_w2",
|
||||
"time_decay_w1",
|
||||
"time_decay_w2",
|
||||
"time_state",
|
||||
"lora.0",
|
||||
],
|
||||
)
|
||||
print(f"Saved to {args.output}")
|
||||
|
@ -1,3 +1,8 @@
|
||||
import setuptools
|
||||
|
||||
if setuptools.__version__ >= "70.0.0":
|
||||
raise ImportError("setuptools>=70.0.0 is not supported")
|
||||
|
||||
import multipart
|
||||
import fitz
|
||||
import safetensors
|
||||
@ -7,7 +12,6 @@ import lm_dataformat
|
||||
import ftfy
|
||||
import tqdm
|
||||
import tiktoken
|
||||
import GPUtil
|
||||
|
||||
import torch
|
||||
import rwkv
|
||||
|
@ -5,6 +5,7 @@ Model = "model"
|
||||
Model_Status = "model_status"
|
||||
Model_Config = "model_config"
|
||||
Deploy_Mode = "deploy_mode"
|
||||
Midi_Vocab_Config_Type = "midi_vocab_config_type"
|
||||
|
||||
|
||||
class ModelStatus(Enum):
|
||||
@ -13,11 +14,17 @@ class ModelStatus(Enum):
|
||||
Working = 3
|
||||
|
||||
|
||||
class MidiVocabConfig(Enum):
|
||||
Default = auto()
|
||||
Piano = auto()
|
||||
|
||||
|
||||
def init():
|
||||
global GLOBALS
|
||||
GLOBALS = {}
|
||||
set(Model_Status, ModelStatus.Offline)
|
||||
set(Deploy_Mode, False)
|
||||
set(Midi_Vocab_Config_Type, MidiVocabConfig.Default)
|
||||
|
||||
|
||||
def set(key, value):
|
||||
|
@ -27,11 +27,6 @@ def get_args(args: Union[Sequence[str], None] = None):
|
||||
action="store_true",
|
||||
help="whether to enable WebUI (default: False)",
|
||||
)
|
||||
group.add_argument(
|
||||
"--rwkv-beta",
|
||||
action="store_true",
|
||||
help="whether to use rwkv-beta (default: False)",
|
||||
)
|
||||
group.add_argument(
|
||||
"--rwkv.cpp",
|
||||
action="store_true",
|
||||
|
@ -1,9 +1,10 @@
|
||||
torch
|
||||
torchvision
|
||||
torchaudio
|
||||
rwkv==0.8.22
|
||||
setuptools==69.5.1
|
||||
rwkv==0.8.26
|
||||
langchain==0.0.322
|
||||
fastapi==0.104.0
|
||||
fastapi==0.109.1
|
||||
uvicorn==0.23.2
|
||||
sse-starlette==1.6.5
|
||||
pydantic==2.4.2
|
||||
@ -19,7 +20,7 @@ midi2audio==0.1.1
|
||||
mido==1.3.0
|
||||
safetensors==0.4.0
|
||||
PyMuPDF==1.23.5
|
||||
python-multipart==0.0.6
|
||||
python-multipart==0.0.7
|
||||
Cython==3.0.4
|
||||
cyac==1.9
|
||||
torch_directml==0.1.13.1.dev230413
|
||||
torch-directml==0.1.13.1.dev230413
|
||||
|
@ -1,9 +1,10 @@
|
||||
torch
|
||||
torchvision
|
||||
torchaudio
|
||||
rwkv==0.8.22
|
||||
setuptools==69.5.1
|
||||
rwkv==0.8.26
|
||||
langchain==0.0.322
|
||||
fastapi==0.104.0
|
||||
fastapi==0.109.1
|
||||
uvicorn==0.23.2
|
||||
sse-starlette==1.6.5
|
||||
pydantic==2.4.2
|
||||
@ -19,5 +20,5 @@ midi2audio==0.1.1
|
||||
mido==1.3.0
|
||||
safetensors==0.4.0
|
||||
PyMuPDF==1.23.5
|
||||
python-multipart==0.0.6
|
||||
python-multipart==0.0.7
|
||||
Cython==3.0.4
|
||||
|
@ -4,6 +4,7 @@ from threading import Lock
|
||||
from typing import List, Union
|
||||
from enum import Enum
|
||||
import base64
|
||||
import time
|
||||
|
||||
from fastapi import APIRouter, Request, status, HTTPException
|
||||
from sse_starlette.sse import EventSourceResponse
|
||||
@ -53,8 +54,11 @@ class ChatCompletionBody(ModelConfigBody):
|
||||
assistant_name: Union[str, None] = Field(
|
||||
None, description="Internal assistant name", min_length=1
|
||||
)
|
||||
system_name: Union[str, None] = Field(
|
||||
None, description="Internal system name", min_length=1
|
||||
)
|
||||
presystem: bool = Field(
|
||||
True, description="Whether to insert default system prompt at the beginning"
|
||||
False, description="Whether to insert default system prompt at the beginning"
|
||||
)
|
||||
|
||||
model_config = {
|
||||
@ -68,12 +72,13 @@ class ChatCompletionBody(ModelConfigBody):
|
||||
"stop": None,
|
||||
"user_name": None,
|
||||
"assistant_name": None,
|
||||
"system_name": None,
|
||||
"presystem": True,
|
||||
"max_tokens": 1000,
|
||||
"temperature": 1.2,
|
||||
"top_p": 0.5,
|
||||
"presence_penalty": 0.4,
|
||||
"frequency_penalty": 0.4,
|
||||
"temperature": 1,
|
||||
"top_p": 0.3,
|
||||
"presence_penalty": 0,
|
||||
"frequency_penalty": 1,
|
||||
}
|
||||
}
|
||||
}
|
||||
@ -94,10 +99,10 @@ class CompletionBody(ModelConfigBody):
|
||||
"stream": False,
|
||||
"stop": None,
|
||||
"max_tokens": 100,
|
||||
"temperature": 1.2,
|
||||
"top_p": 0.5,
|
||||
"presence_penalty": 0.4,
|
||||
"frequency_penalty": 0.4,
|
||||
"temperature": 1,
|
||||
"top_p": 0.3,
|
||||
"presence_penalty": 0,
|
||||
"frequency_penalty": 1,
|
||||
}
|
||||
}
|
||||
}
|
||||
@ -144,39 +149,57 @@ async def eval_rwkv(
|
||||
return
|
||||
set_rwkv_config(model, global_var.get(global_var.Model_Config))
|
||||
set_rwkv_config(model, body)
|
||||
print(get_rwkv_config(model))
|
||||
|
||||
response, prompt_tokens, completion_tokens = "", 0, 0
|
||||
completion_start_time = None
|
||||
for response, delta, prompt_tokens, completion_tokens in model.generate(
|
||||
prompt,
|
||||
stop=stop,
|
||||
):
|
||||
if not completion_start_time:
|
||||
completion_start_time = time.time()
|
||||
if await request.is_disconnected():
|
||||
break
|
||||
if stream:
|
||||
yield json.dumps(
|
||||
{
|
||||
"object": "chat.completion.chunk"
|
||||
if chat_mode
|
||||
else "text_completion",
|
||||
"object": (
|
||||
"chat.completion.chunk"
|
||||
if chat_mode
|
||||
else "text_completion"
|
||||
),
|
||||
# "response": response,
|
||||
"model": model.name,
|
||||
"id": "chatcmpl-123",
|
||||
"system_fingerprint": "fp_44709d6fcb",
|
||||
"choices": [
|
||||
{
|
||||
"delta": {"content": delta},
|
||||
"index": 0,
|
||||
"finish_reason": None,
|
||||
}
|
||||
if chat_mode
|
||||
else {
|
||||
"text": delta,
|
||||
"index": 0,
|
||||
"finish_reason": None,
|
||||
}
|
||||
(
|
||||
{
|
||||
"delta": {"role":Role.Assistant.value,"content": delta},
|
||||
"index": 0,
|
||||
"finish_reason": None,
|
||||
"logprobs":None
|
||||
}
|
||||
if chat_mode
|
||||
else {
|
||||
"text": delta,
|
||||
"index": 0,
|
||||
"finish_reason": None,
|
||||
}
|
||||
)
|
||||
],
|
||||
}
|
||||
)
|
||||
# torch_gc()
|
||||
requests_num = requests_num - 1
|
||||
completion_end_time = time.time()
|
||||
completion_interval = completion_end_time - completion_start_time
|
||||
tps = 0
|
||||
if completion_interval > 0:
|
||||
tps = completion_tokens / completion_interval
|
||||
print(f"Generation TPS: {tps:.2f}")
|
||||
|
||||
if await request.is_disconnected():
|
||||
print(f"{request.client} Stop Waiting")
|
||||
quick_log(
|
||||
@ -193,23 +216,28 @@ async def eval_rwkv(
|
||||
if stream:
|
||||
yield json.dumps(
|
||||
{
|
||||
"object": "chat.completion.chunk"
|
||||
if chat_mode
|
||||
else "text_completion",
|
||||
"object": (
|
||||
"chat.completion.chunk" if chat_mode else "text_completion"
|
||||
),
|
||||
# "response": response,
|
||||
"model": model.name,
|
||||
"id": "chatcmpl-123",
|
||||
"system_fingerprint": "fp_44709d6fcb",
|
||||
"choices": [
|
||||
{
|
||||
"delta": {},
|
||||
"index": 0,
|
||||
"finish_reason": "stop",
|
||||
}
|
||||
if chat_mode
|
||||
else {
|
||||
"text": "",
|
||||
"index": 0,
|
||||
"finish_reason": "stop",
|
||||
}
|
||||
(
|
||||
{
|
||||
"delta": {},
|
||||
"index": 0,
|
||||
"logprobs": None,
|
||||
"finish_reason": "stop",
|
||||
}
|
||||
if chat_mode
|
||||
else {
|
||||
"text": "",
|
||||
"index": 0,
|
||||
"finish_reason": "stop",
|
||||
}
|
||||
)
|
||||
],
|
||||
}
|
||||
)
|
||||
@ -225,38 +253,29 @@ async def eval_rwkv(
|
||||
"total_tokens": prompt_tokens + completion_tokens,
|
||||
},
|
||||
"choices": [
|
||||
{
|
||||
"message": {
|
||||
"role": Role.Assistant.value,
|
||||
"content": response,
|
||||
},
|
||||
"index": 0,
|
||||
"finish_reason": "stop",
|
||||
}
|
||||
if chat_mode
|
||||
else {
|
||||
"text": response,
|
||||
"index": 0,
|
||||
"finish_reason": "stop",
|
||||
}
|
||||
(
|
||||
{
|
||||
"message": {
|
||||
"role": Role.Assistant.value,
|
||||
"content": response,
|
||||
},
|
||||
"index": 0,
|
||||
"finish_reason": "stop",
|
||||
}
|
||||
if chat_mode
|
||||
else {
|
||||
"text": response,
|
||||
"index": 0,
|
||||
"finish_reason": "stop",
|
||||
}
|
||||
)
|
||||
],
|
||||
}
|
||||
|
||||
|
||||
@router.post("/v1/chat/completions", tags=["Completions"])
|
||||
@router.post("/chat/completions", tags=["Completions"])
|
||||
async def chat_completions(body: ChatCompletionBody, request: Request):
|
||||
model: TextRWKV = global_var.get(global_var.Model)
|
||||
if model is None:
|
||||
raise HTTPException(status.HTTP_400_BAD_REQUEST, "model not loaded")
|
||||
|
||||
if body.messages is None or body.messages == []:
|
||||
raise HTTPException(status.HTTP_400_BAD_REQUEST, "messages not found")
|
||||
|
||||
interface = model.interface
|
||||
user = model.user if body.user_name is None else body.user_name
|
||||
bot = model.bot if body.assistant_name is None else body.assistant_name
|
||||
|
||||
def chat_template_old(
|
||||
model: TextRWKV, body: ChatCompletionBody, interface: str, user: str, bot: str
|
||||
):
|
||||
is_raven = model.rwkv_type == RWKVType.Raven
|
||||
|
||||
completion_text: str = ""
|
||||
@ -325,6 +344,53 @@ The following is a coherent verbose detailed conversation between a girl named {
|
||||
completion_text += append_message + "\n\n"
|
||||
completion_text += f"{bot}{interface}"
|
||||
|
||||
return completion_text
|
||||
|
||||
|
||||
def chat_template(
|
||||
model: TextRWKV, body: ChatCompletionBody, interface: str, user: str, bot: str
|
||||
):
|
||||
completion_text: str = ""
|
||||
if body.presystem:
|
||||
completion_text = (
|
||||
f"{user}{interface} hi\n\n{bot}{interface} Hi. "
|
||||
+ "I am your assistant and I will provide expert full response in full details. Please feel free to ask any question and I will always answer it.\n\n"
|
||||
)
|
||||
|
||||
system = "System" if body.system_name is None else body.system_name
|
||||
for message in body.messages:
|
||||
append_message: str = ""
|
||||
if message.role == Role.User:
|
||||
append_message = f"{user}{interface} " + message.content
|
||||
elif message.role == Role.Assistant:
|
||||
append_message = f"{bot}{interface} " + message.content
|
||||
elif message.role == Role.System:
|
||||
append_message = f"{system}{interface} " + message.content
|
||||
completion_text += append_message + "\n\n"
|
||||
completion_text += f"{bot}{interface}"
|
||||
|
||||
return completion_text
|
||||
|
||||
|
||||
@router.post("/v1/chat/completions", tags=["Completions"])
|
||||
@router.post("/chat/completions", tags=["Completions"])
|
||||
async def chat_completions(body: ChatCompletionBody, request: Request):
|
||||
model: TextRWKV = global_var.get(global_var.Model)
|
||||
if model is None:
|
||||
raise HTTPException(status.HTTP_400_BAD_REQUEST, "model not loaded")
|
||||
|
||||
if body.messages is None or body.messages == []:
|
||||
raise HTTPException(status.HTTP_400_BAD_REQUEST, "messages not found")
|
||||
|
||||
interface = model.interface
|
||||
user = model.user if body.user_name is None else body.user_name
|
||||
bot = model.bot if body.assistant_name is None else body.assistant_name
|
||||
|
||||
if model.version < 5:
|
||||
completion_text = chat_template_old(model, body, interface, user, bot)
|
||||
else:
|
||||
completion_text = chat_template(model, body, interface, user, bot)
|
||||
|
||||
user_code = model.pipeline.decode([model.pipeline.encode(user)[0]])
|
||||
bot_code = model.pipeline.decode([model.pipeline.encode(bot)[0]])
|
||||
if type(body.stop) == str:
|
||||
@ -333,7 +399,9 @@ The following is a coherent verbose detailed conversation between a girl named {
|
||||
body.stop.append(f"\n\n{user_code}")
|
||||
body.stop.append(f"\n\n{bot_code}")
|
||||
elif body.stop is None:
|
||||
body.stop = default_stop
|
||||
body.stop = default_stop + [f"\n\n{user_code}", f"\n\n{bot_code}"]
|
||||
# if not body.presystem:
|
||||
# body.stop.append("\n\n")
|
||||
|
||||
if body.stream:
|
||||
return EventSourceResponse(
|
||||
|
@ -86,32 +86,58 @@ def switch_model(body: SwitchModelBody, response: Response, request: Request):
|
||||
|
||||
if body.deploy:
|
||||
global_var.set(global_var.Deploy_Mode, True)
|
||||
if global_var.get(global_var.Model_Config) is None:
|
||||
global_var.set(
|
||||
global_var.Model_Config, get_rwkv_config(global_var.get(global_var.Model))
|
||||
)
|
||||
|
||||
saved_model_config = global_var.get(global_var.Model_Config)
|
||||
init_model_config = get_rwkv_config(global_var.get(global_var.Model))
|
||||
if saved_model_config is not None:
|
||||
merge_model(init_model_config, saved_model_config)
|
||||
global_var.set(global_var.Model_Config, init_model_config)
|
||||
global_var.set(global_var.Model_Status, global_var.ModelStatus.Working)
|
||||
|
||||
return "success"
|
||||
|
||||
|
||||
def merge_model(to_model: BaseModel, from_model: BaseModel):
|
||||
from_model_fields = [x for x in from_model.dict().keys()]
|
||||
to_model_fields = [x for x in to_model.dict().keys()]
|
||||
|
||||
for field_name in from_model_fields:
|
||||
if field_name in to_model_fields:
|
||||
from_value = getattr(from_model, field_name)
|
||||
|
||||
if from_value is not None:
|
||||
setattr(to_model, field_name, from_value)
|
||||
|
||||
|
||||
@router.post("/update-config", tags=["Configs"])
|
||||
def update_config(body: ModelConfigBody):
|
||||
"""
|
||||
Will not update the model config immediately, but set it when completion called to avoid modifications during generation
|
||||
"""
|
||||
|
||||
print(body)
|
||||
global_var.set(global_var.Model_Config, body)
|
||||
model_config = global_var.get(global_var.Model_Config)
|
||||
if model_config is None:
|
||||
model_config = ModelConfigBody()
|
||||
global_var.set(global_var.Model_Config, model_config)
|
||||
merge_model(model_config, body)
|
||||
exception = load_rwkv_state(
|
||||
global_var.get(global_var.Model), model_config.state, True
|
||||
)
|
||||
if exception is not None:
|
||||
raise exception
|
||||
print("Updated Model Config:", model_config)
|
||||
|
||||
return "success"
|
||||
|
||||
|
||||
@router.get("/status", tags=["Configs"])
|
||||
def status():
|
||||
import GPUtil
|
||||
try:
|
||||
import GPUtil
|
||||
|
||||
gpus = GPUtil.getGPUs()
|
||||
gpus = GPUtil.getGPUs()
|
||||
except:
|
||||
gpus = []
|
||||
if len(gpus) == 0:
|
||||
device_name = "CPU"
|
||||
else:
|
||||
|
@ -23,7 +23,11 @@ class TextToMidiBody(BaseModel):
|
||||
|
||||
@router.post("/text-to-midi", tags=["MIDI"])
|
||||
def text_to_midi(body: TextToMidiBody):
|
||||
vocab_config = "backend-python/utils/midi_vocab_config.json"
|
||||
vocab_config_type = global_var.get(global_var.Midi_Vocab_Config_Type)
|
||||
if vocab_config_type == global_var.MidiVocabConfig.Piano:
|
||||
vocab_config = "backend-python/utils/vocab_config_piano.json"
|
||||
else:
|
||||
vocab_config = "backend-python/utils/midi_vocab_config.json"
|
||||
cfg = VocabConfig.from_json(vocab_config)
|
||||
mid = convert_str_to_midi(cfg, body.text.strip())
|
||||
mid_data = io.BytesIO()
|
||||
@ -35,7 +39,11 @@ def text_to_midi(body: TextToMidiBody):
|
||||
|
||||
@router.post("/midi-to-text", tags=["MIDI"])
|
||||
async def midi_to_text(file_data: UploadFile):
|
||||
vocab_config = "backend-python/utils/midi_vocab_config.json"
|
||||
vocab_config_type = global_var.get(global_var.Midi_Vocab_Config_Type)
|
||||
if vocab_config_type == global_var.MidiVocabConfig.Piano:
|
||||
vocab_config = "backend-python/utils/vocab_config_piano.json"
|
||||
else:
|
||||
vocab_config = "backend-python/utils/midi_vocab_config.json"
|
||||
cfg = VocabConfig.from_json(vocab_config)
|
||||
filter_config = "backend-python/utils/midi_filter_config.json"
|
||||
filter_cfg = FilterConfig.from_json(filter_config)
|
||||
@ -69,7 +77,11 @@ def txt_to_midi(body: TxtToMidiBody):
|
||||
if not body.midi_path.startswith("midi/"):
|
||||
raise HTTPException(status.HTTP_400_BAD_REQUEST, "bad output path")
|
||||
|
||||
vocab_config = "backend-python/utils/midi_vocab_config.json"
|
||||
vocab_config_type = global_var.get(global_var.Midi_Vocab_Config_Type)
|
||||
if vocab_config_type == global_var.MidiVocabConfig.Piano:
|
||||
vocab_config = "backend-python/utils/vocab_config_piano.json"
|
||||
else:
|
||||
vocab_config = "backend-python/utils/midi_vocab_config.json"
|
||||
cfg = VocabConfig.from_json(vocab_config)
|
||||
with open(body.txt_path, "r") as f:
|
||||
text = f.read()
|
||||
|
@ -76,6 +76,33 @@ class AddStateBody(BaseModel):
|
||||
logits: Any
|
||||
|
||||
|
||||
def copy_tensor_to_cpu(tensors):
|
||||
import torch
|
||||
import numpy as np
|
||||
|
||||
devices: List[torch.device] = []
|
||||
copied: Union[Any, None] = None
|
||||
|
||||
tensors_type = type(tensors)
|
||||
if tensors_type == list:
|
||||
if hasattr(tensors[0], "device"): # torch state
|
||||
devices = [tensor.device for tensor in tensors]
|
||||
copied = [tensor.cpu() for tensor in tensors]
|
||||
else: # WebGPU logits
|
||||
copied = tensors
|
||||
elif tensors_type == torch.Tensor: # torch logits
|
||||
devices = [tensors.device]
|
||||
copied = tensors.cpu()
|
||||
elif tensors_type == np.ndarray: # rwkv.cpp
|
||||
copied = tensors
|
||||
else: # WebGPU state
|
||||
model = global_var.get(global_var.Model)
|
||||
if model:
|
||||
copied = model.model.model.back_state()
|
||||
|
||||
return copied, devices
|
||||
|
||||
|
||||
# @router.post("/add-state", tags=["State Cache"])
|
||||
def add_state(body: AddStateBody):
|
||||
global trie, dtrie, loop_del_trie_id
|
||||
@ -91,32 +118,24 @@ def add_state(body: AddStateBody):
|
||||
|
||||
try:
|
||||
devices: List[torch.device] = []
|
||||
logits_device: Union[torch.device, None] = None
|
||||
state: Union[Any, None] = None
|
||||
logits: Union[Any, None] = None
|
||||
|
||||
if body.state is not None:
|
||||
if type(body.state) == list or type(body.state) == np.ndarray:
|
||||
devices = [
|
||||
(
|
||||
tensor.device
|
||||
if hasattr(tensor, "device")
|
||||
else torch.device("cpu")
|
||||
)
|
||||
for tensor in body.state
|
||||
]
|
||||
state = (
|
||||
[tensor.cpu() for tensor in body.state]
|
||||
if hasattr(body.state[0], "device")
|
||||
else copy.deepcopy(body.state)
|
||||
)
|
||||
else:
|
||||
pass # WebGPU
|
||||
state, devices = copy_tensor_to_cpu(body.state)
|
||||
if body.logits is not None:
|
||||
logits, logits_devices = copy_tensor_to_cpu(body.logits)
|
||||
if len(logits_devices) > 0:
|
||||
logits_device = logits_devices[0]
|
||||
|
||||
id: int = trie.insert(body.prompt)
|
||||
dtrie[id] = {
|
||||
"tokens": copy.deepcopy(body.tokens),
|
||||
"tokens": body.tokens,
|
||||
"state": state,
|
||||
"logits": copy.deepcopy(body.logits),
|
||||
"logits": logits,
|
||||
"devices": devices,
|
||||
"logits_device": logits_device,
|
||||
}
|
||||
|
||||
if len(trie) >= max_trie_len:
|
||||
@ -134,6 +153,7 @@ def add_state(body: AddStateBody):
|
||||
)
|
||||
return "success"
|
||||
except Exception as e:
|
||||
print(e) # should not happen
|
||||
raise HTTPException(
|
||||
status.HTTP_400_BAD_REQUEST, f"insert failed, bad prompt.\n{e}"
|
||||
)
|
||||
@ -158,6 +178,19 @@ def reset_state():
|
||||
return "success"
|
||||
|
||||
|
||||
def force_reset_state():
|
||||
global trie, dtrie
|
||||
|
||||
if trie is None:
|
||||
return
|
||||
|
||||
import cyac
|
||||
|
||||
trie = cyac.Trie()
|
||||
dtrie = {}
|
||||
gc.collect()
|
||||
|
||||
|
||||
class LongestPrefixStateBody(BaseModel):
|
||||
prompt: str
|
||||
|
||||
@ -199,20 +232,40 @@ def longest_prefix_state(body: LongestPrefixStateBody, request: Request):
|
||||
except:
|
||||
pass
|
||||
if id != -1:
|
||||
v = dtrie[id]
|
||||
devices: List[torch.device] = v["devices"]
|
||||
prompt: str = trie[id]
|
||||
v = dtrie[id]
|
||||
tokens: List[Union[str, int]] = copy.deepcopy(v["tokens"])
|
||||
devices: List[torch.device] = v["devices"]
|
||||
logits_device: Union[torch.device, None] = v["logits_device"]
|
||||
state: Union[Any, None] = v["state"]
|
||||
logits: Union[Any, None] = v["logits"]
|
||||
|
||||
if state is not None and type(state) == list and hasattr(state[0], "device"):
|
||||
state = [tensor.to(devices[i]) for i, tensor in enumerate(state)]
|
||||
state_type = type(state)
|
||||
if state_type == list and hasattr(state[0], "device"): # torch
|
||||
state = [
|
||||
(
|
||||
tensor.to(devices[i])
|
||||
if devices[i] != torch.device("cpu")
|
||||
else tensor.clone()
|
||||
)
|
||||
for i, tensor in enumerate(state)
|
||||
]
|
||||
logits = (
|
||||
logits.to(logits_device)
|
||||
if logits_device != torch.device("cpu")
|
||||
else logits.clone()
|
||||
)
|
||||
elif state_type == np.ndarray: # rwkv.cpp
|
||||
logits = np.copy(logits)
|
||||
else: # WebGPU
|
||||
logits = np.copy(logits)
|
||||
|
||||
quick_log(request, body, "Hit:\n" + prompt)
|
||||
return {
|
||||
"prompt": prompt,
|
||||
"tokens": v["tokens"],
|
||||
"tokens": tokens,
|
||||
"state": state,
|
||||
"logits": v["logits"],
|
||||
"logits": logits,
|
||||
}
|
||||
else:
|
||||
return {"prompt": "", "tokens": [], "state": None, "logits": None}
|
||||
|
124
backend-python/rwkv_pip/beta/cuda/att_one.cu
vendored
124
backend-python/rwkv_pip/beta/cuda/att_one.cu
vendored
@ -1,124 +0,0 @@
|
||||
#include "ATen/ATen.h"
|
||||
#include <cuda_fp16.h>
|
||||
#include <cuda_runtime.h>
|
||||
#include <torch/extension.h>
|
||||
|
||||
#include "element_wise.h"
|
||||
#include "util.h"
|
||||
|
||||
// Equivalent Python code:
|
||||
// ww = t_first + k
|
||||
// p = torch.maximum(pp, ww)
|
||||
// e1 = torch.exp(pp - p)
|
||||
// e2 = torch.exp(ww - p)
|
||||
// wkv = ((e1 * aa + e2 * v) / (e1 * bb + e2)).to(dtype=x.dtype)
|
||||
// ww = t_decay + pp
|
||||
// p = torch.maximum(ww, k)
|
||||
// e1 = torch.exp(ww - p)
|
||||
// e2 = torch.exp(k - p)
|
||||
// t1 = e1 * aa + e2 * v
|
||||
// t2 = e1 * bb + e2
|
||||
// r = r * wkv
|
||||
// return t1, t2, p, r
|
||||
struct WkvForwardOne {
|
||||
const float *t_first;
|
||||
const float *k;
|
||||
const float *pp;
|
||||
const float *aa;
|
||||
const float *bb;
|
||||
const float *t_decay;
|
||||
const float *v;
|
||||
/* out */ float *t1;
|
||||
/* out */ float *t2;
|
||||
/* out */ float *p;
|
||||
/* in & out */ half *r;
|
||||
|
||||
__device__ void operator()(int i) const {
|
||||
float ww = t_first[i] + k[i];
|
||||
float pp_ = pp[i];
|
||||
float p_ = (pp_ > ww) ? pp_ : ww;
|
||||
float e1 = expf(pp_ - p_);
|
||||
float e2 = expf(ww - p_);
|
||||
float aa_ = aa[i];
|
||||
float bb_ = bb[i];
|
||||
float v_ = v[i];
|
||||
r[i] = __hmul(r[i], __float2half(((e1 * aa_ + e2 * v_) / (e1 * bb_ + e2))));
|
||||
ww = t_decay[i] + pp_;
|
||||
float k_ = k[i];
|
||||
p_ = (ww > k_) ? ww : k_;
|
||||
e1 = expf(ww - p_);
|
||||
e2 = expf(k_ - p_);
|
||||
t1[i] = e1 * aa_ + e2 * v_;
|
||||
t2[i] = e1 * bb_ + e2;
|
||||
p[i] = p_;
|
||||
}
|
||||
};
|
||||
|
||||
/*
|
||||
Equivalent Python code:
|
||||
kx = xx * k_mix + sx * (1 - k_mix)
|
||||
vx = xx * v_mix + sx * (1 - v_mix)
|
||||
rx = xx * r_mix + sx * (1 - r_mix)
|
||||
*/
|
||||
|
||||
struct Mix {
|
||||
const half *xx;
|
||||
const half *sx;
|
||||
const half *k_mix;
|
||||
const half *v_mix;
|
||||
const half *r_mix;
|
||||
/* out */ half *kx;
|
||||
/* out */ half *vx;
|
||||
/* out */ half *rx;
|
||||
|
||||
__device__ void operator()(int i) const {
|
||||
half xx_ = xx[i];
|
||||
half sx_ = sx[i];
|
||||
half k_mix_ = k_mix[i];
|
||||
half v_mix_ = v_mix[i];
|
||||
half r_mix_ = r_mix[i];
|
||||
kx[i] = __hadd(__hmul(xx_, k_mix_),
|
||||
__hmul(sx_, __hsub(__float2half(1), k_mix_)));
|
||||
vx[i] = __hadd(__hmul(xx_, v_mix_),
|
||||
__hmul(sx_, __hsub(__float2half(1), v_mix_)));
|
||||
rx[i] = __hadd(__hmul(xx_, r_mix_),
|
||||
__hmul(sx_, __hsub(__float2half(1), r_mix_)));
|
||||
}
|
||||
};
|
||||
|
||||
using torch::Tensor;
|
||||
|
||||
void gemm_fp16_cublas_tensor(Tensor a, Tensor b, Tensor c);
|
||||
|
||||
Tensor att_one(Tensor x, Tensor ln_w, Tensor ln_b, Tensor sx, Tensor k_mix,
|
||||
Tensor v_mix, Tensor r_mix, Tensor kw,
|
||||
/* imm */ Tensor kx, Tensor vw, /* imm */ Tensor vx, Tensor rw,
|
||||
/* imm */ Tensor rx, Tensor ow, Tensor t_first,
|
||||
/* imm */ Tensor k, Tensor pp, Tensor ww, Tensor aa, Tensor bb,
|
||||
Tensor t_decay, /* imm */ Tensor v, /* in & out */ Tensor r,
|
||||
/* out */ Tensor x_plus_out, /* out */ Tensor t1,
|
||||
/* out */ Tensor t2, /* out */ Tensor p) {
|
||||
Tensor xx = at::layer_norm(x, {x.size(-1)}, ln_w, ln_b);
|
||||
element_wise(Mix{data_ptr<half>(xx), data_ptr<half>(sx),
|
||||
data_ptr<half>(k_mix), data_ptr<half>(v_mix),
|
||||
data_ptr<half>(r_mix), data_ptr<half>(kx),
|
||||
data_ptr<half>(vx), data_ptr<half>(rx)},
|
||||
x.numel());
|
||||
|
||||
gemm_fp16_cublas_tensor(kx, kw, k);
|
||||
gemm_fp16_cublas_tensor(vx, vw, v);
|
||||
gemm_fp16_cublas_tensor(rx, rw, r);
|
||||
at::sigmoid_(r);
|
||||
|
||||
element_wise(WkvForwardOne{data_ptr<float>(t_first), data_ptr<float>(k),
|
||||
data_ptr<float>(pp), data_ptr<float>(aa),
|
||||
data_ptr<float>(bb), data_ptr<float>(t_decay),
|
||||
data_ptr<float>(v), data_ptr<float>(t1),
|
||||
data_ptr<float>(t2), data_ptr<float>(p),
|
||||
data_ptr<half>(r)},
|
||||
x.numel());
|
||||
|
||||
gemm_fp16_cublas_tensor(r, ow, x_plus_out);
|
||||
x_plus_out += x;
|
||||
return xx;
|
||||
}
|
109
backend-python/rwkv_pip/beta/cuda/att_one_v5.cu
vendored
109
backend-python/rwkv_pip/beta/cuda/att_one_v5.cu
vendored
@ -1,109 +0,0 @@
|
||||
#include "ATen/ATen.h"
|
||||
#include <cuda_fp16.h>
|
||||
#include <cuda_runtime.h>
|
||||
#include <torch/extension.h>
|
||||
|
||||
#include "element_wise.h"
|
||||
#include "util.h"
|
||||
|
||||
// Equivalent Python code:
|
||||
// s1 = t_first * a + s
|
||||
// s2 = a + t_decay * s
|
||||
struct Fused1 {
|
||||
const float *t_first;
|
||||
const float *t_decay;
|
||||
const float *a;
|
||||
const float *s;
|
||||
const int32_t inner_size;
|
||||
/* out */ float *s1;
|
||||
/* out */ float *s2;
|
||||
|
||||
__device__ void operator()(int i) const {
|
||||
const int j = i / inner_size;
|
||||
s1[i] = t_first[j] * a[i] + s[i];
|
||||
s2[i] = a[i] + t_decay[j] * s[i];
|
||||
}
|
||||
};
|
||||
|
||||
/*
|
||||
Equivalent Python code:
|
||||
kx = xx * k_mix + sx * (1 - k_mix)
|
||||
vx = xx * v_mix + sx * (1 - v_mix)
|
||||
rx = xx * r_mix + sx * (1 - r_mix)
|
||||
*/
|
||||
|
||||
struct Mix {
|
||||
const half *xx;
|
||||
const half *sx;
|
||||
const half *k_mix;
|
||||
const half *v_mix;
|
||||
const half *r_mix;
|
||||
/* out */ half *kx;
|
||||
/* out */ half *vx;
|
||||
/* out */ half *rx;
|
||||
|
||||
__device__ void operator()(int i) const {
|
||||
half xx_ = xx[i];
|
||||
half sx_ = sx[i];
|
||||
half k_mix_ = k_mix[i];
|
||||
half v_mix_ = v_mix[i];
|
||||
half r_mix_ = r_mix[i];
|
||||
kx[i] = __hadd(__hmul(xx_, k_mix_),
|
||||
__hmul(sx_, __hsub(__float2half(1), k_mix_)));
|
||||
vx[i] = __hadd(__hmul(xx_, v_mix_),
|
||||
__hmul(sx_, __hsub(__float2half(1), v_mix_)));
|
||||
rx[i] = __hadd(__hmul(xx_, r_mix_),
|
||||
__hmul(sx_, __hsub(__float2half(1), r_mix_)));
|
||||
}
|
||||
};
|
||||
|
||||
using torch::Tensor;
|
||||
|
||||
void gemm_fp16_cublas_tensor(Tensor a, Tensor b, Tensor c);
|
||||
|
||||
Tensor att_one_v5(Tensor x, Tensor sx, Tensor s, Tensor ln_w, Tensor ln_b,
|
||||
Tensor lx_w, Tensor lx_b, Tensor k_mix, Tensor v_mix,
|
||||
Tensor r_mix, Tensor kw,
|
||||
/* imm */ Tensor kx, Tensor vw, /* imm */ Tensor vx,
|
||||
Tensor rw,
|
||||
/* imm */ Tensor rx, Tensor ow, Tensor t_first,
|
||||
/* imm */ Tensor k, Tensor t_decay, /* imm */ Tensor v,
|
||||
/* imm */ Tensor r, /* imm */ Tensor s1,
|
||||
/* out */ Tensor x_plus_out, /* out */ Tensor s2) {
|
||||
Tensor xx = at::layer_norm(x, {x.size(-1)}, ln_w, ln_b);
|
||||
element_wise(Mix{data_ptr<half>(xx), data_ptr<half>(sx),
|
||||
data_ptr<half>(k_mix), data_ptr<half>(v_mix),
|
||||
data_ptr<half>(r_mix), data_ptr<half>(kx),
|
||||
data_ptr<half>(vx), data_ptr<half>(rx)},
|
||||
x.numel());
|
||||
|
||||
int H = t_decay.size(0);
|
||||
int S = x.size(-1) / H;
|
||||
gemm_fp16_cublas_tensor(rx, rw, r);
|
||||
r = at::reshape(r, {H, 1, S});
|
||||
gemm_fp16_cublas_tensor(kx, kw, k);
|
||||
k = at::reshape(k, {H, S, 1});
|
||||
gemm_fp16_cublas_tensor(vx, vw, v);
|
||||
v = at::reshape(v, {H, 1, S});
|
||||
|
||||
{
|
||||
Tensor a = at::matmul(k, v);
|
||||
|
||||
// s1 = t_first * a + s
|
||||
// s2 = a + t_decay * s
|
||||
element_wise(Fused1{data_ptr<float>(t_first), data_ptr<float>(t_decay),
|
||||
data_ptr<float>(a), data_ptr<float>(s),
|
||||
static_cast<int32_t>(a.size(1) * a.size(2)),
|
||||
data_ptr<float>(s1), data_ptr<float>(s2)},
|
||||
a.numel());
|
||||
}
|
||||
|
||||
Tensor out = at::matmul(r, s1);
|
||||
out = at::flatten(out);
|
||||
out = at::squeeze(at::group_norm(at::unsqueeze(out, 0), H, lx_w, lx_b), 0);
|
||||
out = at::_cast_Half(out);
|
||||
|
||||
gemm_fp16_cublas_tensor(out, ow, x_plus_out);
|
||||
x_plus_out += x;
|
||||
return xx;
|
||||
}
|
178
backend-python/rwkv_pip/beta/cuda/att_seq.cu
vendored
178
backend-python/rwkv_pip/beta/cuda/att_seq.cu
vendored
@ -1,178 +0,0 @@
|
||||
#include "ATen/ATen.h"
|
||||
#include <cuda_fp16.h>
|
||||
#include <cuda_runtime.h>
|
||||
#include <torch/extension.h>
|
||||
|
||||
#include "util.h"
|
||||
#include "element_wise.h"
|
||||
|
||||
using torch::Tensor;
|
||||
|
||||
void gemm_fp16_cublas(const void *a, const void *b, void *c, int m,
|
||||
int n, int k, bool output_fp32);
|
||||
|
||||
// based on `kernel_wkv_forward`, fusing more operations
|
||||
__global__ void kernel_wkv_forward_new(
|
||||
const int B, const int T, const int C, const float *__restrict__ const _w,
|
||||
const float *__restrict__ const _u, const float *__restrict__ const _k,
|
||||
const float *__restrict__ const _v, const half *__restrict__ const r,
|
||||
half *__restrict__ const _y, float *__restrict__ const _aa,
|
||||
float *__restrict__ const _bb, float *__restrict__ const _pp) {
|
||||
const int idx = blockIdx.x * blockDim.x + threadIdx.x;
|
||||
const int _b = idx / C;
|
||||
const int _c = idx % C;
|
||||
const int _offset = _b * T * C + _c;
|
||||
const int _state_offset = _b * C + _c;
|
||||
|
||||
float u = _u[_c];
|
||||
float w = _w[_c];
|
||||
const float *__restrict__ const k = _k + _offset;
|
||||
const float *__restrict__ const v = _v + _offset;
|
||||
half *__restrict__ const y = _y + _offset;
|
||||
|
||||
float aa = _aa[_state_offset];
|
||||
float bb = _bb[_state_offset];
|
||||
float pp = _pp[_state_offset];
|
||||
for (int i = 0; i < T; i++) {
|
||||
const int ii = i * C;
|
||||
const float kk = k[ii];
|
||||
const float vv = v[ii];
|
||||
float ww = u + kk;
|
||||
float p = max(pp, ww);
|
||||
float e1 = exp(pp - p);
|
||||
float e2 = exp(ww - p);
|
||||
y[ii] = __float2half((e1 * aa + e2 * vv) / (e1 * bb + e2));
|
||||
ww = w + pp;
|
||||
p = max(ww, kk);
|
||||
e1 = exp(ww - p);
|
||||
e2 = exp(kk - p);
|
||||
aa = e1 * aa + e2 * vv;
|
||||
bb = e1 * bb + e2;
|
||||
pp = p;
|
||||
}
|
||||
_aa[_state_offset] = aa;
|
||||
_bb[_state_offset] = bb;
|
||||
_pp[_state_offset] = pp;
|
||||
}
|
||||
|
||||
void cuda_wkv_forward_new(int B, int T, int C, float *w, float *u, float *k,
|
||||
float *v, half *r, half *y, float *aa, float *bb,
|
||||
float *pp) {
|
||||
dim3 threadsPerBlock(min(C, 32));
|
||||
assert(B * C % threadsPerBlock.x == 0);
|
||||
dim3 numBlocks(B * C / threadsPerBlock.x);
|
||||
kernel_wkv_forward_new<<<numBlocks, threadsPerBlock>>>(B, T, C, w, u, k, v, r,
|
||||
y, aa, bb, pp);
|
||||
}
|
||||
|
||||
__global__ void _att_mix(const half *xx, const half *sx, const half *k_mix,
|
||||
const half *v_mix, const half *r_mix,
|
||||
const int outer_size, const int inner_size, half *kx,
|
||||
half *vx, half *rx) {
|
||||
for (int idx2 = blockIdx.x * blockDim.x + threadIdx.x; idx2 < inner_size;
|
||||
idx2 += blockDim.x * gridDim.x) {
|
||||
half k_mix_ = k_mix[idx2];
|
||||
half v_mix_ = v_mix[idx2];
|
||||
half r_mix_ = r_mix[idx2];
|
||||
for (int row = 0; row < outer_size; ++row) {
|
||||
int idx1 = row * inner_size + idx2;
|
||||
half xx_ = xx[idx1];
|
||||
half sx_ = sx[idx1];
|
||||
kx[idx1] = __hadd(__hmul(xx_, k_mix_),
|
||||
__hmul(sx_, __hsub(__float2half(1), k_mix_)));
|
||||
vx[idx1] = __hadd(__hmul(xx_, v_mix_),
|
||||
__hmul(sx_, __hsub(__float2half(1), v_mix_)));
|
||||
rx[idx1] = __hadd(__hmul(xx_, r_mix_),
|
||||
__hmul(sx_, __hsub(__float2half(1), r_mix_)));
|
||||
}
|
||||
}
|
||||
}
|
||||
|
||||
void att_mix(const half *xx, const half *sx, const half *k_mix,
|
||||
const half *v_mix, const half *r_mix, const int outer_size,
|
||||
const int inner_size, half *kx, half *vx, half *rx) {
|
||||
// 256 is good enough on most GPUs
|
||||
const int32_t BLOCK_SIZE = 256;
|
||||
assert(inner_size % BLOCK_SIZE == 0);
|
||||
_att_mix<<<inner_size / BLOCK_SIZE, BLOCK_SIZE>>>(
|
||||
xx, sx, k_mix, v_mix, r_mix, outer_size, inner_size, kx, vx, rx);
|
||||
}
|
||||
|
||||
struct InplaceSigmoid {
|
||||
__device__ __forceinline__ half operator()(int i) const {
|
||||
ptr[i] = __float2half(1.0 / (1.0 + exp(-__half2float(ptr[i]))));
|
||||
}
|
||||
half *ptr;
|
||||
};
|
||||
|
||||
struct InplaceMul {
|
||||
__device__ __forceinline__ half operator()(int i) const {
|
||||
y[i] = __hmul(x[i], y[i]);
|
||||
}
|
||||
half *y;
|
||||
half *x;
|
||||
};
|
||||
|
||||
/*
|
||||
Equivalent Python code:
|
||||
|
||||
xx = F.layer_norm(x, (x.shape[-1],), weight=ln_w, bias=ln_b)
|
||||
sx = torch.cat((sx.unsqueeze(0), xx[:-1,:]))
|
||||
kx = xx * k_mix + sx * (1 - k_mix)
|
||||
vx = xx * v_mix + sx * (1 - v_mix)
|
||||
rx = xx * r_mix + sx * (1 - r_mix)
|
||||
|
||||
r = torch.sigmoid(gemm(rx, rw))
|
||||
k = gemm(kx, kw, output_dtype=torch.float32)
|
||||
v = gemm(vx, vw, output_dtype=torch.float32)
|
||||
|
||||
T = x.shape[0]
|
||||
for t in range(T):
|
||||
kk = k[t]
|
||||
vv = v[t]
|
||||
ww = t_first + kk
|
||||
p = torch.maximum(pp, ww)
|
||||
e1 = torch.exp(pp - p)
|
||||
e2 = torch.exp(ww - p)
|
||||
sx[t] = ((e1 * aa + e2 * vv) / (e1 * bb + e2)).to(dtype=x.dtype)
|
||||
ww = t_decay + pp
|
||||
p = torch.maximum(ww, kk)
|
||||
e1 = torch.exp(ww - p)
|
||||
e2 = torch.exp(kk - p)
|
||||
aa = e1 * aa + e2 * vv
|
||||
bb = e1 * bb + e2
|
||||
pp = p
|
||||
out = gemm(r * sx, ow)
|
||||
return x + out, xx[-1,:], aa, bb, pp
|
||||
*/
|
||||
Tensor att_seq(Tensor x, Tensor sx, Tensor ln_w, Tensor ln_b, Tensor k_mix,
|
||||
Tensor v_mix, Tensor r_mix, Tensor kw, Tensor vw, Tensor rw,
|
||||
Tensor ow, Tensor t_first, Tensor pp, Tensor aa, Tensor bb,
|
||||
Tensor t_decay, /* imm */ Tensor buf, /* out */ Tensor x_plus_out) {
|
||||
Tensor xx = at::layer_norm(x, {x.size(-1)}, ln_w, ln_b);
|
||||
sx = at::cat({sx.unsqueeze(0), xx.slice(0, 0, -1)}, 0);
|
||||
char* buf_ptr = (char*)buf.data_ptr();
|
||||
half* kx = (half*)buf_ptr;
|
||||
half* vx = kx + x.numel();
|
||||
half* rx = vx + x.numel();
|
||||
half* wkv_y = rx + x.numel();
|
||||
att_mix(data_ptr<half>(xx), data_ptr<half>(sx), data_ptr<half>(k_mix),
|
||||
data_ptr<half>(v_mix), data_ptr<half>(r_mix), xx.size(0), xx.size(1),
|
||||
kx, vx, rx);
|
||||
float* k = reinterpret_cast<float*>(wkv_y + x.numel());
|
||||
float* v = k + x.size(0) * kw.size(1);
|
||||
half* r = reinterpret_cast<half*>(v + x.size(0) * vw.size(1));
|
||||
|
||||
gemm_fp16_cublas(kx, kw.data_ptr(), k, x.size(0), kw.size(1), kw.size(0), true);
|
||||
gemm_fp16_cublas(vx, vw.data_ptr(), v, x.size(0), vw.size(1), vw.size(0), true);
|
||||
gemm_fp16_cublas(rx, rw.data_ptr(), r, x.size(0), rw.size(1), rw.size(0), false);
|
||||
element_wise(InplaceSigmoid{r}, x.size(0) * rw.size(1));
|
||||
cuda_wkv_forward_new(1, x.size(0), x.size(1), data_ptr<float>(t_decay),
|
||||
data_ptr<float>(t_first), k, v, r,
|
||||
wkv_y, data_ptr<float>(aa),
|
||||
data_ptr<float>(bb), data_ptr<float>(pp));
|
||||
element_wise(InplaceMul{wkv_y, r}, x.numel());
|
||||
gemm_fp16_cublas(wkv_y, ow.data_ptr(), x_plus_out.data_ptr(), x.size(0), ow.size(1), ow.size(0), false);
|
||||
x_plus_out += x;
|
||||
return xx;
|
||||
}
|
21
backend-python/rwkv_pip/beta/cuda/element_wise.h
vendored
21
backend-python/rwkv_pip/beta/cuda/element_wise.h
vendored
@ -1,21 +0,0 @@
|
||||
#include <cassert>
|
||||
#include <cstddef>
|
||||
#include <cstdint>
|
||||
|
||||
template <typename Func> __global__ void _element_wise(Func func, int n) {
|
||||
for (int i = blockIdx.x * blockDim.x + threadIdx.x; i < n;
|
||||
i += blockDim.x * gridDim.x) {
|
||||
func(i);
|
||||
}
|
||||
}
|
||||
|
||||
// NOTE: packed data type (e.g. float4) is a overkill for current sizes
|
||||
// (4096 in 7B model and 768 in 0.1B model),
|
||||
// and is not faster than the plain float version.
|
||||
template <typename Func>
|
||||
void element_wise(Func func, int n) {
|
||||
// 256 is good enough on most GPUs
|
||||
const int32_t BLOCK_SIZE = 256;
|
||||
assert(n % BLOCK_SIZE == 0);
|
||||
_element_wise<<<n / BLOCK_SIZE, BLOCK_SIZE>>>(func, n);
|
||||
}
|
165
backend-python/rwkv_pip/beta/cuda/ffn.cu
vendored
165
backend-python/rwkv_pip/beta/cuda/ffn.cu
vendored
@ -1,165 +0,0 @@
|
||||
#include "ATen/ATen.h"
|
||||
#include <cuda_fp16.h>
|
||||
#include <cuda_runtime.h>
|
||||
#include <torch/extension.h>
|
||||
|
||||
#include "element_wise.h"
|
||||
#include "util.h"
|
||||
|
||||
using torch::Tensor;
|
||||
|
||||
void gemm_fp16_cublas(const void *a, const void *b, void *c, int ori_m,
|
||||
int ori_n, int ori_k, bool output_fp32);
|
||||
|
||||
__global__ void _ffn_seq_mix(const half *xx, const half *sx, const half *k_mix,
|
||||
const half *r_mix, const int outer_size,
|
||||
const int inner_size, half *kx, half *rx) {
|
||||
for (int idx2 = blockIdx.x * blockDim.x + threadIdx.x; idx2 < inner_size;
|
||||
idx2 += blockDim.x * gridDim.x) {
|
||||
half k_mix_ = k_mix[idx2];
|
||||
half r_mix_ = r_mix[idx2];
|
||||
for (int row = 0; row < outer_size; ++row) {
|
||||
int idx1 = row * inner_size + idx2;
|
||||
half xx_ = xx[idx1];
|
||||
half sx_ = sx[idx1];
|
||||
kx[idx1] = __hadd(__hmul(xx_, k_mix_),
|
||||
__hmul(sx_, __hsub(__float2half(1), k_mix_)));
|
||||
rx[idx1] = __hadd(__hmul(xx_, r_mix_),
|
||||
__hmul(sx_, __hsub(__float2half(1), r_mix_)));
|
||||
}
|
||||
}
|
||||
}
|
||||
|
||||
void ffn_seq_mix(const half *xx, const half *sx, const half *k_mix,
|
||||
const half *r_mix, const int outer_size, const int inner_size,
|
||||
half *kx, half *rx) {
|
||||
// 256 is good enough on most GPUs
|
||||
const int32_t BLOCK_SIZE = 256;
|
||||
assert(inner_size % BLOCK_SIZE == 0);
|
||||
_ffn_seq_mix<<<inner_size / BLOCK_SIZE, BLOCK_SIZE>>>(
|
||||
xx, sx, k_mix, r_mix, outer_size, inner_size, kx, rx);
|
||||
}
|
||||
|
||||
struct InplaceSigmoid {
|
||||
__device__ __forceinline__ void operator()(int i) const {
|
||||
ptr[i] = __float2half(1.0 / (1.0 + exp(-__half2float(ptr[i]))));
|
||||
}
|
||||
half *ptr;
|
||||
};
|
||||
|
||||
struct InplaceReLUAndSquare {
|
||||
__device__ __forceinline__ void operator()(int i) const {
|
||||
// __hmax is not defined in old cuda
|
||||
if (__hgt(ptr[i], __float2half(0))) {
|
||||
ptr[i] = __hmul(ptr[i], ptr[i]);
|
||||
} else {
|
||||
ptr[i] = __float2half(0);
|
||||
}
|
||||
}
|
||||
half *ptr;
|
||||
};
|
||||
|
||||
struct InplaceFma {
|
||||
__device__ __forceinline__ void operator()(int i) const {
|
||||
a[i] = __hfma(a[i], b[i], c[i]);
|
||||
}
|
||||
half *a;
|
||||
const half *b;
|
||||
const half *c;
|
||||
};
|
||||
|
||||
/*
|
||||
Equivalent Python code:
|
||||
|
||||
xx = F.layer_norm(x, (x.shape[-1],), weight=ln_w, bias=ln_b)
|
||||
sx = torch.cat((sx.unsqueeze(0), xx[:-1,:]))
|
||||
kx = xx * k_mix + sx * (1 - k_mix)
|
||||
rx = xx * r_mix + sx * (1 - r_mix)
|
||||
|
||||
r = torch.sigmoid(gemm(rx, rw))
|
||||
vx = torch.square(torch.relu(gemm(kx, kw)))
|
||||
out = r * gemm(vx, vw)
|
||||
return x + out, xx[-1,:]
|
||||
*/
|
||||
Tensor ffn_seq(Tensor x, Tensor sx, Tensor ln_w, Tensor ln_b, Tensor k_mix,
|
||||
Tensor r_mix, Tensor kw, Tensor vw, Tensor rw,
|
||||
/* imm */ Tensor buf,
|
||||
/* out */ Tensor x_plus_out) {
|
||||
Tensor xx = at::layer_norm(x, {x.size(-1)}, ln_w, ln_b);
|
||||
sx = at::cat({sx.unsqueeze(0), xx.slice(0, 0, -1)}, 0);
|
||||
char *buf_ptr = (char *)buf.data_ptr();
|
||||
half *kx = (half *)buf_ptr;
|
||||
half *rx = kx + x.numel();
|
||||
half *vx = rx + x.numel();
|
||||
half *r = vx + x.size(0) * kw.size(1);
|
||||
ffn_seq_mix(data_ptr<half>(xx), data_ptr<half>(sx), data_ptr<half>(k_mix),
|
||||
data_ptr<half>(r_mix), xx.size(0), xx.size(1), kx, rx);
|
||||
|
||||
gemm_fp16_cublas(rx, rw.data_ptr(), r, x.size(0), rw.size(1), x.size(1),
|
||||
false);
|
||||
element_wise(InplaceSigmoid{r}, x.size(0) * rw.size(1));
|
||||
gemm_fp16_cublas(kx, kw.data_ptr(), vx, x.size(0), kw.size(1), x.size(1),
|
||||
false);
|
||||
element_wise(InplaceReLUAndSquare{vx}, x.size(0) * kw.size(1));
|
||||
gemm_fp16_cublas(vx, vw.data_ptr(), x_plus_out.data_ptr(), x.size(0),
|
||||
vw.size(1), vw.size(0), false);
|
||||
element_wise(InplaceFma{data_ptr<half>(x_plus_out), r, data_ptr<half>(x)},
|
||||
x_plus_out.numel());
|
||||
return xx;
|
||||
}
|
||||
|
||||
struct FfnOneMix {
|
||||
__device__ __forceinline__ void operator()(int idx) {
|
||||
half k_mix_ = k_mix[idx];
|
||||
half r_mix_ = r_mix[idx];
|
||||
half xx_ = xx[idx];
|
||||
half sx_ = sx[idx];
|
||||
kx[idx] = __hadd(__hmul(xx_, k_mix_),
|
||||
__hmul(sx_, __hsub(__float2half(1), k_mix_)));
|
||||
rx[idx] = __hadd(__hmul(xx_, r_mix_),
|
||||
__hmul(sx_, __hsub(__float2half(1), r_mix_)));
|
||||
}
|
||||
half *k_mix;
|
||||
half *r_mix;
|
||||
half *xx;
|
||||
half *sx;
|
||||
half *kx;
|
||||
half *rx;
|
||||
};
|
||||
|
||||
/*
|
||||
Equivalent Python code:
|
||||
|
||||
xx = F.layer_norm(x, (x.shape[-1],), weight=ln_w, bias=ln_b)
|
||||
kx = xx * k_mix + sx * (1 - k_mix)
|
||||
rx = xx * r_mix + sx * (1 - r_mix)
|
||||
|
||||
r = torch.sigmoid(gemm(rx, rw))
|
||||
vx = torch.square(torch.relu(gemm(kx, kw)))
|
||||
out = r * gemm(vx, vw)
|
||||
return x + out, xx
|
||||
*/
|
||||
Tensor ffn_one(Tensor x, Tensor sx, Tensor ln_w, Tensor ln_b, Tensor k_mix,
|
||||
Tensor r_mix, Tensor kw, Tensor vw, Tensor rw,
|
||||
/* imm */ Tensor buf,
|
||||
/* out */ Tensor x_plus_out) {
|
||||
Tensor xx = at::layer_norm(x, {x.size(-1)}, ln_w, ln_b);
|
||||
char *buf_ptr = (char *)buf.data_ptr();
|
||||
half *kx = (half *)buf_ptr;
|
||||
half *rx = kx + x.numel();
|
||||
half *vx = rx + x.numel();
|
||||
half *r = vx + x.size(0) * kw.size(1);
|
||||
element_wise(FfnOneMix{data_ptr<half>(k_mix), data_ptr<half>(r_mix),
|
||||
data_ptr<half>(xx), data_ptr<half>(sx), kx, rx},
|
||||
x.numel());
|
||||
// vector * matrix, so m = 1
|
||||
gemm_fp16_cublas(rx, rw.data_ptr(), r, 1, rw.size(1), rw.size(0), false);
|
||||
element_wise(InplaceSigmoid{r}, rw.size(1));
|
||||
gemm_fp16_cublas(kx, kw.data_ptr(), vx, 1, kw.size(1), kw.size(0), false);
|
||||
element_wise(InplaceReLUAndSquare{vx}, kw.size(1));
|
||||
gemm_fp16_cublas(vx, vw.data_ptr(), x_plus_out.data_ptr(), 1, vw.size(1),
|
||||
vw.size(0), false);
|
||||
element_wise(InplaceFma{data_ptr<half>(x_plus_out), r, data_ptr<half>(x)},
|
||||
x_plus_out.numel());
|
||||
return xx;
|
||||
}
|
@ -1,128 +0,0 @@
|
||||
#include <cublas_v2.h>
|
||||
#include <cuda.h>
|
||||
#include <cuda_fp16.h>
|
||||
#include <cuda_runtime.h>
|
||||
#include <torch/extension.h>
|
||||
|
||||
#define CUBLAS_CHECK(condition) \
|
||||
for (cublasStatus_t _cublas_check_status = (condition); \
|
||||
_cublas_check_status != CUBLAS_STATUS_SUCCESS;) \
|
||||
throw std::runtime_error("cuBLAS error " + \
|
||||
std::to_string(_cublas_check_status) + " at " + \
|
||||
std::to_string(__LINE__));
|
||||
|
||||
#define CUDA_CHECK(condition) \
|
||||
for (cudaError_t _cuda_check_status = (condition); \
|
||||
_cuda_check_status != cudaSuccess;) \
|
||||
throw std::runtime_error( \
|
||||
"CUDA error " + std::string(cudaGetErrorString(_cuda_check_status)) + \
|
||||
" at " + std::to_string(__LINE__));
|
||||
|
||||
cublasHandle_t get_cublas_handle() {
|
||||
static cublasHandle_t cublas_handle = []() {
|
||||
cublasHandle_t handle = nullptr;
|
||||
CUBLAS_CHECK(cublasCreate(&handle));
|
||||
#if CUDA_VERSION < 11000
|
||||
CUBLAS_CHECK(cublasSetMathMode(handle, CUBLAS_TENSOR_OP_MATH));
|
||||
#else
|
||||
CUBLAS_CHECK(cublasSetMathMode(handle, CUBLAS_DEFAULT_MATH));
|
||||
#endif // CUDA_VERSION < 11000
|
||||
return handle;
|
||||
}();
|
||||
return cublas_handle;
|
||||
}
|
||||
|
||||
/*
|
||||
NOTE: blas gemm is column-major by default, but we need row-major output.
|
||||
The data of row-major, transposed matrix is exactly the same as the
|
||||
column-major, non-transposed matrix, and C = A * B ---> C^T = B^T * A^T
|
||||
*/
|
||||
void gemm_fp16_cublas(const void *a, const void *b, void *c, int ori_m,
|
||||
int ori_n, int ori_k, bool output_fp32) {
|
||||
const auto cuda_data_type = CUDA_R_16F;
|
||||
const auto cuda_c_data_type = output_fp32 ? CUDA_R_32F : CUDA_R_16F;
|
||||
const auto compute_type = CUDA_R_32F;
|
||||
const float sp_alpha = 1.f;
|
||||
// use CUBLAS_OP_N. see the notes above
|
||||
const cublasOperation_t cublas_trans_a = CUBLAS_OP_N;
|
||||
const cublasOperation_t cublas_trans_b = CUBLAS_OP_N;
|
||||
// m = (B^T).size(0) = B.size(1) = n;
|
||||
const int cublas_m = ori_n;
|
||||
const int cublas_k = ori_k;
|
||||
// comptiable with rwkv one mode, where 1-D tensor * 2-D tensor
|
||||
// const int n = a.dense_dim() == 1 ? 1 : a.size(0);
|
||||
const int cublas_n = ori_m;
|
||||
const int cublas_lda = cublas_m;
|
||||
const int cublas_ldb = cublas_k;
|
||||
const int cublas_ldc = cublas_m;
|
||||
cublasHandle_t cublas_handle = get_cublas_handle();
|
||||
|
||||
#if CUDA_VERSION >= 11000
|
||||
cublasGemmAlgo_t algo = CUBLAS_GEMM_DEFAULT;
|
||||
#else
|
||||
cublasGemmAlgo_t algo = CUBLAS_GEMM_DFALT_TENSOR_OP;
|
||||
#endif
|
||||
const float sp_beta = 0.f;
|
||||
CUBLAS_CHECK(cublasGemmEx(
|
||||
cublas_handle, cublas_trans_a, cublas_trans_b, cublas_m, cublas_n,
|
||||
cublas_k, &sp_alpha, b, cuda_data_type, cublas_lda,
|
||||
a, cuda_data_type, cublas_ldb, &sp_beta, c,
|
||||
cuda_c_data_type, cublas_ldc, compute_type, algo));
|
||||
}
|
||||
|
||||
/*
|
||||
NOTE: blas gemm is column-major by default, but we need row-major output.
|
||||
The data of row-major, transposed matrix is exactly the same as the
|
||||
column-major, non-transposed matrix, and C = A * B ---> C^T = B^T * A^T
|
||||
*/
|
||||
void gemm_fp16_cublas_tensor(torch::Tensor a, torch::Tensor b, torch::Tensor c) {
|
||||
if (a.sizes().size() == 1) {
|
||||
assert(b.sizes().size() == 2);
|
||||
a = at::unsqueeze(a, 0);
|
||||
}
|
||||
const auto cuda_data_type = CUDA_R_16F;
|
||||
const auto cuda_c_data_type =
|
||||
c.dtype() == torch::kFloat32 ? CUDA_R_32F : CUDA_R_16F;
|
||||
const auto compute_type = CUDA_R_32F;
|
||||
const float sp_alpha = 1.f;
|
||||
// swap a and b, and use CUBLAS_OP_N. see the notes above
|
||||
std::swap(a, b);
|
||||
const cublasOperation_t cublas_trans_a = CUBLAS_OP_N;
|
||||
const cublasOperation_t cublas_trans_b = CUBLAS_OP_N;
|
||||
// m = (B^T).size(0) = B.size(1), and = A.size(1) after swap,
|
||||
// negative axis is used because of the existence of batch matmul.
|
||||
const int m = a.size(-1);
|
||||
const int k = a.size(-2);
|
||||
const int n = b.size(-2);
|
||||
const int cublas_lda = m;
|
||||
const int cublas_ldb = k;
|
||||
const int cublas_ldc = m;
|
||||
cublasHandle_t cublas_handle = get_cublas_handle();
|
||||
|
||||
#if CUDA_VERSION >= 11000
|
||||
cublasGemmAlgo_t algo = CUBLAS_GEMM_DEFAULT;
|
||||
#else
|
||||
cublasGemmAlgo_t algo = CUBLAS_GEMM_DFALT_TENSOR_OP;
|
||||
#endif
|
||||
const float sp_beta = 0.f;
|
||||
if (a.sizes().size() == 2 && b.sizes().size() == 2) {
|
||||
CUBLAS_CHECK(cublasGemmEx(
|
||||
cublas_handle, cublas_trans_a, cublas_trans_b, m, n, k, &sp_alpha,
|
||||
a.data_ptr(), cuda_data_type, cublas_lda, b.data_ptr(), cuda_data_type,
|
||||
cublas_ldb, &sp_beta, c.data_ptr(), cuda_c_data_type, cublas_ldc,
|
||||
compute_type, algo));
|
||||
} else {
|
||||
// batch matmul
|
||||
assert(a.sizes().size() == 3 && b.sizes().size() == 3);
|
||||
|
||||
const long long int cublas_stride_a = m * k;
|
||||
const long long int cublas_stride_b = k * n;
|
||||
const long long int cublas_stride_c = m * n;
|
||||
CUBLAS_CHECK(cublasGemmStridedBatchedEx(
|
||||
cublas_handle, cublas_trans_a, cublas_trans_b, m,
|
||||
n, k, &sp_alpha, a.data_ptr(), cuda_data_type, cublas_lda,
|
||||
cublas_stride_a, b.data_ptr(), cuda_data_type, cublas_ldb, cublas_stride_b,
|
||||
&sp_beta, c.data_ptr(), cuda_c_data_type, cublas_ldc, cublas_stride_c,
|
||||
a.size(0), compute_type, algo));
|
||||
}
|
||||
}
|
246
backend-python/rwkv_pip/beta/cuda/operators.cu
vendored
246
backend-python/rwkv_pip/beta/cuda/operators.cu
vendored
@ -1,246 +0,0 @@
|
||||
#include <stdio.h>
|
||||
#include <assert.h>
|
||||
#include "ATen/ATen.h"
|
||||
#include <cuda_fp16.h>
|
||||
#define MIN_VALUE (-1e38)
|
||||
typedef at::Half fp16;
|
||||
__half *cast(fp16 *ptr) {
|
||||
return reinterpret_cast<__half *>(ptr);
|
||||
}
|
||||
|
||||
template <typename F>
|
||||
__global__ void kernel_wkv_forward(const int B, const int T, const int C,
|
||||
const float *__restrict__ const _w, const float *__restrict__ const _u, const F *__restrict__ const _k, const F *__restrict__ const _v,
|
||||
F *__restrict__ const _y, float *__restrict__ const _aa, float *__restrict__ const _bb, float *__restrict__ const _pp) {
|
||||
const int idx = blockIdx.x * blockDim.x + threadIdx.x;
|
||||
const int _b = idx / C;
|
||||
const int _c = idx % C;
|
||||
const int _offset = _b * T * C + _c;
|
||||
const int _state_offset = _b * C + _c;
|
||||
|
||||
float u = _u[_c];
|
||||
float w = _w[_c];
|
||||
const F *__restrict__ const k = _k + _offset;
|
||||
const F *__restrict__ const v = _v + _offset;
|
||||
F *__restrict__ const y = _y + _offset;
|
||||
|
||||
float aa = _aa[_state_offset];
|
||||
float bb = _bb[_state_offset];
|
||||
float pp = _pp[_state_offset];
|
||||
for (int i = 0; i < T; i++) {
|
||||
const int ii = i * C;
|
||||
const float kk = float(k[ii]);
|
||||
const float vv = float(v[ii]);
|
||||
float ww = u + kk;
|
||||
float p = max(pp, ww);
|
||||
float e1 = exp(pp - p);
|
||||
float e2 = exp(ww - p);
|
||||
y[ii] = F((e1 * aa + e2 * vv) / (e1 * bb + e2));
|
||||
ww = w + pp;
|
||||
p = max(ww, kk);
|
||||
e1 = exp(ww - p);
|
||||
e2 = exp(kk - p);
|
||||
aa = e1 * aa + e2 * vv;
|
||||
bb = e1 * bb + e2;
|
||||
pp = p;
|
||||
}
|
||||
_aa[_state_offset] = aa;
|
||||
_bb[_state_offset] = bb;
|
||||
_pp[_state_offset] = pp;
|
||||
}
|
||||
|
||||
template <typename F>
|
||||
void cuda_wkv_forward(int B, int T, int C, float *w, float *u, F *k, F *v, F *y, float *aa, float *bb, float *pp) {
|
||||
dim3 threadsPerBlock( min(C, 32) );
|
||||
assert(B * C % threadsPerBlock.x == 0);
|
||||
dim3 numBlocks(B * C / threadsPerBlock.x);
|
||||
kernel_wkv_forward<<<numBlocks, threadsPerBlock>>>(B, T, C, w, u, k, v, y, aa, bb, pp);
|
||||
}
|
||||
|
||||
template void cuda_wkv_forward<fp16>(
|
||||
int B, int T, int C,
|
||||
float *w, float *u, fp16 *k, fp16 *v, fp16 *y,
|
||||
float *aa, float *bb, float *pp);
|
||||
template void cuda_wkv_forward<float>(
|
||||
int B, int T, int C,
|
||||
float *w, float *u, float *k, float *v, float *y,
|
||||
float *aa, float *bb, float *pp);
|
||||
|
||||
__global__ void kernel_mm_seq_fp32i8(
|
||||
const int B, const int N, const int M,
|
||||
const float *__restrict__ const x, const int x_stride,
|
||||
const uint8_t *__restrict__ const w, const int w_stride,
|
||||
const float *__restrict__ const mx,
|
||||
const float *__restrict__ const rx,
|
||||
const float *__restrict__ const my,
|
||||
const float *__restrict__ const ry,
|
||||
float *__restrict__ const y, const int y_stride) {
|
||||
|
||||
const int i = blockIdx.x * blockDim.x + threadIdx.x;
|
||||
const int k = blockIdx.y * blockDim.y + threadIdx.y;
|
||||
|
||||
if (i < B && k < M) {
|
||||
float y_local = 0;
|
||||
for (int j = 0; j < N; ++j) {
|
||||
y_local += x[i * x_stride + j] * (
|
||||
(float(w[j * w_stride + k]) + 0.5f)
|
||||
* rx[k] * ry[j] + mx[k] + my[j]
|
||||
);
|
||||
}
|
||||
y[i * y_stride + k] = y_local;
|
||||
}
|
||||
}
|
||||
|
||||
template <typename F>
|
||||
void cuda_mm8_seq(int B, int N, int M,
|
||||
F *x, int x_stride,
|
||||
uint8_t *w, int w_stride,
|
||||
F *mx, F *rx,
|
||||
F *my, F *ry,
|
||||
F *y, int y_stride);
|
||||
|
||||
template <>
|
||||
void cuda_mm8_seq<float>(int B, int N, int M,
|
||||
float *x, int x_stride,
|
||||
uint8_t *w, int w_stride,
|
||||
float *mx, float *rx,
|
||||
float *my, float *ry,
|
||||
float *y, int y_stride) {
|
||||
dim3 blockSize(1, 128);
|
||||
dim3 gridSize((B + blockSize.x - 1) / blockSize.x, (M + blockSize.y - 1) / blockSize.y);
|
||||
kernel_mm_seq_fp32i8<<<gridSize, blockSize>>>(
|
||||
B, N, M, x, x_stride, w, w_stride,
|
||||
mx, rx, my, ry, y, y_stride);
|
||||
}
|
||||
|
||||
__global__ void kernel_mm_seq_fp16i8(
|
||||
const int B, const int N, const int M,
|
||||
const __half *__restrict__ const x, const int x_stride,
|
||||
const uint8_t *__restrict__ const w, const int w_stride,
|
||||
const __half *__restrict__ const mx,
|
||||
const __half *__restrict__ const rx,
|
||||
const __half *__restrict__ const my,
|
||||
const __half *__restrict__ const ry,
|
||||
__half *__restrict__ const y, const int y_stride) {
|
||||
|
||||
const int i = blockIdx.x * blockDim.x + threadIdx.x;
|
||||
const int k = blockIdx.y * blockDim.y + threadIdx.y;
|
||||
|
||||
if (i < B && k < M) {
|
||||
float y_local = 0;
|
||||
for (int j = 0; j < N; ++j) {
|
||||
y_local += __half2float(x[i * x_stride + j]) * (
|
||||
(float(w[j * w_stride + k]) + 0.5f)
|
||||
* __half2float(rx[k]) * __half2float(ry[j])
|
||||
+ __half2float(mx[k]) + __half2float(my[j])
|
||||
);
|
||||
}
|
||||
y[i * y_stride + k] = __float2half(y_local);
|
||||
}
|
||||
}
|
||||
|
||||
template <>
|
||||
void cuda_mm8_seq<fp16>(int B, int N, int M,
|
||||
fp16 *x, int x_stride,
|
||||
uint8_t *w, int w_stride,
|
||||
fp16 *mx, fp16 *rx,
|
||||
fp16 *my, fp16 *ry,
|
||||
fp16 *y, int y_stride) {
|
||||
dim3 blockSize(1, 128);
|
||||
dim3 gridSize((B + blockSize.x - 1) / blockSize.x, (M + blockSize.y - 1) / blockSize.y);
|
||||
kernel_mm_seq_fp16i8<<<gridSize, blockSize>>>(
|
||||
B, N, M, cast(x), x_stride, w, w_stride,
|
||||
cast(mx), cast(rx), cast(my), cast(ry), cast(y), y_stride);
|
||||
}
|
||||
|
||||
#define MM8_ONE_JSPLIT 24
|
||||
#define MM8_ONE_TILE 1024
|
||||
|
||||
__global__ void kernel_mm_one_fp32i8(
|
||||
const int N, const int M,
|
||||
const float *__restrict__ const x,
|
||||
const uint8_t *__restrict__ const w, const int w_stride,
|
||||
const float *__restrict__ const mx,
|
||||
const float *__restrict__ const rx,
|
||||
const float *__restrict__ const my,
|
||||
const float *__restrict__ const ry,
|
||||
float *__restrict__ const y) {
|
||||
|
||||
const int k = blockIdx.y * blockDim.y + threadIdx.y;
|
||||
const int j0 = min(N, blockIdx.x * ((N + MM8_ONE_JSPLIT - 1) / MM8_ONE_JSPLIT));
|
||||
const int j1 = min(N, (blockIdx.x + 1) * ((N + MM8_ONE_JSPLIT - 1) / MM8_ONE_JSPLIT));
|
||||
|
||||
if (k < M) {
|
||||
float y_local = 0;
|
||||
for (int j = j0; j < j1; ++j) {
|
||||
y_local += x[j] * (
|
||||
(float(w[j * w_stride + k]) + 0.5f)
|
||||
* rx[k] * ry[j] + mx[k] + my[j]
|
||||
);
|
||||
}
|
||||
atomicAdd(&y[k], y_local);
|
||||
}
|
||||
}
|
||||
|
||||
template <typename F>
|
||||
void cuda_mm8_one(int N, int M,
|
||||
F *x,
|
||||
uint8_t *w, int w_stride,
|
||||
F *mx, F *rx,
|
||||
F *my, F *ry,
|
||||
float *y);
|
||||
|
||||
template <>
|
||||
void cuda_mm8_one<float>(int N, int M,
|
||||
float *x,
|
||||
uint8_t *w, int w_stride,
|
||||
float *mx, float *rx,
|
||||
float *my, float *ry,
|
||||
float *y) {
|
||||
dim3 blockSize(1, MM8_ONE_TILE);
|
||||
dim3 gridSize(MM8_ONE_JSPLIT, (M + blockSize.y - 1) / blockSize.y);
|
||||
kernel_mm_one_fp32i8<<<gridSize, blockSize>>>(
|
||||
N, M, x, w, w_stride,
|
||||
mx, rx, my, ry, y);
|
||||
}
|
||||
|
||||
__global__ void kernel_mm_one_fp16i8(
|
||||
const int N, const int M,
|
||||
const __half *__restrict__ const x,
|
||||
const uint8_t *__restrict__ const w, const int w_stride,
|
||||
const __half *__restrict__ const mx,
|
||||
const __half *__restrict__ const rx,
|
||||
const __half *__restrict__ const my,
|
||||
const __half *__restrict__ const ry,
|
||||
float *__restrict__ const y) {
|
||||
|
||||
const int k = blockIdx.y * blockDim.y + threadIdx.y;
|
||||
const int j0 = min(N, blockIdx.x * ((N + MM8_ONE_JSPLIT - 1) / MM8_ONE_JSPLIT));
|
||||
const int j1 = min(N, (blockIdx.x + 1) * ((N + MM8_ONE_JSPLIT - 1) / MM8_ONE_JSPLIT));
|
||||
|
||||
if (k < M) {
|
||||
float y_local = 0;
|
||||
for (int j = j0; j < j1; ++j) {
|
||||
y_local += __half2float(x[j]) * (
|
||||
(float(w[j * w_stride + k]) + 0.5f)
|
||||
* __half2float(rx[k]) * __half2float(ry[j])
|
||||
+ __half2float(mx[k]) + __half2float(my[j])
|
||||
);
|
||||
}
|
||||
atomicAdd(&y[k], y_local);
|
||||
}
|
||||
}
|
||||
|
||||
template <>
|
||||
void cuda_mm8_one<fp16>(int N, int M,
|
||||
fp16 *x,
|
||||
uint8_t *w, int w_stride,
|
||||
fp16 *mx, fp16 *rx,
|
||||
fp16 *my, fp16 *ry,
|
||||
float *y) {
|
||||
dim3 blockSize(1, MM8_ONE_TILE);
|
||||
dim3 gridSize(MM8_ONE_JSPLIT, (M + blockSize.y - 1) / blockSize.y);
|
||||
kernel_mm_one_fp16i8<<<gridSize, blockSize>>>(
|
||||
N, M, cast(x), w, w_stride,
|
||||
cast(mx), cast(rx), cast(my), cast(ry), y);
|
||||
}
|
7
backend-python/rwkv_pip/beta/cuda/util.h
vendored
7
backend-python/rwkv_pip/beta/cuda/util.h
vendored
@ -1,7 +0,0 @@
|
||||
#include "ATen/ATen.h"
|
||||
#include <cuda_fp16.h>
|
||||
|
||||
template <typename T> T *data_ptr(torch::Tensor x) { return x.data_ptr<T>(); }
|
||||
template <> inline half *data_ptr(torch::Tensor x) {
|
||||
return reinterpret_cast<half *>(x.data_ptr<at::Half>());
|
||||
}
|
181
backend-python/rwkv_pip/beta/cuda/wrapper.cpp
vendored
181
backend-python/rwkv_pip/beta/cuda/wrapper.cpp
vendored
@ -1,181 +0,0 @@
|
||||
#include <torch/extension.h>
|
||||
#include "ATen/ATen.h"
|
||||
#include <iostream>
|
||||
#include <c10/cuda/CUDAGuard.h>
|
||||
|
||||
typedef at::Half fp16;
|
||||
|
||||
template <typename F>
|
||||
void cuda_wkv_forward(int B, int T, int C,
|
||||
float *w, float *u, F *k, F *v, F *y,
|
||||
float *aa, float *bb, float *pp);
|
||||
template <typename F>
|
||||
void cuda_mm8_seq(int B, int N, int M,
|
||||
F *x, int x_stride,
|
||||
uint8_t *w, int w_stride,
|
||||
F *mx, F *rx,
|
||||
F *my, F *ry,
|
||||
F *y, int y_stride);
|
||||
template <typename F>
|
||||
void cuda_mm8_one(int N, int M,
|
||||
F *x,
|
||||
uint8_t *w, int w_stride,
|
||||
F *mx, F *rx,
|
||||
F *my, F *ry,
|
||||
float *y);
|
||||
|
||||
void wkv_forward(int64_t B, int64_t T, int64_t C,
|
||||
torch::Tensor &w, torch::Tensor &u,
|
||||
torch::Tensor &k, torch::Tensor &v, torch::Tensor &y,
|
||||
torch::Tensor &aa, torch::Tensor &bb, torch::Tensor &pp) {
|
||||
const at::cuda::OptionalCUDAGuard device_guard(device_of(w));
|
||||
switch (k.scalar_type()) {
|
||||
case c10::ScalarType::Half:
|
||||
cuda_wkv_forward(B, T, C,
|
||||
w.data_ptr<float>(), u.data_ptr<float>(),
|
||||
k.data_ptr<fp16>(), v.data_ptr<fp16>(), y.data_ptr<fp16>(),
|
||||
aa.data_ptr<float>(), bb.data_ptr<float>(), pp.data_ptr<float>());
|
||||
break;
|
||||
case c10::ScalarType::Float:
|
||||
cuda_wkv_forward(B, T, C,
|
||||
w.data_ptr<float>(), u.data_ptr<float>(),
|
||||
k.data_ptr<float>(), v.data_ptr<float>(), y.data_ptr<float>(),
|
||||
aa.data_ptr<float>(), bb.data_ptr<float>(), pp.data_ptr<float>());
|
||||
break;
|
||||
default:
|
||||
assert(false && "Only FP16 and FP32 are currently supported");
|
||||
}
|
||||
}
|
||||
|
||||
void mm8_seq(int64_t B, int64_t N, int64_t M,
|
||||
torch::Tensor &x, torch::Tensor &w,
|
||||
torch::Tensor &mx, torch::Tensor &rx,
|
||||
torch::Tensor &my, torch::Tensor &ry,
|
||||
torch::Tensor &y) {
|
||||
assert(x.stride(1) == 1);
|
||||
assert(w.stride(1) == 1);
|
||||
assert(mx.stride(0) == 1 && rx.stride(0) == 1);
|
||||
assert(my.stride(0) == 1 && ry.stride(0) == 1);
|
||||
assert(y.stride(1) == 1);
|
||||
const at::cuda::OptionalCUDAGuard device_guard(device_of(w));
|
||||
switch (x.scalar_type()) {
|
||||
case c10::ScalarType::Half:
|
||||
cuda_mm8_seq(
|
||||
B, N, M,
|
||||
x.data_ptr<fp16>(), x.stride(0),
|
||||
w.data_ptr<uint8_t>(), w.stride(0),
|
||||
mx.data_ptr<fp16>(), rx.data_ptr<fp16>(),
|
||||
my.data_ptr<fp16>(), ry.data_ptr<fp16>(),
|
||||
y.data_ptr<fp16>(), y.stride(0));
|
||||
break;
|
||||
case c10::ScalarType::Float:
|
||||
cuda_mm8_seq(
|
||||
B, N, M,
|
||||
x.data_ptr<float>(), x.stride(0),
|
||||
w.data_ptr<uint8_t>(), w.stride(0),
|
||||
mx.data_ptr<float>(), rx.data_ptr<float>(),
|
||||
my.data_ptr<float>(), ry.data_ptr<float>(),
|
||||
y.data_ptr<float>(), y.stride(0));
|
||||
break;
|
||||
default:
|
||||
assert(false && "Only FP16 and FP32 are currently supported");
|
||||
}
|
||||
}
|
||||
void mm8_one(int64_t N, int64_t M,
|
||||
torch::Tensor &x, torch::Tensor &w,
|
||||
torch::Tensor &mx, torch::Tensor &rx,
|
||||
torch::Tensor &my, torch::Tensor &ry,
|
||||
torch::Tensor &y) {
|
||||
assert(x.stride(0) == 1);
|
||||
assert(w.stride(1) == 1);
|
||||
assert(mx.stride(0) == 1 && rx.stride(0) == 1);
|
||||
assert(my.stride(0) == 1 && ry.stride(0) == 1);
|
||||
assert(y.stride(0) == 1);
|
||||
const at::cuda::OptionalCUDAGuard device_guard(device_of(w));
|
||||
switch (x.scalar_type()) {
|
||||
case c10::ScalarType::Half:
|
||||
cuda_mm8_one(
|
||||
N, M,
|
||||
x.data_ptr<fp16>(),
|
||||
w.data_ptr<uint8_t>(), w.stride(0),
|
||||
mx.data_ptr<fp16>(), rx.data_ptr<fp16>(),
|
||||
my.data_ptr<fp16>(), ry.data_ptr<fp16>(),
|
||||
y.data_ptr<float>());
|
||||
break;
|
||||
case c10::ScalarType::Float:
|
||||
cuda_mm8_one(
|
||||
N, M,
|
||||
x.data_ptr<float>(),
|
||||
w.data_ptr<uint8_t>(), w.stride(0),
|
||||
mx.data_ptr<float>(), rx.data_ptr<float>(),
|
||||
my.data_ptr<float>(), ry.data_ptr<float>(),
|
||||
y.data_ptr<float>());
|
||||
break;
|
||||
default:
|
||||
assert(false && "Only FP16 and FP32 are currently supported");
|
||||
}
|
||||
}
|
||||
|
||||
using torch::Tensor;
|
||||
|
||||
#ifndef DISABLE_CUBLAS_GEMM
|
||||
void gemm_fp16_cublas_tensor(Tensor a, Tensor b, Tensor c);
|
||||
#endif
|
||||
|
||||
Tensor att_one(Tensor x, Tensor ln_w, Tensor ln_b, Tensor sx, Tensor k_mix,
|
||||
Tensor v_mix, Tensor r_mix, Tensor kw,
|
||||
/* imm */ Tensor kx, Tensor vw, /* imm */ Tensor vx, Tensor rw,
|
||||
/* imm */ Tensor rx, Tensor ow, Tensor t_first,
|
||||
/* imm */ Tensor k, Tensor pp, Tensor ww, Tensor aa, Tensor bb,
|
||||
Tensor t_decay, /* imm */ Tensor v, /* in & out */ Tensor r,
|
||||
/* out */ Tensor x_plus_out, /* out */ Tensor t1,
|
||||
/* out */ Tensor t2, /* out */ Tensor p);
|
||||
|
||||
Tensor att_seq(Tensor x, Tensor sx, Tensor ln_w, Tensor ln_b, Tensor k_mix,
|
||||
Tensor v_mix, Tensor r_mix, Tensor kw, Tensor vw, Tensor rw,
|
||||
Tensor ow, Tensor t_first, Tensor pp, Tensor aa, Tensor bb,
|
||||
Tensor t_decay, /* imm */ Tensor buf, /* out */ Tensor x_plus_out);
|
||||
|
||||
Tensor att_one_v5(Tensor x, Tensor sx, Tensor s, Tensor ln_w, Tensor ln_b,
|
||||
Tensor lx_w, Tensor lx_b, Tensor k_mix, Tensor v_mix,
|
||||
Tensor r_mix, Tensor kw,
|
||||
/* imm */ Tensor kx, Tensor vw, /* imm */ Tensor vx,
|
||||
Tensor rw,
|
||||
/* imm */ Tensor rx, Tensor ow, Tensor t_first,
|
||||
/* imm */ Tensor k, Tensor t_decay, /* imm */ Tensor v,
|
||||
/* imm */ Tensor r, /* imm */ Tensor s1,
|
||||
/* out */ Tensor x_plus_out, /* out */ Tensor s2);
|
||||
|
||||
Tensor ffn_seq(Tensor x, Tensor sx, Tensor ln_w, Tensor ln_b, Tensor k_mix,
|
||||
Tensor r_mix, Tensor kw, Tensor vw, Tensor rw,
|
||||
/* imm */ Tensor buf,
|
||||
/* out */ Tensor x_plus_out);
|
||||
|
||||
Tensor ffn_one(Tensor x, Tensor sx, Tensor ln_w, Tensor ln_b, Tensor k_mix,
|
||||
Tensor r_mix, Tensor kw, Tensor vw, Tensor rw,
|
||||
/* imm */ Tensor buf,
|
||||
/* out */ Tensor x_plus_out);
|
||||
|
||||
PYBIND11_MODULE(TORCH_EXTENSION_NAME, m) {
|
||||
m.def("wkv_forward", &wkv_forward, "wkv forward");
|
||||
m.def("mm8_seq", &mm8_seq, "mm8 seq");
|
||||
m.def("mm8_one", &mm8_one, "mm8 one");
|
||||
m.def("gemm_fp16_cublas", &gemm_fp16_cublas_tensor, "gemv fp16 cublas");
|
||||
m.def("att_one", &att_one, "att one");
|
||||
m.def("att_one_v5", &att_one_v5, "att one v5");
|
||||
m.def("att_seq", &att_seq, "att seq");
|
||||
m.def("ffn_seq", &ffn_seq, "ffn seq");
|
||||
m.def("ffn_one", &ffn_one, "ffn one");
|
||||
}
|
||||
|
||||
TORCH_LIBRARY(rwkv, m) {
|
||||
m.def("wkv_forward", wkv_forward);
|
||||
m.def("mm8_seq", mm8_seq);
|
||||
m.def("mm8_one", mm8_one);
|
||||
m.def("gemm_fp16_cublas", gemm_fp16_cublas_tensor);
|
||||
m.def("att_one", att_one);
|
||||
m.def("att_one_v5", &att_one_v5);
|
||||
m.def("att_seq", att_seq);
|
||||
m.def("ffn_seq", ffn_seq);
|
||||
m.def("ffn_one", ffn_one);
|
||||
}
|
1821
backend-python/rwkv_pip/beta/model.py
vendored
1821
backend-python/rwkv_pip/beta/model.py
vendored
File diff suppressed because it is too large
Load Diff
BIN
backend-python/rwkv_pip/beta/wkv_cuda.pyd
vendored
BIN
backend-python/rwkv_pip/beta/wkv_cuda.pyd
vendored
Binary file not shown.
BIN
backend-python/rwkv_pip/cpp/librwkv.dylib
vendored
BIN
backend-python/rwkv_pip/cpp/librwkv.dylib
vendored
Binary file not shown.
BIN
backend-python/rwkv_pip/cpp/librwkv.so
vendored
BIN
backend-python/rwkv_pip/cpp/librwkv.so
vendored
Binary file not shown.
3
backend-python/rwkv_pip/cpp/model.py
vendored
3
backend-python/rwkv_pip/cpp/model.py
vendored
@ -9,6 +9,9 @@ class RWKV:
|
||||
self.model = rwkv_cpp_model.RWKVModel(self.library, model_path)
|
||||
self.w = {} # fake weight
|
||||
self.w["emb.weight"] = [0] * self.model.n_vocab
|
||||
self.version = (
|
||||
self.model.arch_version_major + self.model.arch_version_minor / 10
|
||||
)
|
||||
|
||||
def forward(self, tokens: List[int], state: Union[Any, None] = None):
|
||||
return self.model.eval_sequence_in_chunks(tokens, state, use_numpy=True)
|
||||
|
BIN
backend-python/rwkv_pip/cpp/rwkv.dll
vendored
BIN
backend-python/rwkv_pip/cpp/rwkv.dll
vendored
Binary file not shown.
57
backend-python/rwkv_pip/cpp/rwkv_cpp_model.py
vendored
57
backend-python/rwkv_pip/cpp/rwkv_cpp_model.py
vendored
@ -52,9 +52,14 @@ class RWKVModel:
|
||||
if 'gpu_layers_count' in kwargs:
|
||||
gpu_layer_count = kwargs['gpu_layers_count']
|
||||
|
||||
assert os.path.isfile(model_path), f'{model_path} is not a file'
|
||||
assert thread_count > 0, 'Thread count must be > 0'
|
||||
assert gpu_layer_count >= 0, 'GPU layer count must be >= 0'
|
||||
if not os.path.isfile(model_path):
|
||||
raise ValueError(f'{model_path} is not a file')
|
||||
|
||||
if not (thread_count > 0):
|
||||
raise ValueError('Thread count must be > 0')
|
||||
|
||||
if not (gpu_layer_count >= 0):
|
||||
raise ValueError('GPU layer count must be >= 0')
|
||||
|
||||
self._library: rwkv_cpp_shared_library.RWKVSharedLibrary = shared_library
|
||||
|
||||
@ -84,10 +89,19 @@ class RWKVModel:
|
||||
Count of layers to offload onto the GPU, must be >= 0.
|
||||
"""
|
||||
|
||||
assert layer_count >= 0, 'Layer count must be >= 0'
|
||||
if not (layer_count >= 0):
|
||||
raise ValueError('Layer count must be >= 0')
|
||||
|
||||
return self._library.rwkv_gpu_offload_layers(self._ctx, layer_count)
|
||||
|
||||
@property
|
||||
def arch_version_major(self) -> int:
|
||||
return self._library.rwkv_get_arch_version_major(self._ctx)
|
||||
|
||||
@property
|
||||
def arch_version_minor(self) -> int:
|
||||
return self._library.rwkv_get_arch_version_minor(self._ctx)
|
||||
|
||||
@property
|
||||
def n_vocab(self) -> int:
|
||||
return self._library.rwkv_get_n_vocab(self._ctx)
|
||||
@ -133,7 +147,8 @@ class RWKVModel:
|
||||
Logits vector of shape (n_vocab); state for the next step.
|
||||
"""
|
||||
|
||||
assert self._valid, 'Model was freed'
|
||||
if not self._valid:
|
||||
raise ValueError('Model was freed')
|
||||
|
||||
use_numpy = self._detect_numpy_usage([state_in, state_out, logits_out], use_numpy)
|
||||
|
||||
@ -207,7 +222,8 @@ class RWKVModel:
|
||||
Logits vector of shape (n_vocab); state for the next step.
|
||||
"""
|
||||
|
||||
assert self._valid, 'Model was freed'
|
||||
if not self._valid:
|
||||
raise ValueError('Model was freed')
|
||||
|
||||
use_numpy = self._detect_numpy_usage([state_in, state_out, logits_out], use_numpy)
|
||||
|
||||
@ -281,7 +297,8 @@ class RWKVModel:
|
||||
Logits vector of shape (n_vocab); state for the next step.
|
||||
"""
|
||||
|
||||
assert self._valid, 'Model was freed'
|
||||
if not self._valid:
|
||||
raise ValueError('Model was freed')
|
||||
|
||||
use_numpy = self._detect_numpy_usage([state_in, state_out, logits_out], use_numpy)
|
||||
|
||||
@ -320,7 +337,8 @@ class RWKVModel:
|
||||
The object must not be used anymore after calling this method.
|
||||
"""
|
||||
|
||||
assert self._valid, 'Already freed'
|
||||
if not self._valid:
|
||||
raise ValueError('Already freed')
|
||||
|
||||
self._valid = False
|
||||
|
||||
@ -344,16 +362,25 @@ class RWKVModel:
|
||||
def _validate_tensor(self, tensor: NumpyArrayOrPyTorchTensor, name: str, size: int) -> None:
|
||||
if self._is_pytorch_tensor(tensor):
|
||||
tensor: torch.Tensor = tensor
|
||||
assert tensor.device == torch.device('cpu'), f'{name} is not on CPU'
|
||||
assert tensor.dtype == torch.float32, f'{name} is not of type float32'
|
||||
assert tensor.shape == (size,), f'{name} has invalid shape {tensor.shape}, expected ({size})'
|
||||
assert tensor.is_contiguous(), f'{name} is not contiguous'
|
||||
|
||||
if tensor.device != torch.device('cpu'):
|
||||
raise ValueError(f'{name} is not on CPU')
|
||||
if tensor.dtype != torch.float32:
|
||||
raise ValueError(f'{name} is not of type float32')
|
||||
if tensor.shape != (size,):
|
||||
raise ValueError(f'{name} has invalid shape {tensor.shape}, expected ({size})')
|
||||
if not tensor.is_contiguous():
|
||||
raise ValueError(f'{name} is not contiguous')
|
||||
else:
|
||||
import numpy as np
|
||||
tensor: np.ndarray = tensor
|
||||
assert tensor.dtype == np.float32, f'{name} is not of type float32'
|
||||
assert tensor.shape == (size,), f'{name} has invalid shape {tensor.shape}, expected ({size})'
|
||||
assert tensor.data.contiguous, f'{name} is not contiguous'
|
||||
|
||||
if tensor.dtype != np.float32:
|
||||
raise ValueError(f'{name} is not of type float32')
|
||||
if tensor.shape != (size,):
|
||||
raise ValueError(f'{name} has invalid shape {tensor.shape}, expected ({size})')
|
||||
if not tensor.data.contiguous:
|
||||
raise ValueError(f'{name} is not contiguous')
|
||||
|
||||
def _get_data_ptr(self, tensor: NumpyArrayOrPyTorchTensor):
|
||||
if self._is_pytorch_tensor(tensor):
|
||||
|
@ -6,21 +6,22 @@ import platform
|
||||
from typing import Optional, List, Tuple, Callable
|
||||
|
||||
QUANTIZED_FORMAT_NAMES: Tuple[str, str, str, str, str] = (
|
||||
'Q4_0',
|
||||
'Q4_1',
|
||||
'Q5_0',
|
||||
'Q5_1',
|
||||
'Q8_0'
|
||||
"Q4_0",
|
||||
"Q4_1",
|
||||
"Q5_0",
|
||||
"Q5_1",
|
||||
"Q8_0",
|
||||
)
|
||||
|
||||
P_FLOAT = ctypes.POINTER(ctypes.c_float)
|
||||
P_INT = ctypes.POINTER(ctypes.c_int32)
|
||||
|
||||
class RWKVContext:
|
||||
|
||||
class RWKVContext:
|
||||
def __init__(self, ptr: ctypes.pointer) -> None:
|
||||
self.ptr: ctypes.pointer = ptr
|
||||
|
||||
|
||||
class RWKVSharedLibrary:
|
||||
"""
|
||||
Python wrapper around rwkv.cpp shared library.
|
||||
@ -39,7 +40,7 @@ class RWKVSharedLibrary:
|
||||
# When Python is greater than 3.8, we need to reprocess the custom dll
|
||||
# according to the documentation to prevent loading failure errors.
|
||||
# https://docs.python.org/3/whatsnew/3.8.html#ctypes
|
||||
if platform.system().lower() == 'windows':
|
||||
if platform.system().lower() == "windows":
|
||||
self.library = ctypes.CDLL(shared_library_path, winmode=0)
|
||||
else:
|
||||
self.library = ctypes.cdll.LoadLibrary(shared_library_path)
|
||||
@ -47,39 +48,48 @@ class RWKVSharedLibrary:
|
||||
self.library.rwkv_init_from_file.argtypes = [ctypes.c_char_p, ctypes.c_uint32]
|
||||
self.library.rwkv_init_from_file.restype = ctypes.c_void_p
|
||||
|
||||
self.library.rwkv_gpu_offload_layers.argtypes = [ctypes.c_void_p, ctypes.c_uint32]
|
||||
self.library.rwkv_gpu_offload_layers.argtypes = [
|
||||
ctypes.c_void_p,
|
||||
ctypes.c_uint32,
|
||||
]
|
||||
self.library.rwkv_gpu_offload_layers.restype = ctypes.c_bool
|
||||
|
||||
self.library.rwkv_eval.argtypes = [
|
||||
ctypes.c_void_p, # ctx
|
||||
ctypes.c_int32, # token
|
||||
P_FLOAT, # state_in
|
||||
P_FLOAT, # state_out
|
||||
P_FLOAT # logits_out
|
||||
ctypes.c_void_p, # ctx
|
||||
ctypes.c_int32, # token
|
||||
P_FLOAT, # state_in
|
||||
P_FLOAT, # state_out
|
||||
P_FLOAT, # logits_out
|
||||
]
|
||||
self.library.rwkv_eval.restype = ctypes.c_bool
|
||||
|
||||
self.library.rwkv_eval_sequence.argtypes = [
|
||||
ctypes.c_void_p, # ctx
|
||||
P_INT, # tokens
|
||||
ctypes.c_size_t, # token count
|
||||
P_FLOAT, # state_in
|
||||
P_FLOAT, # state_out
|
||||
P_FLOAT # logits_out
|
||||
ctypes.c_void_p, # ctx
|
||||
P_INT, # tokens
|
||||
ctypes.c_size_t, # token count
|
||||
P_FLOAT, # state_in
|
||||
P_FLOAT, # state_out
|
||||
P_FLOAT, # logits_out
|
||||
]
|
||||
self.library.rwkv_eval_sequence.restype = ctypes.c_bool
|
||||
|
||||
self.library.rwkv_eval_sequence_in_chunks.argtypes = [
|
||||
ctypes.c_void_p, # ctx
|
||||
P_INT, # tokens
|
||||
ctypes.c_size_t, # token count
|
||||
ctypes.c_size_t, # chunk size
|
||||
P_FLOAT, # state_in
|
||||
P_FLOAT, # state_out
|
||||
P_FLOAT # logits_out
|
||||
ctypes.c_void_p, # ctx
|
||||
P_INT, # tokens
|
||||
ctypes.c_size_t, # token count
|
||||
ctypes.c_size_t, # chunk size
|
||||
P_FLOAT, # state_in
|
||||
P_FLOAT, # state_out
|
||||
P_FLOAT, # logits_out
|
||||
]
|
||||
self.library.rwkv_eval_sequence_in_chunks.restype = ctypes.c_bool
|
||||
|
||||
self.library.rwkv_get_arch_version_major.argtypes = [ctypes.c_void_p]
|
||||
self.library.rwkv_get_arch_version_major.restype = ctypes.c_uint32
|
||||
|
||||
self.library.rwkv_get_arch_version_minor.argtypes = [ctypes.c_void_p]
|
||||
self.library.rwkv_get_arch_version_minor.restype = ctypes.c_uint32
|
||||
|
||||
self.library.rwkv_get_n_vocab.argtypes = [ctypes.c_void_p]
|
||||
self.library.rwkv_get_n_vocab.restype = ctypes.c_size_t
|
||||
|
||||
@ -101,7 +111,11 @@ class RWKVSharedLibrary:
|
||||
self.library.rwkv_free.argtypes = [ctypes.c_void_p]
|
||||
self.library.rwkv_free.restype = None
|
||||
|
||||
self.library.rwkv_quantize_model_file.argtypes = [ctypes.c_char_p, ctypes.c_char_p, ctypes.c_char_p]
|
||||
self.library.rwkv_quantize_model_file.argtypes = [
|
||||
ctypes.c_char_p,
|
||||
ctypes.c_char_p,
|
||||
ctypes.c_char_p,
|
||||
]
|
||||
self.library.rwkv_quantize_model_file.restype = ctypes.c_bool
|
||||
|
||||
self.library.rwkv_get_system_info_string.argtypes = []
|
||||
@ -109,7 +123,9 @@ class RWKVSharedLibrary:
|
||||
|
||||
self.nullptr = ctypes.cast(0, ctypes.c_void_p)
|
||||
|
||||
def rwkv_init_from_file(self, model_file_path: str, thread_count: int) -> RWKVContext:
|
||||
def rwkv_init_from_file(
|
||||
self, model_file_path: str, thread_count: int
|
||||
) -> RWKVContext:
|
||||
"""
|
||||
Loads the model from a file and prepares it for inference.
|
||||
Throws an exception in case of any error. Error messages would be printed to stderr.
|
||||
@ -122,9 +138,12 @@ class RWKVSharedLibrary:
|
||||
Count of threads to use, must be positive.
|
||||
"""
|
||||
|
||||
ptr = self.library.rwkv_init_from_file(model_file_path.encode('utf-8'), ctypes.c_uint32(thread_count))
|
||||
ptr = self.library.rwkv_init_from_file(
|
||||
model_file_path.encode("utf-8"), ctypes.c_uint32(thread_count)
|
||||
)
|
||||
|
||||
assert ptr is not None, 'rwkv_init_from_file failed, check stderr'
|
||||
if ptr is None:
|
||||
raise ValueError("rwkv_init_from_file failed, check stderr")
|
||||
|
||||
return RWKVContext(ptr)
|
||||
|
||||
@ -145,17 +164,20 @@ class RWKVSharedLibrary:
|
||||
Count of layers to offload onto the GPU, must be >= 0.
|
||||
"""
|
||||
|
||||
assert layer_count >= 0, 'Layer count must be >= 0'
|
||||
if not (layer_count >= 0):
|
||||
raise ValueError("Layer count must be >= 0")
|
||||
|
||||
return self.library.rwkv_gpu_offload_layers(ctx.ptr, ctypes.c_uint32(layer_count))
|
||||
return self.library.rwkv_gpu_offload_layers(
|
||||
ctx.ptr, ctypes.c_uint32(layer_count)
|
||||
)
|
||||
|
||||
def rwkv_eval(
|
||||
self,
|
||||
ctx: RWKVContext,
|
||||
token: int,
|
||||
state_in_address: Optional[int],
|
||||
state_out_address: int,
|
||||
logits_out_address: int
|
||||
self,
|
||||
ctx: RWKVContext,
|
||||
token: int,
|
||||
state_in_address: Optional[int],
|
||||
state_out_address: int,
|
||||
logits_out_address: int,
|
||||
) -> None:
|
||||
"""
|
||||
Evaluates the model for a single token.
|
||||
@ -176,21 +198,22 @@ class RWKVSharedLibrary:
|
||||
Address of the first element of a FP32 buffer of size rwkv_get_logits_buffer_element_count. This buffer will be written to.
|
||||
"""
|
||||
|
||||
assert self.library.rwkv_eval(
|
||||
if not self.library.rwkv_eval(
|
||||
ctx.ptr,
|
||||
ctypes.c_int32(token),
|
||||
ctypes.cast(0 if state_in_address is None else state_in_address, P_FLOAT),
|
||||
ctypes.cast(state_out_address, P_FLOAT),
|
||||
ctypes.cast(logits_out_address, P_FLOAT)
|
||||
), 'rwkv_eval failed, check stderr'
|
||||
ctypes.cast(logits_out_address, P_FLOAT),
|
||||
):
|
||||
raise ValueError("rwkv_eval failed, check stderr")
|
||||
|
||||
def rwkv_eval_sequence(
|
||||
self,
|
||||
ctx: RWKVContext,
|
||||
tokens: List[int],
|
||||
state_in_address: Optional[int],
|
||||
state_out_address: int,
|
||||
logits_out_address: int
|
||||
self,
|
||||
ctx: RWKVContext,
|
||||
tokens: List[int],
|
||||
state_in_address: Optional[int],
|
||||
state_out_address: int,
|
||||
logits_out_address: int,
|
||||
) -> None:
|
||||
"""
|
||||
Evaluates the model for a sequence of tokens.
|
||||
@ -223,23 +246,24 @@ class RWKVSharedLibrary:
|
||||
Address of the first element of a FP32 buffer of size rwkv_get_logits_buffer_element_count. This buffer will be written to.
|
||||
"""
|
||||
|
||||
assert self.library.rwkv_eval_sequence(
|
||||
if not self.library.rwkv_eval_sequence(
|
||||
ctx.ptr,
|
||||
ctypes.cast((ctypes.c_int32 * len(tokens))(*tokens), P_INT),
|
||||
ctypes.c_size_t(len(tokens)),
|
||||
ctypes.cast(0 if state_in_address is None else state_in_address, P_FLOAT),
|
||||
ctypes.cast(state_out_address, P_FLOAT),
|
||||
ctypes.cast(logits_out_address, P_FLOAT)
|
||||
), 'rwkv_eval_sequence failed, check stderr'
|
||||
ctypes.cast(logits_out_address, P_FLOAT),
|
||||
):
|
||||
raise ValueError("rwkv_eval_sequence failed, check stderr")
|
||||
|
||||
def rwkv_eval_sequence_in_chunks(
|
||||
self,
|
||||
ctx: RWKVContext,
|
||||
tokens: List[int],
|
||||
chunk_size: int,
|
||||
state_in_address: Optional[int],
|
||||
state_out_address: int,
|
||||
logits_out_address: int
|
||||
self,
|
||||
ctx: RWKVContext,
|
||||
tokens: List[int],
|
||||
chunk_size: int,
|
||||
state_in_address: Optional[int],
|
||||
state_out_address: int,
|
||||
logits_out_address: int,
|
||||
) -> None:
|
||||
"""
|
||||
Evaluates the model for a sequence of tokens using `rwkv_eval_sequence`, splitting a potentially long sequence into fixed-length chunks.
|
||||
@ -269,15 +293,40 @@ class RWKVSharedLibrary:
|
||||
Address of the first element of a FP32 buffer of size rwkv_get_logits_buffer_element_count. This buffer will be written to.
|
||||
"""
|
||||
|
||||
assert self.library.rwkv_eval_sequence_in_chunks(
|
||||
if not self.library.rwkv_eval_sequence_in_chunks(
|
||||
ctx.ptr,
|
||||
ctypes.cast((ctypes.c_int32 * len(tokens))(*tokens), P_INT),
|
||||
ctypes.c_size_t(len(tokens)),
|
||||
ctypes.c_size_t(chunk_size),
|
||||
ctypes.cast(0 if state_in_address is None else state_in_address, P_FLOAT),
|
||||
ctypes.cast(state_out_address, P_FLOAT),
|
||||
ctypes.cast(logits_out_address, P_FLOAT)
|
||||
), 'rwkv_eval_sequence_in_chunks failed, check stderr'
|
||||
ctypes.cast(logits_out_address, P_FLOAT),
|
||||
):
|
||||
raise ValueError("rwkv_eval_sequence_in_chunks failed, check stderr")
|
||||
|
||||
def rwkv_get_arch_version_major(self, ctx: RWKVContext) -> int:
|
||||
"""
|
||||
Returns the major version used by the given model.
|
||||
|
||||
Parameters
|
||||
----------
|
||||
ctx : RWKVContext
|
||||
RWKV context obtained from rwkv_init_from_file.
|
||||
"""
|
||||
|
||||
return self.library.rwkv_get_arch_version_major(ctx.ptr)
|
||||
|
||||
def rwkv_get_arch_version_minor(self, ctx: RWKVContext) -> int:
|
||||
"""
|
||||
Returns the minor version used by the given model.
|
||||
|
||||
Parameters
|
||||
----------
|
||||
ctx : RWKVContext
|
||||
RWKV context obtained from rwkv_init_from_file.
|
||||
"""
|
||||
|
||||
return self.library.rwkv_get_arch_version_minor(ctx.ptr)
|
||||
|
||||
def rwkv_get_n_vocab(self, ctx: RWKVContext) -> int:
|
||||
"""
|
||||
@ -358,7 +407,9 @@ class RWKVSharedLibrary:
|
||||
|
||||
ctx.ptr = self.nullptr
|
||||
|
||||
def rwkv_quantize_model_file(self, model_file_path_in: str, model_file_path_out: str, format_name: str) -> None:
|
||||
def rwkv_quantize_model_file(
|
||||
self, model_file_path_in: str, model_file_path_out: str, format_name: str
|
||||
) -> None:
|
||||
"""
|
||||
Quantizes FP32 or FP16 model to one of INT4 formats.
|
||||
Throws an exception in case of any error. Error messages would be printed to stderr.
|
||||
@ -373,20 +424,25 @@ class RWKVSharedLibrary:
|
||||
One of QUANTIZED_FORMAT_NAMES.
|
||||
"""
|
||||
|
||||
assert format_name in QUANTIZED_FORMAT_NAMES, f'Unknown format name {format_name}, use one of {QUANTIZED_FORMAT_NAMES}'
|
||||
if format_name not in QUANTIZED_FORMAT_NAMES:
|
||||
raise ValueError(
|
||||
f"Unknown format name {format_name}, use one of {QUANTIZED_FORMAT_NAMES}"
|
||||
)
|
||||
|
||||
assert self.library.rwkv_quantize_model_file(
|
||||
model_file_path_in.encode('utf-8'),
|
||||
model_file_path_out.encode('utf-8'),
|
||||
format_name.encode('utf-8')
|
||||
), 'rwkv_quantize_model_file failed, check stderr'
|
||||
if not self.library.rwkv_quantize_model_file(
|
||||
model_file_path_in.encode("utf-8"),
|
||||
model_file_path_out.encode("utf-8"),
|
||||
format_name.encode("utf-8"),
|
||||
):
|
||||
raise ValueError("rwkv_quantize_model_file failed, check stderr")
|
||||
|
||||
def rwkv_get_system_info_string(self) -> str:
|
||||
"""
|
||||
Returns system information string.
|
||||
"""
|
||||
|
||||
return self.library.rwkv_get_system_info_string().decode('utf-8')
|
||||
return self.library.rwkv_get_system_info_string().decode("utf-8")
|
||||
|
||||
|
||||
def load_rwkv_shared_library() -> RWKVSharedLibrary:
|
||||
"""
|
||||
@ -396,27 +452,27 @@ def load_rwkv_shared_library() -> RWKVSharedLibrary:
|
||||
|
||||
file_name: str
|
||||
|
||||
if 'win32' in sys.platform or 'cygwin' in sys.platform:
|
||||
file_name = 'rwkv.dll'
|
||||
elif 'darwin' in sys.platform:
|
||||
file_name = 'librwkv.dylib'
|
||||
if "win32" in sys.platform or "cygwin" in sys.platform:
|
||||
file_name = "rwkv.dll"
|
||||
elif "darwin" in sys.platform:
|
||||
file_name = "librwkv.dylib"
|
||||
else:
|
||||
file_name = 'librwkv.so'
|
||||
file_name = "librwkv.so"
|
||||
|
||||
# Possible sub-paths to the library relative to the repo dir.
|
||||
child_paths: List[Callable[[pathlib.Path], pathlib.Path]] = [
|
||||
# No lookup for Debug config here.
|
||||
# I assume that if a user wants to debug the library,
|
||||
# they will be able to find the library and set the exact path explicitly.
|
||||
lambda p: p / 'backend-python' / 'rwkv_pip' / 'cpp' / file_name,
|
||||
lambda p: p / 'bin' / 'Release' / file_name,
|
||||
lambda p: p / 'bin' / file_name,
|
||||
lambda p: p / "backend-python" / "rwkv_pip" / "cpp" / file_name,
|
||||
lambda p: p / "bin" / "Release" / file_name,
|
||||
lambda p: p / "bin" / file_name,
|
||||
# Some people prefer to build in the "build" subdirectory.
|
||||
lambda p: p / 'build' / 'bin' / 'Release' / file_name,
|
||||
lambda p: p / 'build' / 'bin' / file_name,
|
||||
lambda p: p / 'build' / file_name,
|
||||
lambda p: p / "build" / "bin" / "Release" / file_name,
|
||||
lambda p: p / "build" / "bin" / file_name,
|
||||
lambda p: p / "build" / file_name,
|
||||
# Fallback.
|
||||
lambda p: p / file_name
|
||||
lambda p: p / file_name,
|
||||
]
|
||||
|
||||
working_dir: pathlib.Path = pathlib.Path(os.path.abspath(os.getcwd()))
|
||||
@ -430,7 +486,7 @@ def load_rwkv_shared_library() -> RWKVSharedLibrary:
|
||||
# .
|
||||
working_dir,
|
||||
# Repo dir relative to this Python file.
|
||||
pathlib.Path(os.path.abspath(__file__)).parent.parent.parent
|
||||
pathlib.Path(os.path.abspath(__file__)).parent.parent.parent,
|
||||
]
|
||||
|
||||
for parent_path in parent_paths:
|
||||
@ -440,5 +496,7 @@ def load_rwkv_shared_library() -> RWKVSharedLibrary:
|
||||
if os.path.isfile(full_path):
|
||||
return RWKVSharedLibrary(str(full_path))
|
||||
|
||||
assert False, (f'Failed to find {file_name} automatically; '
|
||||
f'you need to find the library and create RWKVSharedLibrary specifying the path to it')
|
||||
raise ValueError(
|
||||
f"Failed to find {file_name} automatically; "
|
||||
f"you need to find the library and create RWKVSharedLibrary specifying the path to it"
|
||||
)
|
||||
|
57
backend-python/rwkv_pip/model.py
vendored
57
backend-python/rwkv_pip/model.py
vendored
@ -342,7 +342,7 @@ class RWKV(MyModule):
|
||||
)
|
||||
assert (
|
||||
w["_strategy"] == args.strategy_string
|
||||
) # if you are using a new strategy, re-convert the model
|
||||
), "model has been converted and does not match current strategy; if you are using a new strategy, re-convert the model"
|
||||
assert (
|
||||
float(w["_version"]) >= 0.7
|
||||
) # sometimes you should re-convert using latest convert_model.py
|
||||
@ -488,14 +488,19 @@ class RWKV(MyModule):
|
||||
print_need_newline = False
|
||||
|
||||
REAL_TIME_FIRST = False
|
||||
args.time_state = False
|
||||
for x in list(w.keys()):
|
||||
if ".time_faaaa" in x:
|
||||
REAL_TIME_FIRST = True
|
||||
if ".time_state" in x:
|
||||
args.time_state = True
|
||||
if REAL_TIME_FIRST:
|
||||
w = {
|
||||
k.replace(".time_faaaa", ".time_first")
|
||||
if ".time_faaaa" in k
|
||||
else k: v
|
||||
(
|
||||
k.replace(".time_faaaa", ".time_first")
|
||||
if ".time_faaaa" in k
|
||||
else k
|
||||
): v
|
||||
for k, v in w.items()
|
||||
}
|
||||
self.w = w
|
||||
@ -552,7 +557,12 @@ class RWKV(MyModule):
|
||||
elif ".ln_x" in x: # need fp32 for group_norm
|
||||
w[x] = w[x].float()
|
||||
else:
|
||||
if (len(w[x].shape) == 2) and ("emb" not in x):
|
||||
if (
|
||||
(len(w[x].shape) == 2)
|
||||
and ("emb" not in x)
|
||||
and ("_w1" not in x)
|
||||
and ("_w2" not in x)
|
||||
):
|
||||
if WTYPE != torch.uint8:
|
||||
w[x] = w[x].to(dtype=WTYPE)
|
||||
else:
|
||||
@ -626,10 +636,12 @@ class RWKV(MyModule):
|
||||
torch.cuda.empty_cache()
|
||||
|
||||
shape = [i for i in w[x].shape if i != 1]
|
||||
if len(shape) > 1:
|
||||
shape = f" {str(shape[0]).rjust(5)} {str(shape[1]).rjust(5)}"
|
||||
if len(shape) > 2:
|
||||
shape = f" {str(shape[0]).rjust(5)} {str(shape[1]).rjust(5)} {str(shape[2]).rjust(5)}"
|
||||
elif len(shape) > 1:
|
||||
shape = f" {str(shape[0]).rjust(5)} {str(shape[1]).rjust(5)} "
|
||||
else:
|
||||
shape = f" {str(shape[0]).rjust(5)} "
|
||||
shape = f" {str(shape[0]).rjust(5)} "
|
||||
if layer_id == 0 or layer_id >= args.n_layer - 1:
|
||||
if print_need_newline:
|
||||
prxxx("\n", end="")
|
||||
@ -2103,16 +2115,25 @@ class RWKV(MyModule):
|
||||
state[i * 3 + 0] = torch.zeros(
|
||||
args.n_embd, dtype=atype, requires_grad=False, device=dev
|
||||
).contiguous()
|
||||
state[i * 3 + 1] = torch.zeros(
|
||||
(
|
||||
args.n_head,
|
||||
args.n_att // args.n_head,
|
||||
args.n_att // args.n_head,
|
||||
),
|
||||
dtype=torch.float,
|
||||
requires_grad=False,
|
||||
device=dev,
|
||||
).contiguous()
|
||||
if args.time_state:
|
||||
state[i * 3 + 1] = (
|
||||
w[f"blocks.{i}.att.time_state"]
|
||||
.transpose(1, 2)
|
||||
.to(dtype=torch.float, device=dev)
|
||||
.requires_grad_(False)
|
||||
.contiguous()
|
||||
)
|
||||
else:
|
||||
state[i * 3 + 1] = torch.zeros(
|
||||
(
|
||||
args.n_head,
|
||||
args.n_att // args.n_head,
|
||||
args.n_att // args.n_head,
|
||||
),
|
||||
dtype=torch.float,
|
||||
requires_grad=False,
|
||||
device=dev,
|
||||
).contiguous()
|
||||
state[i * 3 + 2] = torch.zeros(
|
||||
args.n_embd, dtype=atype, requires_grad=False, device=dev
|
||||
).contiguous()
|
||||
|
65532
backend-python/rwkv_pip/rwkv_vocab_v20230424_special_token.txt
vendored
Normal file
65532
backend-python/rwkv_pip/rwkv_vocab_v20230424_special_token.txt
vendored
Normal file
File diff suppressed because it is too large
Load Diff
2224
backend-python/rwkv_pip/tokenizer-midipiano.json
vendored
Normal file
2224
backend-python/rwkv_pip/tokenizer-midipiano.json
vendored
Normal file
File diff suppressed because it is too large
Load Diff
32
backend-python/rwkv_pip/utils.py
vendored
32
backend-python/rwkv_pip/utils.py
vendored
@ -34,6 +34,25 @@ class PIPELINE_ARGS:
|
||||
)
|
||||
|
||||
|
||||
class ABC_TOKENIZER:
|
||||
def __init__(self):
|
||||
self.pad_token_id = 0
|
||||
self.bos_token_id = 2
|
||||
self.eos_token_id = 3
|
||||
|
||||
def encode(self, text):
|
||||
ids = [ord(c) for c in text]
|
||||
return ids
|
||||
|
||||
def decode(self, ids):
|
||||
txt = "".join(
|
||||
chr(idx) if idx > self.eos_token_id else ""
|
||||
for idx in ids
|
||||
if idx != self.eos_token_id
|
||||
)
|
||||
return txt
|
||||
|
||||
|
||||
class PIPELINE:
|
||||
def __init__(self, model, WORD_NAME: str):
|
||||
self.model = model
|
||||
@ -48,6 +67,8 @@ class PIPELINE:
|
||||
self.tokenizer = TRIE_TOKENIZER(
|
||||
os.path.dirname(os.path.abspath(__file__)) + "/rwkv_vocab_v20230424.txt"
|
||||
)
|
||||
elif WORD_NAME == "abc_tokenizer":
|
||||
self.tokenizer = ABC_TOKENIZER()
|
||||
else:
|
||||
if WORD_NAME.endswith(".txt"):
|
||||
sys.path.insert(0, os.path.dirname(os.path.abspath(__file__)))
|
||||
@ -150,10 +171,17 @@ class PIPELINE:
|
||||
all_tokens += [token]
|
||||
for xxx in occurrence:
|
||||
occurrence[xxx] *= args.alpha_decay
|
||||
|
||||
ttt = self.decode([token])
|
||||
www = 1
|
||||
if ttt in " \t0123456789":
|
||||
www = 0
|
||||
# elif ttt in '\r\n,.;?!"\':+-*/=#@$%^&_`~|<>\\()[]{},。;“”:?!()【】':
|
||||
# www = 0.5
|
||||
if token not in occurrence:
|
||||
occurrence[token] = 1
|
||||
occurrence[token] = www
|
||||
else:
|
||||
occurrence[token] += 1
|
||||
occurrence[token] += www
|
||||
# print(occurrence) # debug
|
||||
|
||||
# output
|
||||
|
38
backend-python/rwkv_pip/webgpu/model.py
vendored
38
backend-python/rwkv_pip/webgpu/model.py
vendored
@ -13,14 +13,38 @@ except ModuleNotFoundError:
|
||||
|
||||
class RWKV:
|
||||
def __init__(self, model_path: str, strategy: str = None):
|
||||
self.model = wrp.v5.Model(
|
||||
model_path,
|
||||
turbo=False,
|
||||
quant=32 if "i8" in strategy else None,
|
||||
quant_nf4=26 if "i4" in strategy else None,
|
||||
layer = (
|
||||
int(s.lstrip("layer"))
|
||||
for s in strategy.split()
|
||||
for s in s.split(",")
|
||||
if s.startswith("layer")
|
||||
)
|
||||
|
||||
chunk_size = (
|
||||
int(s.lstrip("chunk"))
|
||||
for s in strategy.split()
|
||||
for s in s.split(",")
|
||||
if s.startswith("chunk")
|
||||
)
|
||||
self.token_chunk_size = next(chunk_size, 32)
|
||||
|
||||
args = {
|
||||
"path": model_path,
|
||||
"quant": next(layer, 31) if "i8" in strategy else 0,
|
||||
"quant_nf4": next(layer, 26) if "i4" in strategy else 0,
|
||||
}
|
||||
self.model = wrp.Model(**args)
|
||||
self.info = self.model.info()
|
||||
self.w = {} # fake weight
|
||||
self.w["emb.weight"] = [0] * wrp.peek_info(model_path).num_vocab
|
||||
self.w["emb.weight"] = [0] * self.info.num_vocab
|
||||
self.version = str(self.info.version).lower()
|
||||
self.version = float(self.version.lower().replace("v", ""))
|
||||
|
||||
def forward(self, tokens: List[int], state: Union[Any, None] = None):
|
||||
return wrp.v5.run_one(self.model, tokens, state)
|
||||
if state is None:
|
||||
self.model.clear_state()
|
||||
elif type(state).__name__ == "State_Cpu":
|
||||
self.model.load_state(state)
|
||||
logits = self.model.run(tokens, self.token_chunk_size)
|
||||
ret_state = "State_Gpu"
|
||||
return logits, ret_state
|
||||
|
Binary file not shown.
@ -1,5 +1,5 @@
|
||||
{
|
||||
"deduplicate_md5": true,
|
||||
"piece_split_delay": 10.0,
|
||||
"min_piece_length": 30.0
|
||||
"piece_split_delay": 10000,
|
||||
"min_piece_length": 0
|
||||
}
|
@ -4,18 +4,14 @@ import os
|
||||
import pathlib
|
||||
import copy
|
||||
import re
|
||||
from typing import Dict, Iterable, List, Tuple, Union, Type
|
||||
import time
|
||||
from typing import Dict, Iterable, List, Tuple, Union, Type, Callable
|
||||
from utils.log import quick_log
|
||||
from fastapi import HTTPException
|
||||
from fastapi import HTTPException, status
|
||||
from pydantic import BaseModel, Field
|
||||
from routes import state_cache
|
||||
import global_var
|
||||
|
||||
|
||||
END_OF_TEXT = 0
|
||||
END_OF_LINE_DOUBLE = 535
|
||||
|
||||
|
||||
os.environ["TORCH_EXTENSIONS_DIR"] = f"{pathlib.Path(__file__).parent.parent.resolve()}"
|
||||
|
||||
|
||||
@ -28,7 +24,11 @@ class RWKVType(Enum):
|
||||
|
||||
class AbstractRWKV(ABC):
|
||||
def __init__(self, model, pipeline):
|
||||
self.EOS_ID = 0
|
||||
|
||||
self.name = "rwkv"
|
||||
self.model_path = ""
|
||||
self.version = 4
|
||||
self.model = model
|
||||
self.pipeline = pipeline
|
||||
self.model_state = None
|
||||
@ -42,6 +42,10 @@ class AbstractRWKV(ABC):
|
||||
self.top_k = 0
|
||||
self.penalty_alpha_presence = 0
|
||||
self.penalty_alpha_frequency = 1
|
||||
self.penalty_decay = 0.99
|
||||
self.global_penalty = False
|
||||
self.state_path = ""
|
||||
self.state_tuned = None
|
||||
|
||||
@abstractmethod
|
||||
def adjust_occurrence(self, occurrence: Dict, token: int):
|
||||
@ -235,19 +239,29 @@ class AbstractRWKV(ABC):
|
||||
except HTTPException:
|
||||
pass
|
||||
if cache is None or cache["prompt"] == "" or cache["state"] is None:
|
||||
self.model_state = None
|
||||
if self.state_path:
|
||||
self.model_state = copy.deepcopy(self.state_tuned)
|
||||
else:
|
||||
self.model_state = None
|
||||
self.model_tokens = []
|
||||
else:
|
||||
delta_prompt = prompt[len(cache["prompt"]) :]
|
||||
self.model_state = copy.deepcopy(cache["state"])
|
||||
self.model_tokens = copy.deepcopy(cache["tokens"])
|
||||
logits = copy.deepcopy(cache["logits"])
|
||||
self.model_state = cache["state"]
|
||||
self.model_tokens = cache["tokens"]
|
||||
logits = cache["logits"]
|
||||
|
||||
prompt_token_len = 0
|
||||
if delta_prompt != "":
|
||||
prompt_start_time = time.time()
|
||||
logits, prompt_token_len = self.run_rnn(
|
||||
self.fix_tokens(self.pipeline.encode(delta_prompt))
|
||||
)
|
||||
prompt_end_time = time.time()
|
||||
prompt_interval = prompt_end_time - prompt_start_time
|
||||
tps = 0
|
||||
if prompt_interval > 0:
|
||||
tps = prompt_token_len / prompt_interval
|
||||
print(f"Prompt Prefill TPS: {tps:.2f}", end=" ", flush=True)
|
||||
try:
|
||||
state_cache.add_state(
|
||||
state_cache.AddStateBody(
|
||||
@ -274,7 +288,18 @@ class AbstractRWKV(ABC):
|
||||
logits, temperature=self.temperature, top_p=self.top_p, top_k=self.top_k
|
||||
)
|
||||
|
||||
if token == END_OF_TEXT:
|
||||
if token == self.EOS_ID:
|
||||
try:
|
||||
state_cache.add_state(
|
||||
state_cache.AddStateBody(
|
||||
prompt=prompt + response,
|
||||
tokens=self.model_tokens,
|
||||
state=self.model_state,
|
||||
logits=logits,
|
||||
)
|
||||
)
|
||||
except HTTPException:
|
||||
pass
|
||||
yield response, "", prompt_token_len, completion_token_len
|
||||
break
|
||||
|
||||
@ -305,22 +330,25 @@ class AbstractRWKV(ABC):
|
||||
yield response, "", prompt_token_len, completion_token_len
|
||||
break
|
||||
elif type(stop) == list:
|
||||
stop_exist_regex = "|".join(stop)
|
||||
matched = re.search(stop_exist_regex, response)
|
||||
if matched:
|
||||
try:
|
||||
state_cache.add_state(
|
||||
state_cache.AddStateBody(
|
||||
prompt=prompt + response,
|
||||
tokens=self.model_tokens,
|
||||
state=self.model_state,
|
||||
logits=logits,
|
||||
exit_flag = False
|
||||
for s in stop:
|
||||
if s in response:
|
||||
try:
|
||||
state_cache.add_state(
|
||||
state_cache.AddStateBody(
|
||||
prompt=prompt + response,
|
||||
tokens=self.model_tokens,
|
||||
state=self.model_state,
|
||||
logits=logits,
|
||||
)
|
||||
)
|
||||
)
|
||||
except HTTPException:
|
||||
pass
|
||||
response = response.split(matched.group())[0]
|
||||
yield response, "", prompt_token_len, completion_token_len
|
||||
except HTTPException:
|
||||
pass
|
||||
exit_flag = True
|
||||
response = response.split(s)[0]
|
||||
yield response, "", prompt_token_len, completion_token_len
|
||||
break
|
||||
if exit_flag:
|
||||
break
|
||||
out_last = begin + i + 1
|
||||
if i == self.max_tokens_per_generation - 1:
|
||||
@ -363,18 +391,24 @@ class TextRWKV(AbstractRWKV):
|
||||
self.bot = "Assistant"
|
||||
self.END_OF_LINE = 11
|
||||
|
||||
self.AVOID_REPEAT_TOKENS = []
|
||||
self.AVOID_REPEAT_TOKENS = set()
|
||||
AVOID_REPEAT = ",:?!"
|
||||
for i in AVOID_REPEAT:
|
||||
dd = self.pipeline.encode(i)
|
||||
assert len(dd) == 1
|
||||
self.AVOID_REPEAT_TOKENS += dd
|
||||
self.AVOID_REPEAT_TOKENS.add(dd[0])
|
||||
self.AVOID_PENALTY_TOKENS = set()
|
||||
AVOID_PENALTY = '\n,.:?!,。:?!"“”<>[]{}/\\|;;~`@#$%^&*()_+-=0123456789 '
|
||||
for i in AVOID_PENALTY:
|
||||
dd = self.pipeline.encode(i)
|
||||
if len(dd) == 1:
|
||||
self.AVOID_PENALTY_TOKENS.add(dd[0])
|
||||
|
||||
self.__preload()
|
||||
|
||||
def adjust_occurrence(self, occurrence: Dict, token: int):
|
||||
for xxx in occurrence:
|
||||
occurrence[xxx] *= 0.996
|
||||
occurrence[xxx] *= self.penalty_decay
|
||||
if token not in occurrence:
|
||||
occurrence[token] = 1
|
||||
else:
|
||||
@ -382,26 +416,24 @@ class TextRWKV(AbstractRWKV):
|
||||
|
||||
def adjust_forward_logits(self, logits: List[float], occurrence: Dict, i: int):
|
||||
for n in occurrence:
|
||||
# if n not in self.AVOID_PENALTY_TOKENS:
|
||||
logits[n] -= (
|
||||
self.penalty_alpha_presence
|
||||
+ occurrence[n] * self.penalty_alpha_frequency
|
||||
)
|
||||
|
||||
if i == 0:
|
||||
# set global_penalty to False to get the same generated results as the official RWKV Gradio
|
||||
if self.global_penalty and i == 0:
|
||||
for token in self.model_tokens:
|
||||
token = int(token)
|
||||
for xxx in occurrence:
|
||||
occurrence[xxx] *= 0.996
|
||||
if token not in occurrence:
|
||||
occurrence[token] = 1
|
||||
else:
|
||||
occurrence[token] += 1
|
||||
if token not in self.AVOID_PENALTY_TOKENS:
|
||||
self.adjust_occurrence(occurrence, token)
|
||||
|
||||
# Model only saw '\n\n' as [187, 187] before, but the tokenizer outputs [535] for it at the end
|
||||
def fix_tokens(self, tokens) -> List[int]:
|
||||
if self.rwkv_type == RWKVType.World:
|
||||
return tokens
|
||||
if len(tokens) > 0 and tokens[-1] == END_OF_LINE_DOUBLE:
|
||||
if len(tokens) > 0 and tokens[-1] == 535:
|
||||
tokens = tokens[:-1] + [self.END_OF_LINE, self.END_OF_LINE]
|
||||
return tokens
|
||||
|
||||
@ -459,7 +491,7 @@ The following is a coherent verbose detailed conversation between a girl named {
|
||||
pass
|
||||
|
||||
|
||||
class MusicRWKV(AbstractRWKV):
|
||||
class MusicMidiRWKV(AbstractRWKV):
|
||||
def __init__(self, model, pipeline):
|
||||
super().__init__(model, pipeline)
|
||||
|
||||
@ -501,8 +533,47 @@ class MusicRWKV(AbstractRWKV):
|
||||
return " " + delta
|
||||
|
||||
|
||||
class MusicAbcRWKV(AbstractRWKV):
|
||||
def __init__(self, model, pipeline):
|
||||
super().__init__(model, pipeline)
|
||||
|
||||
self.EOS_ID = 3
|
||||
|
||||
self.max_tokens_per_generation = 500
|
||||
self.temperature = 1
|
||||
self.top_p = 0.8
|
||||
self.top_k = 8
|
||||
|
||||
self.rwkv_type = RWKVType.Music
|
||||
|
||||
def adjust_occurrence(self, occurrence: Dict, token: int):
|
||||
pass
|
||||
|
||||
def adjust_forward_logits(self, logits: List[float], occurrence: Dict, i: int):
|
||||
pass
|
||||
|
||||
def fix_tokens(self, tokens) -> List[int]:
|
||||
return tokens
|
||||
|
||||
def run_rnn(
|
||||
self, _tokens: List[str], newline_adj: int = 0
|
||||
) -> Tuple[List[float], int]:
|
||||
tokens = [int(x) for x in _tokens]
|
||||
token_len = len(tokens)
|
||||
self.model_tokens += tokens
|
||||
out, self.model_state = self.model.forward(tokens, self.model_state)
|
||||
return out, token_len
|
||||
|
||||
def delta_postprocess(self, delta: str) -> str:
|
||||
return delta
|
||||
|
||||
|
||||
def get_tokenizer(tokenizer_len: int):
|
||||
tokenizer_dir = f"{pathlib.Path(__file__).parent.parent.resolve()}/rwkv_pip/"
|
||||
if tokenizer_len < 2176:
|
||||
return "abc_tokenizer"
|
||||
if tokenizer_len < 20096:
|
||||
return tokenizer_dir + "tokenizer-midipiano.json"
|
||||
if tokenizer_len < 50277:
|
||||
return tokenizer_dir + "tokenizer-midi.json"
|
||||
elif tokenizer_len < 65536:
|
||||
@ -511,21 +582,49 @@ def get_tokenizer(tokenizer_len: int):
|
||||
return "rwkv_vocab_v20230424"
|
||||
|
||||
|
||||
def get_model_path(model_path: str) -> str:
|
||||
if os.path.isabs(model_path):
|
||||
return model_path
|
||||
|
||||
working_dir: pathlib.Path = pathlib.Path(os.path.abspath(os.getcwd()))
|
||||
|
||||
parent_paths: List[pathlib.Path] = [
|
||||
working_dir, # [cwd](RWKV-Runner)/models/xxx
|
||||
working_dir.parent, # [cwd](backend-python)/../models/xxx
|
||||
pathlib.Path(
|
||||
os.path.abspath(__file__)
|
||||
).parent.parent, # backend-python/models/xxx
|
||||
pathlib.Path(
|
||||
os.path.abspath(__file__)
|
||||
).parent.parent.parent, # RWKV-Runner/models/xxx
|
||||
]
|
||||
|
||||
child_paths: List[Callable[[pathlib.Path], pathlib.Path]] = [
|
||||
lambda p: p / model_path,
|
||||
lambda p: p / "build" / "bin" / model_path, # for dev
|
||||
]
|
||||
|
||||
for parent_path in parent_paths:
|
||||
for child_path in child_paths:
|
||||
full_path: pathlib.Path = child_path(parent_path)
|
||||
|
||||
if os.path.isfile(full_path):
|
||||
return str(full_path)
|
||||
|
||||
return model_path
|
||||
|
||||
|
||||
def RWKV(model: str, strategy: str, tokenizer: Union[str, None]) -> AbstractRWKV:
|
||||
rwkv_beta = global_var.get(global_var.Args).rwkv_beta
|
||||
model_path = get_model_path(model)
|
||||
|
||||
rwkv_cpp = getattr(global_var.get(global_var.Args), "rwkv.cpp")
|
||||
webgpu = global_var.get(global_var.Args).webgpu
|
||||
|
||||
if "midi" in model.lower() or "abc" in model.lower():
|
||||
if "midi" in model_path.lower() or "abc" in model_path.lower():
|
||||
os.environ["RWKV_RESCALE_LAYER"] = "999"
|
||||
|
||||
# dynamic import to make RWKV_CUDA_ON work
|
||||
if rwkv_beta:
|
||||
print("Using rwkv-beta")
|
||||
from rwkv_pip.beta.model import (
|
||||
RWKV as Model,
|
||||
)
|
||||
elif rwkv_cpp:
|
||||
if rwkv_cpp:
|
||||
print("Using rwkv.cpp, strategy is ignored")
|
||||
from rwkv_pip.cpp.model import (
|
||||
RWKV as Model,
|
||||
@ -541,8 +640,8 @@ def RWKV(model: str, strategy: str, tokenizer: Union[str, None]) -> AbstractRWKV
|
||||
)
|
||||
from rwkv_pip.utils import PIPELINE
|
||||
|
||||
filename, _ = os.path.splitext(os.path.basename(model))
|
||||
model = Model(model, strategy)
|
||||
filename, _ = os.path.splitext(os.path.basename(model_path))
|
||||
model = Model(model_path, strategy)
|
||||
if not tokenizer:
|
||||
tokenizer = get_tokenizer(len(model.w["emb.weight"]))
|
||||
pipeline = PIPELINE(model, tokenizer)
|
||||
@ -550,39 +649,152 @@ def RWKV(model: str, strategy: str, tokenizer: Union[str, None]) -> AbstractRWKV
|
||||
rwkv_map: dict[str, Type[AbstractRWKV]] = {
|
||||
"20B_tokenizer": TextRWKV,
|
||||
"rwkv_vocab_v20230424": TextRWKV,
|
||||
"tokenizer-midi": MusicRWKV,
|
||||
"tokenizer-midi": MusicMidiRWKV,
|
||||
"tokenizer-midipiano": MusicMidiRWKV,
|
||||
"abc_tokenizer": MusicAbcRWKV,
|
||||
}
|
||||
tokenizer_name = os.path.splitext(os.path.basename(tokenizer))[0]
|
||||
global_var.set(
|
||||
global_var.Midi_Vocab_Config_Type,
|
||||
(
|
||||
global_var.MidiVocabConfig.Piano
|
||||
if tokenizer_name == "tokenizer-midipiano"
|
||||
else global_var.MidiVocabConfig.Default
|
||||
),
|
||||
)
|
||||
rwkv: AbstractRWKV
|
||||
if tokenizer_name in rwkv_map:
|
||||
rwkv = rwkv_map[tokenizer_name](model, pipeline)
|
||||
else:
|
||||
rwkv = TextRWKV(model, pipeline)
|
||||
tokenizer_name = tokenizer_name.lower()
|
||||
if "music" in tokenizer_name or "midi" in tokenizer_name:
|
||||
rwkv = MusicMidiRWKV(model, pipeline)
|
||||
elif "abc" in tokenizer_name:
|
||||
rwkv = MusicAbcRWKV(model, pipeline)
|
||||
else:
|
||||
rwkv = TextRWKV(model, pipeline)
|
||||
rwkv.name = filename
|
||||
rwkv.model_path = model_path
|
||||
rwkv.version = model.version
|
||||
|
||||
return rwkv
|
||||
|
||||
|
||||
class ModelConfigBody(BaseModel):
|
||||
max_tokens: int = Field(default=None, gt=0, le=102400)
|
||||
temperature: float = Field(default=None, ge=0, le=2)
|
||||
temperature: float = Field(default=None, ge=0, le=3)
|
||||
top_p: float = Field(default=None, ge=0, le=1)
|
||||
presence_penalty: float = Field(default=None, ge=-2, le=2)
|
||||
frequency_penalty: float = Field(default=None, ge=-2, le=2)
|
||||
penalty_decay: float = Field(default=None, ge=0.99, le=0.999)
|
||||
top_k: int = Field(default=None, ge=0, le=25)
|
||||
global_penalty: bool = Field(
|
||||
default=None,
|
||||
description="When generating a response, whether to include the submitted prompt as a penalty factor. By turning this off, you will get the same generated results as official RWKV Gradio. If you find duplicate results in the generated results, turning this on can help avoid generating duplicates.",
|
||||
)
|
||||
state: str = Field(default=None, description="state-tuned file path")
|
||||
|
||||
model_config = {
|
||||
"json_schema_extra": {
|
||||
"example": {
|
||||
"max_tokens": 1000,
|
||||
"temperature": 1.2,
|
||||
"top_p": 0.5,
|
||||
"presence_penalty": 0.4,
|
||||
"frequency_penalty": 0.4,
|
||||
"temperature": 1,
|
||||
"top_p": 0.3,
|
||||
"presence_penalty": 0,
|
||||
"frequency_penalty": 1,
|
||||
"penalty_decay": 0.996,
|
||||
"global_penalty": False,
|
||||
"state": "",
|
||||
}
|
||||
}
|
||||
}
|
||||
|
||||
|
||||
def load_rwkv_state(
|
||||
model: AbstractRWKV, state_path: str, print_log: bool = True
|
||||
) -> HTTPException:
|
||||
if model:
|
||||
if state_path:
|
||||
if model.model_path.endswith(".pth") and state_path.endswith(".pth"):
|
||||
import torch
|
||||
|
||||
state_path = get_model_path(state_path)
|
||||
if model.state_path == state_path:
|
||||
return
|
||||
|
||||
if not os.path.isfile(state_path):
|
||||
return HTTPException(
|
||||
status.HTTP_400_BAD_REQUEST, "state file not found"
|
||||
)
|
||||
|
||||
try:
|
||||
state_raw = torch.load(state_path, map_location="cpu")
|
||||
except Exception as e:
|
||||
print(e)
|
||||
return HTTPException(
|
||||
status.HTTP_400_BAD_REQUEST, "state file failed to load"
|
||||
)
|
||||
state_raw_shape = next(iter(state_raw.values())).shape
|
||||
|
||||
args = model.model.args
|
||||
if (
|
||||
len(state_raw) != args.n_layer
|
||||
or state_raw_shape[0] * state_raw_shape[1] != args.n_embd
|
||||
):
|
||||
if model.state_path:
|
||||
pass
|
||||
elif print_log:
|
||||
print("state failed to load")
|
||||
return HTTPException(
|
||||
status.HTTP_400_BAD_REQUEST, "state shape mismatch"
|
||||
)
|
||||
|
||||
strategy = model.model.strategy
|
||||
model.state_tuned = [None] * args.n_layer * 3
|
||||
|
||||
for i in range(args.n_layer):
|
||||
dd = strategy[i]
|
||||
dev = dd.device
|
||||
atype = dd.atype
|
||||
model.state_tuned[i * 3 + 0] = torch.zeros(
|
||||
args.n_embd, dtype=atype, requires_grad=False, device=dev
|
||||
).contiguous()
|
||||
model.state_tuned[i * 3 + 1] = (
|
||||
state_raw[f"blocks.{i}.att.time_state"]
|
||||
.transpose(1, 2)
|
||||
.to(dtype=torch.float, device=dev)
|
||||
.requires_grad_(False)
|
||||
.contiguous()
|
||||
)
|
||||
model.state_tuned[i * 3 + 2] = torch.zeros(
|
||||
args.n_embd, dtype=atype, requires_grad=False, device=dev
|
||||
).contiguous()
|
||||
|
||||
state_cache.force_reset_state()
|
||||
model.state_path = state_path
|
||||
if print_log:
|
||||
print("state loaded")
|
||||
else:
|
||||
if model.state_path:
|
||||
pass
|
||||
elif print_log:
|
||||
print("state failed to load")
|
||||
return HTTPException(
|
||||
status.HTTP_400_BAD_REQUEST,
|
||||
"file format of the model or state model not supported",
|
||||
)
|
||||
else:
|
||||
if state_path == "" and model.state_path != "":
|
||||
state_cache.force_reset_state()
|
||||
model.state_path = ""
|
||||
model.state_tuned = None # TODO cached
|
||||
if print_log:
|
||||
print("state unloaded")
|
||||
else:
|
||||
if print_log:
|
||||
print("state not loaded")
|
||||
|
||||
|
||||
def set_rwkv_config(model: AbstractRWKV, body: ModelConfigBody):
|
||||
if body.max_tokens is not None:
|
||||
model.max_tokens_per_generation = body.max_tokens
|
||||
@ -597,6 +809,14 @@ def set_rwkv_config(model: AbstractRWKV, body: ModelConfigBody):
|
||||
model.penalty_alpha_presence = body.presence_penalty
|
||||
if body.frequency_penalty is not None:
|
||||
model.penalty_alpha_frequency = body.frequency_penalty
|
||||
if body.penalty_decay is not None:
|
||||
model.penalty_decay = body.penalty_decay
|
||||
if body.top_k is not None:
|
||||
model.top_k = body.top_k
|
||||
if body.global_penalty is not None:
|
||||
model.global_penalty = body.global_penalty
|
||||
if body.state is not None:
|
||||
load_rwkv_state(model, body.state, False)
|
||||
|
||||
|
||||
def get_rwkv_config(model: AbstractRWKV) -> ModelConfigBody:
|
||||
@ -606,4 +826,8 @@ def get_rwkv_config(model: AbstractRWKV) -> ModelConfigBody:
|
||||
top_p=model.top_p,
|
||||
presence_penalty=model.penalty_alpha_presence,
|
||||
frequency_penalty=model.penalty_alpha_frequency,
|
||||
penalty_decay=model.penalty_decay,
|
||||
top_k=model.top_k,
|
||||
global_penalty=model.global_penalty,
|
||||
state=model.state_path,
|
||||
)
|
||||
|
@ -19,9 +19,12 @@ def set_torch():
|
||||
|
||||
|
||||
def torch_gc():
|
||||
import torch
|
||||
try:
|
||||
import torch
|
||||
|
||||
if torch.cuda.is_available():
|
||||
with torch.cuda.device(0):
|
||||
torch.cuda.empty_cache()
|
||||
torch.cuda.ipc_collect()
|
||||
if torch.cuda.is_available():
|
||||
with torch.cuda.device(0):
|
||||
torch.cuda.empty_cache()
|
||||
torch.cuda.ipc_collect()
|
||||
except:
|
||||
pass # prevent 'torch' has no attribute 'cuda' error, so user can use CPU or WebGPU
|
||||
|
279
backend-python/utils/vocab_config_piano.json
Normal file
279
backend-python/utils/vocab_config_piano.json
Normal file
@ -0,0 +1,279 @@
|
||||
{
|
||||
"note_events": 128,
|
||||
"wait_events": 125,
|
||||
"max_wait_time": 1000,
|
||||
"velocity_events": 128,
|
||||
"velocity_bins": 16,
|
||||
"velocity_exp": 0.33,
|
||||
"do_token_sorting": true,
|
||||
"unrolled_tokens": false,
|
||||
"decode_end_held_note_delay": 5.0,
|
||||
"decode_fix_repeated_notes": true,
|
||||
"bin_instrument_names": [
|
||||
"piano"
|
||||
],
|
||||
"ch10_instrument_bin_name": "",
|
||||
"program_name_to_bin_name": {
|
||||
"Acoustic Grand Piano": "piano",
|
||||
"Bright Acoustic Piano": "piano",
|
||||
"Electric Grand Piano": "piano",
|
||||
"Honky-tonk Piano": "piano",
|
||||
"Electric Piano 1 (Rhodes Piano)": "piano",
|
||||
"Electric Piano 2 (Chorused Piano)": "piano",
|
||||
"Harpsichord": "piano",
|
||||
"Clavinet": "piano",
|
||||
"Celesta": "",
|
||||
"Glockenspiel": "",
|
||||
"Music Box": "",
|
||||
"Vibraphone": "",
|
||||
"Marimba": "",
|
||||
"Xylophone": "",
|
||||
"Tubular Bells": "",
|
||||
"Dulcimer (Santur)": "",
|
||||
"Drawbar Organ (Hammond)": "",
|
||||
"Percussive Organ": "piano",
|
||||
"Rock Organ": "piano",
|
||||
"Church Organ": "piano",
|
||||
"Reed Organ": "piano",
|
||||
"Accordion (French)": "piano",
|
||||
"Harmonica": "piano",
|
||||
"Tango Accordion (Band neon)": "piano",
|
||||
"Acoustic Guitar (nylon)": "",
|
||||
"Acoustic Guitar (steel)": "",
|
||||
"Electric Guitar (jazz)": "",
|
||||
"Electric Guitar (clean)": "",
|
||||
"Electric Guitar (muted)": "",
|
||||
"Overdriven Guitar": "",
|
||||
"Distortion Guitar": "",
|
||||
"Guitar harmonics": "",
|
||||
"Acoustic Bass": "",
|
||||
"Electric Bass (fingered)": "",
|
||||
"Electric Bass (picked)": "",
|
||||
"Fretless Bass": "",
|
||||
"Slap Bass 1": "",
|
||||
"Slap Bass 2": "",
|
||||
"Synth Bass 1": "",
|
||||
"Synth Bass 2": "",
|
||||
"Violin": "",
|
||||
"Viola": "",
|
||||
"Cello": "",
|
||||
"Contrabass": "",
|
||||
"Tremolo Strings": "",
|
||||
"Pizzicato Strings": "",
|
||||
"Orchestral Harp": "",
|
||||
"Timpani": "",
|
||||
"String Ensemble 1 (strings)": "",
|
||||
"String Ensemble 2 (slow strings)": "",
|
||||
"SynthStrings 1": "",
|
||||
"SynthStrings 2": "",
|
||||
"Choir Aahs": "",
|
||||
"Voice Oohs": "",
|
||||
"Synth Voice": "",
|
||||
"Orchestra Hit": "",
|
||||
"Trumpet": "",
|
||||
"Trombone": "",
|
||||
"Tuba": "",
|
||||
"Muted Trumpet": "",
|
||||
"French Horn": "",
|
||||
"Brass Section": "",
|
||||
"SynthBrass 1": "",
|
||||
"SynthBrass 2": "",
|
||||
"Soprano Sax": "",
|
||||
"Alto Sax": "",
|
||||
"Tenor Sax": "",
|
||||
"Baritone Sax": "",
|
||||
"Oboe": "",
|
||||
"English Horn": "",
|
||||
"Bassoon": "",
|
||||
"Clarinet": "",
|
||||
"Piccolo": "",
|
||||
"Flute": "",
|
||||
"Recorder": "",
|
||||
"Pan Flute": "",
|
||||
"Blown Bottle": "",
|
||||
"Shakuhachi": "",
|
||||
"Whistle": "",
|
||||
"Ocarina": "",
|
||||
"Lead 1 (square wave)": "",
|
||||
"Lead 2 (sawtooth wave)": "",
|
||||
"Lead 3 (calliope)": "",
|
||||
"Lead 4 (chiffer)": "",
|
||||
"Lead 5 (charang)": "",
|
||||
"Lead 6 (voice solo)": "",
|
||||
"Lead 7 (fifths)": "",
|
||||
"Lead 8 (bass + lead)": "",
|
||||
"Pad 1 (new age Fantasia)": "",
|
||||
"Pad 2 (warm)": "",
|
||||
"Pad 3 (polysynth)": "",
|
||||
"Pad 4 (choir space voice)": "",
|
||||
"Pad 5 (bowed glass)": "",
|
||||
"Pad 6 (metallic pro)": "",
|
||||
"Pad 7 (halo)": "",
|
||||
"Pad 8 (sweep)": "",
|
||||
"FX 1 (rain)": "",
|
||||
"FX 2 (soundtrack)": "",
|
||||
"FX 3 (crystal)": "",
|
||||
"FX 4 (atmosphere)": "",
|
||||
"FX 5 (brightness)": "",
|
||||
"FX 6 (goblins)": "",
|
||||
"FX 7 (echoes, drops)": "",
|
||||
"FX 8 (sci-fi, star theme)": "",
|
||||
"Sitar": "",
|
||||
"Banjo": "",
|
||||
"Shamisen": "",
|
||||
"Koto": "",
|
||||
"Kalimba": "",
|
||||
"Bag pipe": "",
|
||||
"Fiddle": "",
|
||||
"Shanai": "",
|
||||
"Tinkle Bell": "",
|
||||
"Agogo": "",
|
||||
"Steel Drums": "",
|
||||
"Woodblock": "",
|
||||
"Taiko Drum": "",
|
||||
"Melodic Tom": "",
|
||||
"Synth Drum": "",
|
||||
"Reverse Cymbal": "",
|
||||
"Guitar Fret Noise": "",
|
||||
"Breath Noise": "",
|
||||
"Seashore": "",
|
||||
"Bird Tweet": "",
|
||||
"Telephone Ring": "",
|
||||
"Helicopter": "",
|
||||
"Applause": "",
|
||||
"Gunshot": ""
|
||||
},
|
||||
"bin_name_to_program_name": {
|
||||
"piano": "Acoustic Grand Piano"
|
||||
},
|
||||
"instrument_names": {
|
||||
"0": "Acoustic Grand Piano",
|
||||
"1": "Bright Acoustic Piano",
|
||||
"2": "Electric Grand Piano",
|
||||
"3": "Honky-tonk Piano",
|
||||
"4": "Electric Piano 1 (Rhodes Piano)",
|
||||
"5": "Electric Piano 2 (Chorused Piano)",
|
||||
"6": "Harpsichord",
|
||||
"7": "Clavinet",
|
||||
"8": "Celesta",
|
||||
"9": "Glockenspiel",
|
||||
"10": "Music Box",
|
||||
"11": "Vibraphone",
|
||||
"12": "Marimba",
|
||||
"13": "Xylophone",
|
||||
"14": "Tubular Bells",
|
||||
"15": "Dulcimer (Santur)",
|
||||
"16": "Drawbar Organ (Hammond)",
|
||||
"17": "Percussive Organ",
|
||||
"18": "Rock Organ",
|
||||
"19": "Church Organ",
|
||||
"20": "Reed Organ",
|
||||
"21": "Accordion (French)",
|
||||
"22": "Harmonica",
|
||||
"23": "Tango Accordion (Band neon)",
|
||||
"24": "Acoustic Guitar (nylon)",
|
||||
"25": "Acoustic Guitar (steel)",
|
||||
"26": "Electric Guitar (jazz)",
|
||||
"27": "Electric Guitar (clean)",
|
||||
"28": "Electric Guitar (muted)",
|
||||
"29": "Overdriven Guitar",
|
||||
"30": "Distortion Guitar",
|
||||
"31": "Guitar harmonics",
|
||||
"32": "Acoustic Bass",
|
||||
"33": "Electric Bass (fingered)",
|
||||
"34": "Electric Bass (picked)",
|
||||
"35": "Fretless Bass",
|
||||
"36": "Slap Bass 1",
|
||||
"37": "Slap Bass 2",
|
||||
"38": "Synth Bass 1",
|
||||
"39": "Synth Bass 2",
|
||||
"40": "Violin",
|
||||
"41": "Viola",
|
||||
"42": "Cello",
|
||||
"43": "Contrabass",
|
||||
"44": "Tremolo Strings",
|
||||
"45": "Pizzicato Strings",
|
||||
"46": "Orchestral Harp",
|
||||
"47": "Timpani",
|
||||
"48": "String Ensemble 1 (strings)",
|
||||
"49": "String Ensemble 2 (slow strings)",
|
||||
"50": "SynthStrings 1",
|
||||
"51": "SynthStrings 2",
|
||||
"52": "Choir Aahs",
|
||||
"53": "Voice Oohs",
|
||||
"54": "Synth Voice",
|
||||
"55": "Orchestra Hit",
|
||||
"56": "Trumpet",
|
||||
"57": "Trombone",
|
||||
"58": "Tuba",
|
||||
"59": "Muted Trumpet",
|
||||
"60": "French Horn",
|
||||
"61": "Brass Section",
|
||||
"62": "SynthBrass 1",
|
||||
"63": "SynthBrass 2",
|
||||
"64": "Soprano Sax",
|
||||
"65": "Alto Sax",
|
||||
"66": "Tenor Sax",
|
||||
"67": "Baritone Sax",
|
||||
"68": "Oboe",
|
||||
"69": "English Horn",
|
||||
"70": "Bassoon",
|
||||
"71": "Clarinet",
|
||||
"72": "Piccolo",
|
||||
"73": "Flute",
|
||||
"74": "Recorder",
|
||||
"75": "Pan Flute",
|
||||
"76": "Blown Bottle",
|
||||
"77": "Shakuhachi",
|
||||
"78": "Whistle",
|
||||
"79": "Ocarina",
|
||||
"80": "Lead 1 (square wave)",
|
||||
"81": "Lead 2 (sawtooth wave)",
|
||||
"82": "Lead 3 (calliope)",
|
||||
"83": "Lead 4 (chiffer)",
|
||||
"84": "Lead 5 (charang)",
|
||||
"85": "Lead 6 (voice solo)",
|
||||
"86": "Lead 7 (fifths)",
|
||||
"87": "Lead 8 (bass + lead)",
|
||||
"88": "Pad 1 (new age Fantasia)",
|
||||
"89": "Pad 2 (warm)",
|
||||
"90": "Pad 3 (polysynth)",
|
||||
"91": "Pad 4 (choir space voice)",
|
||||
"92": "Pad 5 (bowed glass)",
|
||||
"93": "Pad 6 (metallic pro)",
|
||||
"94": "Pad 7 (halo)",
|
||||
"95": "Pad 8 (sweep)",
|
||||
"96": "FX 1 (rain)",
|
||||
"97": "FX 2 (soundtrack)",
|
||||
"98": "FX 3 (crystal)",
|
||||
"99": "FX 4 (atmosphere)",
|
||||
"100": "FX 5 (brightness)",
|
||||
"101": "FX 6 (goblins)",
|
||||
"102": "FX 7 (echoes, drops)",
|
||||
"103": "FX 8 (sci-fi, star theme)",
|
||||
"104": "Sitar",
|
||||
"105": "Banjo",
|
||||
"106": "Shamisen",
|
||||
"107": "Koto",
|
||||
"108": "Kalimba",
|
||||
"109": "Bag pipe",
|
||||
"110": "Fiddle",
|
||||
"111": "Shanai",
|
||||
"112": "Tinkle Bell",
|
||||
"113": "Agogo",
|
||||
"114": "Steel Drums",
|
||||
"115": "Woodblock",
|
||||
"116": "Taiko Drum",
|
||||
"117": "Melodic Tom",
|
||||
"118": "Synth Drum",
|
||||
"119": "Reverse Cymbal",
|
||||
"120": "Guitar Fret Noise",
|
||||
"121": "Breath Noise",
|
||||
"122": "Seashore",
|
||||
"123": "Bird Tweet",
|
||||
"124": "Telephone Ring",
|
||||
"125": "Helicopter",
|
||||
"126": "Applause",
|
||||
"127": "Gunshot"
|
||||
}
|
||||
}
|
18
docker-compose.yml
Normal file
18
docker-compose.yml
Normal file
@ -0,0 +1,18 @@
|
||||
services:
|
||||
rmkv_runner:
|
||||
image: rwkv-runner:latest
|
||||
build: .
|
||||
# Append "--rwkv.cpp" parameter to use rwkv.cpp
|
||||
# command: python3.10 ./backend-python/main.py --port 27777 --host 0.0.0.0 --webui --rwkv.cpp
|
||||
volumes:
|
||||
- /mnt:/mnt
|
||||
ports:
|
||||
- "27777:27777"
|
||||
# Comment the following lines if use rwkv.cpp
|
||||
deploy:
|
||||
resources:
|
||||
reservations:
|
||||
devices:
|
||||
- driver: nvidia
|
||||
count: 1
|
||||
capabilities: [gpu]
|
@ -19,14 +19,15 @@ document.querySelectorAll('.grid.h-10.grid-cols-12.place-content-center.gap-x-3.
|
||||
if (!data.name.endsWith('.bin') && !data.name.endsWith('.pth'))
|
||||
return
|
||||
|
||||
data.desc = {en: '', zh: ''}
|
||||
data.desc = { en: '', zh: '', ja: '' }
|
||||
const rawText = await (await fetch(e.children[1].href.replace('/resolve/', '/raw/'))).text()
|
||||
|
||||
data.size = parseInt(extractValue(rawText, 'size'))
|
||||
data.SHA256 = extractValue(rawText, 'oid sha256:')
|
||||
data.lastUpdated = e.children[3].children[0].getAttribute('datetime')
|
||||
data.url = e.children[1].href.replace('/resolve/', '/blob/')
|
||||
data.downloadUrl = e.children[1].href
|
||||
data.url = e.children[1].href.replace('/resolve/', '/blob/').replace('?download=true', '')
|
||||
data.downloadUrl = e.children[1].href.replace('?download=true', '')
|
||||
data.tags = []
|
||||
|
||||
modelsJson.push(data)
|
||||
})
|
||||
|
@ -32,6 +32,7 @@ cleaner_thread.start()
|
||||
w = torch.load(model_file, map_location="cpu")
|
||||
gc.collect()
|
||||
|
||||
vocab_size = w["emb.weight"].shape[0]
|
||||
n_embd = w["emb.weight"].shape[1]
|
||||
n_layer = 0
|
||||
keys = list(w.keys())
|
||||
@ -51,7 +52,14 @@ for x in keys:
|
||||
if "time_maa" in x:
|
||||
version = max(6, version)
|
||||
|
||||
params = f"--vocab_size {vocab_size} --n_layer {n_layer} --n_embd {n_embd}"
|
||||
|
||||
if version <= expected_max_version:
|
||||
print(f"--n_layer {n_layer} --n_embd {n_embd}", end="")
|
||||
if version == 6:
|
||||
params += ' --my_testing "x060"'
|
||||
print(
|
||||
f"v{int(version)}/train.py {params}",
|
||||
end="",
|
||||
)
|
||||
else:
|
||||
raise Exception(f"RWKV{version} is not supported")
|
||||
|
@ -1,7 +1,7 @@
|
||||
echo $@
|
||||
|
||||
if [[ ${cnMirror} == 1 ]]; then
|
||||
export PIP_INDEX_URL="https://pypi.tuna.tsinghua.edu.cn/simple"
|
||||
export PIP_INDEX_URL="https://mirrors.aliyun.com/pypi/simple"
|
||||
if grep -q "mirrors.aliyun.com" /etc/apt/sources.list; then
|
||||
echo "apt cnMirror already set"
|
||||
else
|
||||
@ -22,6 +22,12 @@ else
|
||||
sudo apt -y install python3-pip
|
||||
fi
|
||||
|
||||
if dpkg -s "python3-dev" >/dev/null 2>&1; then
|
||||
echo "python3-dev installed"
|
||||
else
|
||||
sudo apt -y install python3-dev
|
||||
fi
|
||||
|
||||
if dpkg -s "ninja-build" >/dev/null 2>&1; then
|
||||
echo "ninja installed"
|
||||
else
|
||||
@ -47,11 +53,12 @@ else
|
||||
fi
|
||||
|
||||
echo "loading $loadModel"
|
||||
modelInfo=$(python3 ./finetune/get_layer_and_embd.py $loadModel 4)
|
||||
modelInfo=$(python3 ./finetune/get_layer_and_embd.py $loadModel 6.0)
|
||||
echo $modelInfo
|
||||
if [[ $modelInfo =~ "--n_layer" ]]; then
|
||||
python3 ./finetune/lora/train.py $modelInfo $@ --proj_dir lora-models --data_type binidx --lora \
|
||||
--lora_parts=att,ffn,time,ln --strategy deepspeed_stage_2 --accelerator gpu
|
||||
sudo rm -rf /root/.cache/torch_extensions
|
||||
python3 ./finetune/lora/$modelInfo $@ --proj_dir lora-models --data_type binidx --lora \
|
||||
--lora_parts=att,ffn,time,ln --strategy deepspeed_stage_2 --accelerator gpu --ds_bucket_mb 2
|
||||
else
|
||||
echo "modelInfo is invalid"
|
||||
exit 1
|
||||
|
@ -7,6 +7,7 @@ import struct
|
||||
from functools import lru_cache
|
||||
from itertools import accumulate
|
||||
|
||||
|
||||
def print_rank_0(*message):
|
||||
pass
|
||||
# """If distributed is initialized print only on rank 0."""
|
||||
@ -16,12 +17,14 @@ def print_rank_0(*message):
|
||||
# else:
|
||||
# print(*message, flush=True)
|
||||
|
||||
|
||||
def _warmup_mmap_file(path):
|
||||
pass
|
||||
# with open(path, "rb") as stream:
|
||||
# while stream.read(100 * 1024 * 1024):
|
||||
# pass
|
||||
|
||||
|
||||
dtypes = {
|
||||
1: np.uint8,
|
||||
2: np.int8,
|
||||
@ -33,18 +36,22 @@ dtypes = {
|
||||
8: np.uint16,
|
||||
}
|
||||
|
||||
|
||||
def code(dtype):
|
||||
for k in dtypes.keys():
|
||||
if dtypes[k] == dtype:
|
||||
return k
|
||||
raise ValueError(dtype)
|
||||
|
||||
|
||||
def index_file_path(prefix_path):
|
||||
return prefix_path + ".idx"
|
||||
|
||||
|
||||
def data_file_path(prefix_path):
|
||||
return prefix_path + ".bin"
|
||||
|
||||
|
||||
class MMapIndexedDataset(torch.utils.data.Dataset):
|
||||
class Index(object):
|
||||
_HDR_MAGIC = b"MMIDIDX\x00\x00"
|
||||
@ -100,7 +107,7 @@ class MMapIndexedDataset(torch.utils.data.Dataset):
|
||||
self._file.close()
|
||||
|
||||
return _Writer()
|
||||
|
||||
|
||||
def __init__(self, path, skip_warmup=False):
|
||||
with open(path, "rb") as stream:
|
||||
magic_test = stream.read(9)
|
||||
@ -217,8 +224,7 @@ class MMapIndexedDataset(torch.utils.data.Dataset):
|
||||
elif isinstance(idx, slice):
|
||||
start, stop, step = idx.indices(len(self))
|
||||
if step != 1:
|
||||
raise ValueError(
|
||||
"Slices into indexed_dataset must be contiguous")
|
||||
raise ValueError("Slices into indexed_dataset must be contiguous")
|
||||
ptr = self._index._pointers[start]
|
||||
sizes = self._index._sizes[idx]
|
||||
offsets = list(accumulate(sizes))
|
@ -17,9 +17,11 @@ class MyDataset(Dataset):
|
||||
|
||||
if args.data_type == "binidx":
|
||||
self.vocab_size = args.vocab_size
|
||||
rank_zero_info(f"Current vocab size = {self.vocab_size} (make sure it's correct)")
|
||||
rank_zero_info(
|
||||
f"Current vocab size = {self.vocab_size} (make sure it's correct)"
|
||||
)
|
||||
|
||||
if args.data_file.endswith('/'):
|
||||
if args.data_file.endswith("/"):
|
||||
d_all = []
|
||||
for p in os.listdir(args.data_file):
|
||||
if p.endswith(".idx"):
|
||||
@ -29,33 +31,52 @@ class MyDataset(Dataset):
|
||||
exit(0)
|
||||
else:
|
||||
self.data = MMapIndexedDataset(args.data_file)
|
||||
self.data_size = len(self.data._bin_buffer) // self.data._index._dtype_size
|
||||
self.data_size = (
|
||||
len(self.data._bin_buffer) // self.data._index._dtype_size
|
||||
)
|
||||
rank_zero_info(f"Data has {self.data_size} tokens.")
|
||||
|
||||
if args.my_qa_mask > 0:
|
||||
self.data_pile = MMapIndexedDataset('/fsx/BlinkDL/pile/pile_20B_tokenizer_text_document')
|
||||
self.data_pile_size = len(self.data_pile._bin_buffer) // self.data._index._dtype_size
|
||||
self.data_pile = MMapIndexedDataset(
|
||||
"/fsx/BlinkDL/pile/pile_20B_tokenizer_text_document"
|
||||
)
|
||||
self.data_pile_size = (
|
||||
len(self.data_pile._bin_buffer) // self.data._index._dtype_size
|
||||
)
|
||||
|
||||
if args.my_pile_stage > 0:
|
||||
# assert self.data_size == 332115325534 and self.vocab_size == 50277
|
||||
self.samples_per_epoch = args.epoch_steps * args.real_bsz
|
||||
assert self.samples_per_epoch == 40320
|
||||
rank_zero_info(f"########## Pile 20b-tokenized stage {args.my_pile_stage} ##########")
|
||||
rank_zero_info(
|
||||
f"########## Pile 20b-tokenized stage {args.my_pile_stage} ##########"
|
||||
)
|
||||
dataset_slot = self.data_size // args.ctx_len
|
||||
if args.my_pile_stage != 4:
|
||||
assert MaybeIsPrime(args.magic_prime)
|
||||
assert args.magic_prime % 3 == 2
|
||||
assert args.magic_prime / dataset_slot > 0.99 and args.magic_prime / dataset_slot <= 1
|
||||
assert (
|
||||
args.magic_prime / dataset_slot > 0.99
|
||||
and args.magic_prime / dataset_slot <= 1
|
||||
)
|
||||
elif args.data_type == "numpy":
|
||||
self.data = np.load(args.data_file).astype("int")
|
||||
self.vocab_size = args.vocab_size
|
||||
rank_zero_info("Current vocab size =", self.vocab_size, "(make sure it's correct)")
|
||||
rank_zero_info(
|
||||
"Current vocab size =", self.vocab_size, "(make sure it's correct)"
|
||||
)
|
||||
self.data_size = len(self.data)
|
||||
rank_zero_info(f"Data has {self.data_size} tokens.")
|
||||
elif args.data_type == "uint16":
|
||||
self.data = np.fromfile(args.data_file, dtype=np.uint16).astype("int32").reshape(-1, args.my_sample_len)
|
||||
self.data = (
|
||||
np.fromfile(args.data_file, dtype=np.uint16)
|
||||
.astype("int32")
|
||||
.reshape(-1, args.my_sample_len)
|
||||
)
|
||||
self.vocab_size = args.vocab_size
|
||||
rank_zero_info("Current vocab size =", self.vocab_size, "(make sure it's correct)")
|
||||
rank_zero_info(
|
||||
"Current vocab size =", self.vocab_size, "(make sure it's correct)"
|
||||
)
|
||||
self.data_size = self.data.shape[0]
|
||||
rank_zero_info(f"Data has {self.data_size} samples.")
|
||||
elif args.data_type == "wds_img":
|
||||
@ -86,10 +107,14 @@ class MyDataset(Dataset):
|
||||
for u in unique:
|
||||
xxObj[xx] = u
|
||||
xx += 1
|
||||
with open(f"{args.proj_dir}/vocab.json", "w", encoding="utf-16le") as vocab_file:
|
||||
with open(
|
||||
f"{args.proj_dir}/vocab.json", "w", encoding="utf-16le"
|
||||
) as vocab_file:
|
||||
vocab_file.write(json.dumps(xxObj, ensure_ascii=False))
|
||||
self.data_size = len(self.data)
|
||||
rank_zero_info(f"Data has {self.data_size} tokens, {self.vocab_size} vocab size.")
|
||||
rank_zero_info(
|
||||
f"Data has {self.data_size} tokens, {self.vocab_size} vocab size."
|
||||
)
|
||||
self.stoi = {ch: i for i, ch in enumerate(unique)}
|
||||
self.itos = {i: ch for i, ch in enumerate(unique)}
|
||||
|
||||
@ -104,36 +129,53 @@ class MyDataset(Dataset):
|
||||
# print(f"epoch {epoch} idx {idx} rank {rank}/{world_size}")
|
||||
|
||||
if args.data_type == "wds_img":
|
||||
|
||||
def init_wds(self, bias=0):
|
||||
def identity(x):
|
||||
return x
|
||||
return x
|
||||
|
||||
import webdataset as wds
|
||||
import torchvision.transforms as transforms
|
||||
|
||||
# img_transform = transforms.Compose(
|
||||
# [transforms.CenterCrop(256)]
|
||||
# )
|
||||
img_transform = transforms.Compose([
|
||||
transforms.CenterCrop(512),
|
||||
transforms.Resize((args.my_img_size))
|
||||
])
|
||||
self.data_raw = wds.WebDataset(args.data_file, resampled=True).shuffle(10000, initial=1000, rng=random.Random(epoch*100000+rank+bias*1e9)).decode("torchrgb").to_tuple("jpg", "json", "txt").map_tuple(img_transform, identity, identity)
|
||||
img_transform = transforms.Compose(
|
||||
[transforms.CenterCrop(512), transforms.Resize((args.my_img_size))]
|
||||
)
|
||||
self.data_raw = (
|
||||
wds.WebDataset(args.data_file, resampled=True)
|
||||
.shuffle(
|
||||
10000,
|
||||
initial=1000,
|
||||
rng=random.Random(epoch * 100000 + rank + bias * 1e9),
|
||||
)
|
||||
.decode("torchrgb")
|
||||
.to_tuple("jpg", "json", "txt")
|
||||
.map_tuple(img_transform, identity, identity)
|
||||
)
|
||||
for pp in self.data_raw.pipeline:
|
||||
if 'Resampled' in str(pp):
|
||||
if "Resampled" in str(pp):
|
||||
pp.deterministic = True
|
||||
|
||||
def worker_seed():
|
||||
return rank*100000+epoch+bias*1e9
|
||||
return rank * 100000 + epoch + bias * 1e9
|
||||
|
||||
pp.worker_seed = worker_seed
|
||||
self.data = iter(self.data_raw)
|
||||
# print(f"WebDataset loaded for rank {rank} epoch {epoch}")
|
||||
|
||||
if self.data == None:
|
||||
init_wds(self)
|
||||
trial = 0
|
||||
while trial < 10:
|
||||
try:
|
||||
dd = next(self.data) # jpg, json, txt
|
||||
dd = next(self.data) # jpg, json, txt
|
||||
break
|
||||
except:
|
||||
print(f'[dataloader error - epoch {epoch} rank {rank} - trying a new shuffle]')
|
||||
print(
|
||||
f"[dataloader error - epoch {epoch} rank {rank} - trying a new shuffle]"
|
||||
)
|
||||
self.error_count += 1
|
||||
init_wds(self, self.error_count)
|
||||
trial += 1
|
||||
@ -144,7 +186,7 @@ class MyDataset(Dataset):
|
||||
return dd[0], dd[2]
|
||||
else:
|
||||
if args.data_type == "uint16":
|
||||
i = np.random.randint(0, self.data_size-1)
|
||||
i = np.random.randint(0, self.data_size - 1)
|
||||
dix = self.data[i]
|
||||
x = torch.tensor(dix[:-1], dtype=torch.long)
|
||||
y = torch.tensor(dix[1:], dtype=torch.long)
|
||||
@ -196,7 +238,12 @@ class MyDataset(Dataset):
|
||||
z_sum = 0
|
||||
isGood = False
|
||||
for i in range(3, ctx_len):
|
||||
if dix[i] == 27 and dix[i-1] == 34 and dix[i-2] == 187 and dix[i-3] == 187:
|
||||
if (
|
||||
dix[i] == 27
|
||||
and dix[i - 1] == 34
|
||||
and dix[i - 2] == 187
|
||||
and dix[i - 3] == 187
|
||||
):
|
||||
isGood = True
|
||||
if dix[i] == 0:
|
||||
isGood = False
|
||||
@ -206,7 +253,9 @@ class MyDataset(Dataset):
|
||||
if z_sum == 0:
|
||||
z = [1] * ctx_len
|
||||
i = np.random.randint(0, self.data_pile_size - req_len)
|
||||
dix = self.data_pile.get(idx=0, offset=i, length=req_len).astype(int)
|
||||
dix = self.data_pile.get(
|
||||
idx=0, offset=i, length=req_len
|
||||
).astype(int)
|
||||
z = torch.tensor(z, dtype=torch.bfloat16)
|
||||
|
||||
x = torch.tensor(dix[:-1], dtype=torch.long)
|
@ -5,6 +5,7 @@
|
||||
import functools
|
||||
import os, math, gc, importlib
|
||||
import torch
|
||||
|
||||
# torch._C._jit_set_profiling_executor(True)
|
||||
# torch._C._jit_set_profiling_mode(True)
|
||||
import torch.nn as nn
|
||||
@ -13,7 +14,8 @@ from torch.nn import functional as F
|
||||
import pytorch_lightning as pl
|
||||
from pytorch_lightning.utilities import rank_zero_info, rank_zero_only
|
||||
from pytorch_lightning.strategies import DeepSpeedStrategy
|
||||
if importlib.util.find_spec('deepspeed'):
|
||||
|
||||
if importlib.util.find_spec("deepspeed"):
|
||||
import deepspeed
|
||||
from deepspeed.ops.adam import DeepSpeedCPUAdam, FusedAdam
|
||||
|
||||
@ -28,9 +30,10 @@ LORA_CONFIG = {
|
||||
|
||||
|
||||
try:
|
||||
print('RWKV_MY_TESTING', os.environ["RWKV_MY_TESTING"])
|
||||
print("RWKV_MY_TESTING", os.environ["RWKV_MY_TESTING"])
|
||||
except:
|
||||
os.environ["RWKV_MY_TESTING"] = ''
|
||||
os.environ["RWKV_MY_TESTING"] = ""
|
||||
|
||||
|
||||
def __nop(ob):
|
||||
return ob
|
||||
@ -53,7 +56,26 @@ T_MAX = int(os.environ["RWKV_T_MAX"]) # TAKES LOTS OF VRAM!
|
||||
from torch.utils.cpp_extension import load
|
||||
|
||||
if os.environ["RWKV_FLOAT_MODE"] == "bf16":
|
||||
wkv_cuda = load(name=f"wkv_{T_MAX}_bf16", sources=["finetune/lora/cuda/wkv_op_bf16.cpp", "finetune/lora/cuda/wkv_cuda_bf16.cu"], verbose=True, extra_cuda_cflags=["-t 4", "-std=c++17", "-res-usage", "--maxrregcount 60", "--use_fast_math", "-O3", "-Xptxas -O3", "--extra-device-vectorization", f"-DTmax={T_MAX}"])
|
||||
wkv_cuda = load(
|
||||
name=f"wkv_{T_MAX}_bf16",
|
||||
sources=[
|
||||
"finetune/lora/v4/cuda/wkv_op_bf16.cpp",
|
||||
"finetune/lora/v4/cuda/wkv_cuda_bf16.cu",
|
||||
],
|
||||
verbose=True,
|
||||
extra_cuda_cflags=[
|
||||
"-t 4",
|
||||
"-std=c++17",
|
||||
"-res-usage",
|
||||
"--maxrregcount 60",
|
||||
"--use_fast_math",
|
||||
"-O3",
|
||||
"-Xptxas -O3",
|
||||
"--extra-device-vectorization",
|
||||
f"-DTmax={T_MAX}",
|
||||
],
|
||||
)
|
||||
|
||||
class WKV(torch.autograd.Function):
|
||||
@staticmethod
|
||||
def forward(ctx, B, T, C, w, u, k, v):
|
||||
@ -66,10 +88,16 @@ if os.environ["RWKV_FLOAT_MODE"] == "bf16":
|
||||
u = u.contiguous()
|
||||
k = k.contiguous()
|
||||
v = v.contiguous()
|
||||
y = torch.empty((B, T, C), device=w.device, memory_format=torch.contiguous_format, dtype=torch.bfloat16)
|
||||
y = torch.empty(
|
||||
(B, T, C),
|
||||
device=w.device,
|
||||
memory_format=torch.contiguous_format,
|
||||
dtype=torch.bfloat16,
|
||||
)
|
||||
wkv_cuda.forward(B, T, C, w, u, k, v, y)
|
||||
ctx.save_for_backward(w, u, k, v, y)
|
||||
return y
|
||||
|
||||
@staticmethod
|
||||
def backward(ctx, gy):
|
||||
B = ctx.B
|
||||
@ -78,16 +106,54 @@ if os.environ["RWKV_FLOAT_MODE"] == "bf16":
|
||||
assert T <= T_MAX
|
||||
assert B * C % min(C, 32) == 0
|
||||
w, u, k, v, y = ctx.saved_tensors
|
||||
gw = torch.empty((B, C), device=gy.device, memory_format=torch.contiguous_format, dtype=torch.bfloat16)
|
||||
gu = torch.empty((B, C), device=gy.device, memory_format=torch.contiguous_format, dtype=torch.bfloat16)
|
||||
gk = torch.empty((B, T, C), device=gy.device, memory_format=torch.contiguous_format, dtype=torch.bfloat16)
|
||||
gv = torch.empty((B, T, C), device=gy.device, memory_format=torch.contiguous_format, dtype=torch.bfloat16)
|
||||
gw = torch.empty(
|
||||
(B, C),
|
||||
device=gy.device,
|
||||
memory_format=torch.contiguous_format,
|
||||
dtype=torch.bfloat16,
|
||||
)
|
||||
gu = torch.empty(
|
||||
(B, C),
|
||||
device=gy.device,
|
||||
memory_format=torch.contiguous_format,
|
||||
dtype=torch.bfloat16,
|
||||
)
|
||||
gk = torch.empty(
|
||||
(B, T, C),
|
||||
device=gy.device,
|
||||
memory_format=torch.contiguous_format,
|
||||
dtype=torch.bfloat16,
|
||||
)
|
||||
gv = torch.empty(
|
||||
(B, T, C),
|
||||
device=gy.device,
|
||||
memory_format=torch.contiguous_format,
|
||||
dtype=torch.bfloat16,
|
||||
)
|
||||
wkv_cuda.backward(B, T, C, w, u, k, v, y, gy.contiguous(), gw, gu, gk, gv)
|
||||
gw = torch.sum(gw, dim=0)
|
||||
gu = torch.sum(gu, dim=0)
|
||||
return (None, None, None, gw, gu, gk, gv)
|
||||
|
||||
else:
|
||||
wkv_cuda = load(name=f"wkv_{T_MAX}", sources=["finetune/lora/cuda/wkv_op.cpp", "finetune/lora/cuda/wkv_cuda.cu"], verbose=True, extra_cuda_cflags=["-res-usage", "--maxrregcount 60", "--use_fast_math", "-O3", "-Xptxas -O3", "--extra-device-vectorization", f"-DTmax={T_MAX}"])
|
||||
wkv_cuda = load(
|
||||
name=f"wkv_{T_MAX}",
|
||||
sources=[
|
||||
"finetune/lora/v4/cuda/wkv_op.cpp",
|
||||
"finetune/lora/v4/cuda/wkv_cuda.cu",
|
||||
],
|
||||
verbose=True,
|
||||
extra_cuda_cflags=[
|
||||
"-res-usage",
|
||||
"--maxrregcount 60",
|
||||
"--use_fast_math",
|
||||
"-O3",
|
||||
"-Xptxas -O3",
|
||||
"--extra-device-vectorization",
|
||||
f"-DTmax={T_MAX}",
|
||||
],
|
||||
)
|
||||
|
||||
class WKV(torch.autograd.Function):
|
||||
@staticmethod
|
||||
def forward(ctx, B, T, C, w, u, k, v):
|
||||
@ -106,7 +172,9 @@ else:
|
||||
u = u.float().contiguous()
|
||||
k = k.float().contiguous()
|
||||
v = v.float().contiguous()
|
||||
y = torch.empty((B, T, C), device=w.device, memory_format=torch.contiguous_format)
|
||||
y = torch.empty(
|
||||
(B, T, C), device=w.device, memory_format=torch.contiguous_format
|
||||
)
|
||||
wkv_cuda.forward(B, T, C, w, u, k, v, y)
|
||||
ctx.save_for_backward(w, u, k, v, y)
|
||||
if "32" in os.environ["RWKV_FLOAT_MODE"]:
|
||||
@ -115,6 +183,7 @@ else:
|
||||
return y.half()
|
||||
elif os.environ["RWKV_FLOAT_MODE"] == "bf16":
|
||||
return y.bfloat16()
|
||||
|
||||
@staticmethod
|
||||
def backward(ctx, gy):
|
||||
B = ctx.B
|
||||
@ -123,14 +192,26 @@ else:
|
||||
assert T <= T_MAX
|
||||
assert B * C % min(C, 32) == 0
|
||||
w, u, k, v, y = ctx.saved_tensors
|
||||
gw = torch.empty((B, C), device=gy.device, memory_format=torch.contiguous_format)
|
||||
gu = torch.empty((B, C), device=gy.device, memory_format=torch.contiguous_format)
|
||||
gk = torch.empty((B, T, C), device=gy.device, memory_format=torch.contiguous_format)
|
||||
gv = torch.empty((B, T, C), device=gy.device, memory_format=torch.contiguous_format)
|
||||
gw = torch.empty(
|
||||
(B, C), device=gy.device, memory_format=torch.contiguous_format
|
||||
)
|
||||
gu = torch.empty(
|
||||
(B, C), device=gy.device, memory_format=torch.contiguous_format
|
||||
)
|
||||
gk = torch.empty(
|
||||
(B, T, C), device=gy.device, memory_format=torch.contiguous_format
|
||||
)
|
||||
gv = torch.empty(
|
||||
(B, T, C), device=gy.device, memory_format=torch.contiguous_format
|
||||
)
|
||||
if "32" in os.environ["RWKV_FLOAT_MODE"]:
|
||||
wkv_cuda.backward(B, T, C, w, u, k, v, y, gy.contiguous(), gw, gu, gk, gv)
|
||||
wkv_cuda.backward(
|
||||
B, T, C, w, u, k, v, y, gy.contiguous(), gw, gu, gk, gv
|
||||
)
|
||||
else:
|
||||
wkv_cuda.backward(B, T, C, w, u, k, v, y, gy.float().contiguous(), gw, gu, gk, gv)
|
||||
wkv_cuda.backward(
|
||||
B, T, C, w, u, k, v, y, gy.float().contiguous(), gw, gu, gk, gv
|
||||
)
|
||||
gw = torch.sum(gw, dim=0)
|
||||
gu = torch.sum(gu, dim=0)
|
||||
if "32" in os.environ["RWKV_FLOAT_MODE"]:
|
||||
@ -138,7 +219,15 @@ else:
|
||||
elif os.environ["RWKV_FLOAT_MODE"] == "fp16":
|
||||
return (None, None, None, gw.half(), gu.half(), gk.half(), gv.half())
|
||||
elif os.environ["RWKV_FLOAT_MODE"] == "bf16":
|
||||
return (None, None, None, gw.bfloat16(), gu.bfloat16(), gk.bfloat16(), gv.bfloat16())
|
||||
return (
|
||||
None,
|
||||
None,
|
||||
None,
|
||||
gw.bfloat16(),
|
||||
gu.bfloat16(),
|
||||
gk.bfloat16(),
|
||||
gv.bfloat16(),
|
||||
)
|
||||
|
||||
|
||||
def RUN_CUDA(B, T, C, w, u, k, v):
|
||||
@ -151,15 +240,17 @@ def RUN_CUDA(B, T, C, w, u, k, v):
|
||||
|
||||
|
||||
class LoraLinear(nn.Module):
|
||||
|
||||
def __init__(self, in_features: int, out_features: int, bias: bool):
|
||||
super().__init__()
|
||||
|
||||
self.weight = nn.Parameter(torch.empty((out_features, in_features)))
|
||||
assert bias == False, "Biased LoraLinear not supported"
|
||||
|
||||
r, alpha, dropout = LORA_CONFIG["r"], LORA_CONFIG[
|
||||
"alpha"], LORA_CONFIG["dropout"]
|
||||
r, alpha, dropout = (
|
||||
LORA_CONFIG["r"],
|
||||
LORA_CONFIG["alpha"],
|
||||
LORA_CONFIG["dropout"],
|
||||
)
|
||||
self.lora_A = nn.Parameter(torch.empty(r, in_features))
|
||||
self.lora_B = nn.Parameter(torch.empty(out_features, r))
|
||||
self.lora_dropout = nn.Dropout(dropout)
|
||||
@ -170,9 +261,9 @@ class LoraLinear(nn.Module):
|
||||
nn.init.zeros_(self.lora_B)
|
||||
|
||||
def forward(self, x):
|
||||
return (
|
||||
F.linear(x, self.weight) + self.scaling *
|
||||
F.linear(F.linear(self.lora_dropout(x), self.lora_A), self.lora_B))
|
||||
return F.linear(x, self.weight) + self.scaling * F.linear(
|
||||
F.linear(self.lora_dropout(x), self.lora_A), self.lora_B
|
||||
)
|
||||
|
||||
|
||||
@functools.wraps(LoraLinear)
|
||||
@ -214,17 +305,23 @@ class RWKV_TimeMix(MyModule):
|
||||
# fancy time_decay
|
||||
decay_speed = torch.ones(args.dim_att)
|
||||
for h in range(args.dim_att):
|
||||
decay_speed[h] = -5 + 8 * (h / (args.dim_att - 1)) ** (0.7 + 1.3 * ratio_0_to_1)
|
||||
decay_speed[h] = -5 + 8 * (h / (args.dim_att - 1)) ** (
|
||||
0.7 + 1.3 * ratio_0_to_1
|
||||
)
|
||||
self.time_decay = nn.Parameter(decay_speed)
|
||||
# print(layer_id, self.time_decay.flatten()[:3].cpu().numpy(), '...', self.time_decay.flatten()[-3:].cpu().numpy())
|
||||
|
||||
# fancy time_first
|
||||
zigzag = torch.tensor([(i + 1) % 3 - 1 for i in range(args.dim_att)]) * 0.5
|
||||
self.time_first = nn.Parameter(torch.ones(args.dim_att) * math.log(0.3) + zigzag)
|
||||
self.time_first = nn.Parameter(
|
||||
torch.ones(args.dim_att) * math.log(0.3) + zigzag
|
||||
)
|
||||
|
||||
# fancy time_mix
|
||||
self.time_mix_k = nn.Parameter(torch.pow(ddd, ratio_1_to_almost0))
|
||||
self.time_mix_v = nn.Parameter(torch.pow(ddd, ratio_1_to_almost0) + 0.3 * ratio_0_to_1)
|
||||
self.time_mix_v = nn.Parameter(
|
||||
torch.pow(ddd, ratio_1_to_almost0) + 0.3 * ratio_0_to_1
|
||||
)
|
||||
self.time_mix_r = nn.Parameter(torch.pow(ddd, 0.5 * ratio_1_to_almost0))
|
||||
|
||||
self.time_shift = nn.ZeroPad2d((0, 0, 1, -1))
|
||||
@ -235,8 +332,10 @@ class RWKV_TimeMix(MyModule):
|
||||
|
||||
self.output = nn.Linear(args.dim_att, args.n_embd, bias=False)
|
||||
|
||||
if 'a' in os.environ["RWKV_MY_TESTING"]:
|
||||
self.register_buffer("att_mask", torch.tril(torch.ones(args.ctx_len, args.ctx_len)))
|
||||
if "a" in os.environ["RWKV_MY_TESTING"]:
|
||||
self.register_buffer(
|
||||
"att_mask", torch.tril(torch.ones(args.ctx_len, args.ctx_len))
|
||||
)
|
||||
d_qkv = args.n_embd // 16
|
||||
self.qq = nn.Linear(args.n_embd, d_qkv, bias=False)
|
||||
self.kk = nn.Linear(args.n_embd, d_qkv, bias=False)
|
||||
@ -245,12 +344,17 @@ class RWKV_TimeMix(MyModule):
|
||||
with torch.no_grad():
|
||||
self.time_mix_qq = nn.Parameter(torch.pow(ddd, ratio_1_to_almost0))
|
||||
self.time_mix_kk = nn.Parameter(torch.pow(ddd, ratio_1_to_almost0))
|
||||
self.time_mix_vv = nn.Parameter(torch.pow(ddd, ratio_1_to_almost0) + 0.3 * ratio_0_to_1)
|
||||
self.time_mix_vv = nn.Parameter(
|
||||
torch.pow(ddd, ratio_1_to_almost0) + 0.3 * ratio_0_to_1
|
||||
)
|
||||
|
||||
if "a" not in os.environ["RWKV_MY_TESTING"]:
|
||||
|
||||
if 'a' not in os.environ["RWKV_MY_TESTING"]:
|
||||
@MyFunction
|
||||
def jit_func(self, x):
|
||||
xx = self.time_shift(x) # Mix x with the previous timestep to produce xk, xv, xr
|
||||
xx = self.time_shift(
|
||||
x
|
||||
) # Mix x with the previous timestep to produce xk, xv, xr
|
||||
xk = x * self.time_mix_k + xx * (1 - self.time_mix_k)
|
||||
xv = x * self.time_mix_v + xx * (1 - self.time_mix_v)
|
||||
xr = x * self.time_mix_r + xx * (1 - self.time_mix_r)
|
||||
@ -263,21 +367,26 @@ class RWKV_TimeMix(MyModule):
|
||||
def forward(self, x):
|
||||
B, T, C = x.size() # x = (Batch,Time,Channel)
|
||||
sr, k, v = self.jit_func(x)
|
||||
rwkv = sr * RUN_CUDA(B, T, self.args.dim_att, self.time_decay, self.time_first, k, v)
|
||||
rwkv = sr * RUN_CUDA(
|
||||
B, T, self.args.dim_att, self.time_decay, self.time_first, k, v
|
||||
)
|
||||
return self.output(rwkv)
|
||||
|
||||
if 'a' in os.environ["RWKV_MY_TESTING"]:
|
||||
if "a" in os.environ["RWKV_MY_TESTING"]:
|
||||
|
||||
@MyFunction
|
||||
def QKV(self, q, k, v):
|
||||
att = (q @ k.transpose(-2, -1)) * (1.0 / math.sqrt(k.size(-1)))
|
||||
att = att.masked_fill(self.att_mask == 0, float('-inf'))
|
||||
att = F.softmax(att, dim = -1)
|
||||
att = att.masked_fill(self.att_mask == 0, float("-inf"))
|
||||
att = F.softmax(att, dim=-1)
|
||||
x = att @ v
|
||||
return x
|
||||
|
||||
@MyFunction
|
||||
def jit_funcQKV(self, x):
|
||||
xx = self.time_shift(x) # Mix x with the previous timestep to produce xk, xv, xr
|
||||
xx = self.time_shift(
|
||||
x
|
||||
) # Mix x with the previous timestep to produce xk, xv, xr
|
||||
xk = x * self.time_mix_k + xx * (1 - self.time_mix_k)
|
||||
xv = x * self.time_mix_v + xx * (1 - self.time_mix_v)
|
||||
xr = x * self.time_mix_r + xx * (1 - self.time_mix_r)
|
||||
@ -296,12 +405,16 @@ class RWKV_TimeMix(MyModule):
|
||||
def forward(self, x):
|
||||
B, T, C = x.size() # x = (Batch,Time,Channel)
|
||||
sr, k, v, qq, kk, vv = self.jit_funcQKV(x)
|
||||
rwkv = sr * RUN_CUDA(B, T, self.args.dim_att, self.time_decay, self.time_first, k, v)
|
||||
rwkv = sr * RUN_CUDA(
|
||||
B, T, self.args.dim_att, self.time_decay, self.time_first, k, v
|
||||
)
|
||||
rwkv = self.output(rwkv) + self.oo(self.QKV(qq, kk, vv))
|
||||
return rwkv
|
||||
|
||||
|
||||
########################################################################################################
|
||||
|
||||
|
||||
class RWKV_ChannelMix(MyModule):
|
||||
def __init__(self, args, layer_id):
|
||||
super().__init__()
|
||||
@ -331,6 +444,7 @@ class RWKV_ChannelMix(MyModule):
|
||||
kv = self.value(k)
|
||||
return torch.sigmoid(self.receptance(xr)) * kv
|
||||
|
||||
|
||||
class MishGLU(MyModule):
|
||||
def __init__(self, args, layer_id):
|
||||
super().__init__()
|
||||
@ -360,6 +474,7 @@ class MishGLU(MyModule):
|
||||
b = self.bb(xb)
|
||||
return self.value(a * F.mish(b))
|
||||
|
||||
|
||||
########################################################################################################
|
||||
# The RWKV Model with our blocks
|
||||
########################################################################################################
|
||||
@ -377,15 +492,19 @@ class Block(nn.Module):
|
||||
if self.layer_id == 0:
|
||||
self.ln0 = nn.LayerNorm(args.n_embd)
|
||||
if args.my_pos_emb > 0:
|
||||
self.pos_emb_x = nn.Parameter(torch.zeros((1,args.my_pos_emb,args.n_embd)))
|
||||
self.pos_emb_y = nn.Parameter(torch.zeros((args.my_pos_emb,1,args.n_embd)))
|
||||
self.pos_emb_x = nn.Parameter(
|
||||
torch.zeros((1, args.my_pos_emb, args.n_embd))
|
||||
)
|
||||
self.pos_emb_y = nn.Parameter(
|
||||
torch.zeros((args.my_pos_emb, 1, args.n_embd))
|
||||
)
|
||||
|
||||
if self.layer_id == 0 and self.args.pre_ffn > 0:
|
||||
self.ffnPre = RWKV_ChannelMix(args, 0)
|
||||
else:
|
||||
self.att = RWKV_TimeMix(args, layer_id)
|
||||
|
||||
if 'g' in os.environ["RWKV_MY_TESTING"]:
|
||||
if "g" in os.environ["RWKV_MY_TESTING"]:
|
||||
self.ffn = MishGLU(args, layer_id)
|
||||
else:
|
||||
self.ffn = RWKV_ChannelMix(args, layer_id)
|
||||
@ -395,7 +514,9 @@ class Block(nn.Module):
|
||||
self.tiny_q = nn.Linear(args.n_embd, args.tiny_att_dim, bias=False)
|
||||
self.tiny_k = nn.Linear(args.n_embd, args.tiny_att_dim, bias=False)
|
||||
self.tiny_v = nn.Linear(args.n_embd, args.n_embd, bias=False)
|
||||
self.register_buffer("tiny_mask", torch.tril(torch.ones(args.ctx_len, args.ctx_len)))
|
||||
self.register_buffer(
|
||||
"tiny_mask", torch.tril(torch.ones(args.ctx_len, args.ctx_len))
|
||||
)
|
||||
|
||||
def forward(self, x, x_emb=None):
|
||||
args = self.args
|
||||
@ -403,7 +524,7 @@ class Block(nn.Module):
|
||||
if self.layer_id == 0:
|
||||
x = self.ln0(x)
|
||||
if args.my_pos_emb > 0:
|
||||
pos_emb = (self.pos_emb_x + self.pos_emb_y).reshape(T+1, -1)[:-1,:]
|
||||
pos_emb = (self.pos_emb_x + self.pos_emb_y).reshape(T + 1, -1)[:-1, :]
|
||||
x = x + pos_emb
|
||||
|
||||
if self.layer_id == 0 and args.pre_ffn > 0:
|
||||
@ -443,13 +564,13 @@ class RWKV(pl.LightningModule):
|
||||
def __init__(self, args):
|
||||
super().__init__()
|
||||
self.args = args
|
||||
if not hasattr(args, 'dim_att'):
|
||||
if not hasattr(args, "dim_att"):
|
||||
args.dim_att = args.n_embd
|
||||
if not hasattr(args, 'dim_ffn'):
|
||||
if not hasattr(args, "dim_ffn"):
|
||||
args.dim_ffn = args.n_embd * 4
|
||||
if not hasattr(args, 'tiny_att_layer'):
|
||||
if not hasattr(args, "tiny_att_layer"):
|
||||
args.tiny_att_layer = -1
|
||||
if not hasattr(args, 'tiny_att_dim'):
|
||||
if not hasattr(args, "tiny_att_dim"):
|
||||
args.tiny_att_dim = -1
|
||||
|
||||
self.emb = nn.Embedding(args.vocab_size, args.n_embd)
|
||||
@ -462,7 +583,9 @@ class RWKV(pl.LightningModule):
|
||||
if args.head_qk > 0:
|
||||
self.head_q = nn.Linear(args.n_embd, args.head_qk, bias=False)
|
||||
self.head_k = nn.Linear(args.n_embd, args.head_qk, bias=False)
|
||||
self.register_buffer("copy_mask", torch.tril(torch.ones(args.ctx_len, args.ctx_len)))
|
||||
self.register_buffer(
|
||||
"copy_mask", torch.tril(torch.ones(args.ctx_len, args.ctx_len))
|
||||
)
|
||||
|
||||
def configure_optimizers(self):
|
||||
args = self.args
|
||||
@ -494,19 +617,46 @@ class RWKV(pl.LightningModule):
|
||||
param_dict = {n: p for n, p in self.named_parameters()}
|
||||
if args.my_pile_stage == 2:
|
||||
optim_groups = [
|
||||
{"params": [param_dict[n] for n in lr_1x], "weight_decay": 0.0, "my_lr_scale": 1.0},
|
||||
{"params": [param_dict[n] for n in lr_2x], "weight_decay": 0.0, "my_lr_scale": 5.0},# test: 2e-3 / args.lr_init},
|
||||
{"params": [param_dict[n] for n in lr_3x], "weight_decay": 0.0, "my_lr_scale": 5.0},# test: 3e-3 / args.lr_init},
|
||||
{
|
||||
"params": [param_dict[n] for n in lr_1x],
|
||||
"weight_decay": 0.0,
|
||||
"my_lr_scale": 1.0,
|
||||
},
|
||||
{
|
||||
"params": [param_dict[n] for n in lr_2x],
|
||||
"weight_decay": 0.0,
|
||||
"my_lr_scale": 5.0,
|
||||
}, # test: 2e-3 / args.lr_init},
|
||||
{
|
||||
"params": [param_dict[n] for n in lr_3x],
|
||||
"weight_decay": 0.0,
|
||||
"my_lr_scale": 5.0,
|
||||
}, # test: 3e-3 / args.lr_init},
|
||||
]
|
||||
else:
|
||||
optim_groups = [
|
||||
{"params": [param_dict[n] for n in lr_1x], "weight_decay": 0.0, "my_lr_scale": 1.0},
|
||||
{"params": [param_dict[n] for n in lr_2x], "weight_decay": 0.0, "my_lr_scale": 2.0},
|
||||
{"params": [param_dict[n] for n in lr_3x], "weight_decay": 0.0, "my_lr_scale": 3.0},
|
||||
{
|
||||
"params": [param_dict[n] for n in lr_1x],
|
||||
"weight_decay": 0.0,
|
||||
"my_lr_scale": 1.0,
|
||||
},
|
||||
{
|
||||
"params": [param_dict[n] for n in lr_2x],
|
||||
"weight_decay": 0.0,
|
||||
"my_lr_scale": 2.0,
|
||||
},
|
||||
{
|
||||
"params": [param_dict[n] for n in lr_3x],
|
||||
"weight_decay": 0.0,
|
||||
"my_lr_scale": 3.0,
|
||||
},
|
||||
]
|
||||
else:
|
||||
optim_groups = [
|
||||
{"params": [p for n, p in self.named_parameters()], "weight_decay": 0.0},
|
||||
{
|
||||
"params": [p for n, p in self.named_parameters()],
|
||||
"weight_decay": 0.0,
|
||||
},
|
||||
]
|
||||
|
||||
for g in optim_groups:
|
||||
@ -514,8 +664,26 @@ class RWKV(pl.LightningModule):
|
||||
optim_groups = [g for g in optim_groups if len(g["params"]) > 0]
|
||||
|
||||
if self.deepspeed_offload:
|
||||
return DeepSpeedCPUAdam(optim_groups, lr=self.args.lr_init, betas=self.args.betas, eps=self.args.adam_eps, bias_correction=True, adamw_mode=False, weight_decay=0, amsgrad=False)
|
||||
return FusedAdam(optim_groups, lr=self.args.lr_init, betas=self.args.betas, eps=self.args.adam_eps, bias_correction=True, adam_w_mode=False, weight_decay=0, amsgrad=False)
|
||||
return DeepSpeedCPUAdam(
|
||||
optim_groups,
|
||||
lr=self.args.lr_init,
|
||||
betas=self.args.betas,
|
||||
eps=self.args.adam_eps,
|
||||
bias_correction=True,
|
||||
adamw_mode=False,
|
||||
weight_decay=0,
|
||||
amsgrad=False,
|
||||
)
|
||||
return FusedAdam(
|
||||
optim_groups,
|
||||
lr=self.args.lr_init,
|
||||
betas=self.args.betas,
|
||||
eps=self.args.adam_eps,
|
||||
bias_correction=True,
|
||||
adam_w_mode=False,
|
||||
weight_decay=0,
|
||||
amsgrad=False,
|
||||
)
|
||||
# return ZeroOneAdam(optim_groups, lr=self.args.lr_init, betas=self.args.betas, eps=self.args.adam_eps, bias_correction=True, weight_decay=0, amsgrad=False, cuda_aware=False)
|
||||
|
||||
@property
|
||||
@ -589,10 +757,14 @@ class RWKV(pl.LightningModule):
|
||||
|
||||
logits = self(idx)
|
||||
if sum_mask == mask.shape[0]:
|
||||
loss = F.cross_entropy(logits.view(-1, logits.size(-1)), targets.view(-1))
|
||||
loss = F.cross_entropy(
|
||||
logits.view(-1, logits.size(-1)), targets.view(-1)
|
||||
)
|
||||
# print('rank', self.global_rank, 'loss', loss.item())
|
||||
else:
|
||||
loss = F.cross_entropy(logits.view(-1, logits.size(-1)), targets.view(-1), reduction='none')
|
||||
loss = F.cross_entropy(
|
||||
logits.view(-1, logits.size(-1)), targets.view(-1), reduction="none"
|
||||
)
|
||||
# loss_raw = loss
|
||||
loss = torch.sum(loss * mask) / sum_mask
|
||||
|
||||
@ -632,7 +804,14 @@ class RWKV(pl.LightningModule):
|
||||
|
||||
gain = 1.0
|
||||
scale = 1.0
|
||||
if "ln_" in n or ".ln" in n or "time_" in n or "_mask" in n or "pos_emb" in n or '.mask.' in n:
|
||||
if (
|
||||
"ln_" in n
|
||||
or ".ln" in n
|
||||
or "time_" in n
|
||||
or "_mask" in n
|
||||
or "pos_emb" in n
|
||||
or ".mask." in n
|
||||
):
|
||||
m[n] = p
|
||||
else:
|
||||
if n == "emb.weight":
|
||||
@ -640,7 +819,19 @@ class RWKV(pl.LightningModule):
|
||||
else:
|
||||
if shape[0] > shape[1]:
|
||||
gain = math.sqrt(shape[0] / shape[1])
|
||||
for kk in [".att.key.", ".att.receptance.", ".att.output.", ".att.key.", ".ffn.value.", ".ffn.receptance.", ".ffnPre.value.", ".ffnPre.receptance.", "head_q.", '.oo.', '.rr.']:
|
||||
for kk in [
|
||||
".att.key.",
|
||||
".att.receptance.",
|
||||
".att.output.",
|
||||
".att.key.",
|
||||
".ffn.value.",
|
||||
".ffn.receptance.",
|
||||
".ffnPre.value.",
|
||||
".ffnPre.receptance.",
|
||||
"head_q.",
|
||||
".oo.",
|
||||
".rr.",
|
||||
]:
|
||||
if kk in n:
|
||||
scale = 0
|
||||
if n == "head.weight":
|
||||
@ -650,7 +841,9 @@ class RWKV(pl.LightningModule):
|
||||
if "head_q." in n:
|
||||
scale = 0
|
||||
|
||||
print(f"{str(shape[0]).ljust(5)} {str(shape[1]).ljust(5)} {str(scale).ljust(4)} {n}")
|
||||
print(
|
||||
f"{str(shape[0]).ljust(5)} {str(shape[1]).ljust(5)} {str(scale).ljust(4)} {n}"
|
||||
)
|
||||
|
||||
if self.args.accelerator.upper() == "GPU":
|
||||
m[n] = torch.empty((shape[0], shape[1]), device="cuda")
|
@ -5,15 +5,17 @@ import pytorch_lightning as pl
|
||||
from pytorch_lightning.utilities import rank_zero_info, rank_zero_only
|
||||
from .model import LORA_CONFIG
|
||||
|
||||
|
||||
def my_save(dd, ff):
|
||||
if '14b-run1' not in ff:
|
||||
if "14b-run1" not in ff:
|
||||
torch.save(dd, ff)
|
||||
else:
|
||||
fn = ff.split('/')[-1]
|
||||
fff = '/dev/shm/' + fn
|
||||
fn = ff.split("/")[-1]
|
||||
fff = "/dev/shm/" + fn
|
||||
torch.save(dd, fff)
|
||||
subprocess.Popen(f" aws s3 mv {fff} s3://rwkv-14b-4k/{fn} --quiet", shell=True)
|
||||
|
||||
|
||||
class train_callback(pl.Callback):
|
||||
def __init__(self, args):
|
||||
super().__init__()
|
||||
@ -38,7 +40,9 @@ class train_callback(pl.Callback):
|
||||
if args.lr_final == 0 or args.lr_init == 0: # linear decay
|
||||
lr = args.lr_init + (args.lr_final - args.lr_init) * progress
|
||||
else: # exp decay
|
||||
lr = args.lr_init * math.exp(math.log(args.lr_final / args.lr_init) * pow(progress, 1))
|
||||
lr = args.lr_init * math.exp(
|
||||
math.log(args.lr_final / args.lr_init) * pow(progress, 1)
|
||||
)
|
||||
|
||||
if trainer.global_step < w_step:
|
||||
lr = lr * (0.2 + 0.8 * trainer.global_step / w_step)
|
||||
@ -60,7 +64,9 @@ class train_callback(pl.Callback):
|
||||
trainer.my_loss_sum = 0
|
||||
trainer.my_loss_count = 0
|
||||
trainer.my_log = open(args.proj_dir + "/train_log.txt", "a")
|
||||
trainer.my_log.write(f"NEW RUN {args.my_timestamp}\n{vars(self.args)}\n")
|
||||
trainer.my_log.write(
|
||||
f"NEW RUN {args.my_timestamp}\n{vars(self.args)}\n"
|
||||
)
|
||||
try:
|
||||
print(f"\n{trainer.strategy.config}\n")
|
||||
trainer.my_log.write(f"{trainer.strategy.config}\n")
|
||||
@ -70,6 +76,7 @@ class train_callback(pl.Callback):
|
||||
if len(args.wandb) > 0:
|
||||
print("Login to wandb...")
|
||||
import wandb
|
||||
|
||||
wandb.init(
|
||||
project=args.wandb,
|
||||
name=args.run_name + " " + args.my_timestamp,
|
||||
@ -102,20 +109,26 @@ class train_callback(pl.Callback):
|
||||
# self.log("s", real_step, prog_bar=True, on_step=True)
|
||||
|
||||
if len(args.wandb) > 0:
|
||||
lll = {"loss": trainer.my_loss, "lr": trainer.my_lr, "Gtokens": real_step * token_per_step / 1e9}
|
||||
lll = {
|
||||
"loss": trainer.my_loss,
|
||||
"lr": trainer.my_lr,
|
||||
"Gtokens": real_step * token_per_step / 1e9,
|
||||
}
|
||||
if kt_s > 0:
|
||||
lll["kt/s"] = kt_s
|
||||
trainer.my_wandb.log(lll, step=int(real_step))
|
||||
if args.magic_prime > 0:
|
||||
expand_factor = 2 if args.my_qa_mask > 0 else 1
|
||||
if int(real_step) == int(args.magic_prime * expand_factor // args.real_bsz) - 1:
|
||||
if (
|
||||
int(real_step)
|
||||
== int(args.magic_prime * expand_factor // args.real_bsz) - 1
|
||||
):
|
||||
to_save_dict = pl_module.state_dict()
|
||||
my_save(
|
||||
to_save_dict,
|
||||
f"{args.proj_dir}/rwkv-final.pth",
|
||||
)
|
||||
|
||||
|
||||
def on_train_epoch_start(self, trainer, pl_module):
|
||||
args = self.args
|
||||
dataset = trainer.train_dataloader.dataset.datasets
|
||||
@ -128,24 +141,28 @@ class train_callback(pl.Callback):
|
||||
def on_train_epoch_end(self, trainer, pl_module):
|
||||
args = self.args
|
||||
if trainer.is_global_zero: # logging & save state_dict
|
||||
if (args.epoch_save > 0 and trainer.current_epoch % args.epoch_save == 0) or trainer.current_epoch == args.epoch_count - 1:
|
||||
if args.data_type == 'wds_img':
|
||||
if (
|
||||
args.epoch_save > 0 and trainer.current_epoch % args.epoch_save == 0
|
||||
) or trainer.current_epoch == args.epoch_count - 1:
|
||||
if args.data_type == "wds_img":
|
||||
raw_dict = pl_module.state_dict()
|
||||
to_save_dict = {}
|
||||
for k in raw_dict:
|
||||
if k.startswith('encoder.') or k.startswith('decoder.'):
|
||||
if k.startswith("encoder.") or k.startswith("decoder."):
|
||||
to_save_dict[k] = raw_dict[k]
|
||||
else:
|
||||
to_save_dict = pl_module.state_dict()
|
||||
|
||||
if args.lora:
|
||||
enable_time_finetune = 'time' in LORA_CONFIG["parts"]
|
||||
enable_ln_finetune = 'ln' in LORA_CONFIG["parts"]
|
||||
enable_time_finetune = "time" in LORA_CONFIG["parts"]
|
||||
enable_ln_finetune = "ln" in LORA_CONFIG["parts"]
|
||||
lora_dict = {}
|
||||
for name, state in to_save_dict.items():
|
||||
if ('.lora_' in name
|
||||
or (enable_time_finetune and '.time_' in name)
|
||||
or (enable_ln_finetune and '.ln' in name)):
|
||||
if (
|
||||
".lora_" in name
|
||||
or (enable_time_finetune and ".time_" in name)
|
||||
or (enable_ln_finetune and ".ln" in name)
|
||||
):
|
||||
lora_dict[name] = state
|
||||
to_save_dict = lora_dict
|
||||
|
||||
@ -155,8 +172,10 @@ class train_callback(pl.Callback):
|
||||
f"{args.proj_dir}/rwkv-{args.epoch_begin + trainer.current_epoch}.pth",
|
||||
)
|
||||
except Exception as e:
|
||||
print('Error\n\n', e, '\n\n')
|
||||
trainer.my_log.write(f"{args.epoch_begin + trainer.current_epoch} {trainer.my_epoch_loss:.6f} {math.exp(trainer.my_epoch_loss):.4f} {trainer.my_lr:.8f} {datetime.datetime.now()} {trainer.current_epoch}\n")
|
||||
print("Error\n\n", e, "\n\n")
|
||||
trainer.my_log.write(
|
||||
f"{args.epoch_begin + trainer.current_epoch} {trainer.my_epoch_loss:.6f} {math.exp(trainer.my_epoch_loss):.4f} {trainer.my_lr:.8f} {datetime.datetime.now()} {trainer.current_epoch}\n"
|
||||
)
|
||||
trainer.my_log.flush()
|
||||
|
||||
trainer.my_loss_sum = 0
|
||||
@ -178,22 +197,22 @@ def generate_init_weight(model, init_weight_name):
|
||||
mm[k] = src.reshape(mm[k].shape)
|
||||
except:
|
||||
tmp = mm[k].squeeze().clone()
|
||||
print(k, src.shape, '-->', mm[k].shape)
|
||||
print(k, src.shape, "-->", mm[k].shape)
|
||||
ss = src.shape[0]
|
||||
dd = tmp.shape[0]
|
||||
for i in range(dd):
|
||||
pos = i / dd * ss
|
||||
if pos >= ss - 1:
|
||||
tmp[i] = src[ss-1]
|
||||
tmp[i] = src[ss - 1]
|
||||
else:
|
||||
p0 = int(math.floor(pos))
|
||||
ii = pos - p0
|
||||
tmp[i] = src[p0] * (1-ii) + src[p0+1] * (ii)
|
||||
tmp[i] = src[p0] * (1 - ii) + src[p0 + 1] * (ii)
|
||||
mm[k] = tmp.reshape(mm[k].shape)
|
||||
sss = src.squeeze().float().cpu().numpy()
|
||||
print(sss[:10], '...', sss[-10:])
|
||||
print(sss[:10], "...", sss[-10:])
|
||||
mmm = mm[k].squeeze().float().cpu().numpy()
|
||||
print(mmm[:10], '...', mmm[-10:])
|
||||
print(mmm[:10], "...", mmm[-10:])
|
||||
|
||||
print(f"Save to {init_weight_name}...")
|
||||
torch.save(mm, init_weight_name)
|
@ -6,6 +6,7 @@ from torch.nn import functional as F
|
||||
time_slot = {}
|
||||
time_ref = time.time_ns()
|
||||
|
||||
|
||||
def record_time(name):
|
||||
if name not in time_slot:
|
||||
time_slot[name] = 1e20
|
||||
@ -13,20 +14,23 @@ def record_time(name):
|
||||
if tt < time_slot[name]:
|
||||
time_slot[name] = tt
|
||||
|
||||
class TOKENIZER():
|
||||
def __init__(self, WORD_NAME, UNKNOWN_CHAR='\ue083'):
|
||||
if 'list' in str(type(WORD_NAME)):
|
||||
|
||||
class TOKENIZER:
|
||||
def __init__(self, WORD_NAME, UNKNOWN_CHAR="\ue083"):
|
||||
if "list" in str(type(WORD_NAME)):
|
||||
self.charMode = False
|
||||
if WORD_NAME[0] == WORD_NAME[1]:
|
||||
from transformers import PreTrainedTokenizerFast
|
||||
|
||||
self.tokenizer = PreTrainedTokenizerFast(tokenizer_file=WORD_NAME[0])
|
||||
else:
|
||||
from transformers import GPT2TokenizerFast
|
||||
|
||||
self.tokenizer = GPT2TokenizerFast(WORD_NAME[0], WORD_NAME[1])
|
||||
self.vocab_size = len(self.tokenizer)
|
||||
else:
|
||||
self.charMode = True
|
||||
with open(WORD_NAME + '.json', "r", encoding="utf-16") as result_file:
|
||||
with open(WORD_NAME + ".json", "r", encoding="utf-16") as result_file:
|
||||
self.word_table = json.load(result_file)
|
||||
|
||||
self.vocab_size = len(self.word_table)
|
||||
@ -37,23 +41,25 @@ class TOKENIZER():
|
||||
self.UNKNOWN_CHAR = self.stoi[UNKNOWN_CHAR]
|
||||
|
||||
def refine_context(self, context):
|
||||
context = context.strip().split('\n')
|
||||
context = context.strip().split("\n")
|
||||
for c in range(len(context)):
|
||||
context[c] = context[c].strip().strip('\u3000').strip('\r')
|
||||
context = list(filter(lambda c: c != '', context))
|
||||
context = '\n' + ('\n'.join(context)).strip()
|
||||
if context == '':
|
||||
context = '\n'
|
||||
context[c] = context[c].strip().strip("\u3000").strip("\r")
|
||||
context = list(filter(lambda c: c != "", context))
|
||||
context = "\n" + ("\n".join(context)).strip()
|
||||
if context == "":
|
||||
context = "\n"
|
||||
return context
|
||||
|
||||
def sample_logits(self, out, x, ctx_len, temperature=1.0, top_p_usual=None, top_p_newline=None):
|
||||
def sample_logits(
|
||||
self, out, x, ctx_len, temperature=1.0, top_p_usual=None, top_p_newline=None
|
||||
):
|
||||
# out[self.UNKNOWN_CHAR] = -float('Inf')
|
||||
lastChar = int(x[-1])
|
||||
|
||||
probs = F.softmax(out, dim=-1)
|
||||
|
||||
if self.charMode:
|
||||
if self.itos[lastChar] == '\n':
|
||||
if self.itos[lastChar] == "\n":
|
||||
top_p = top_p_newline
|
||||
else:
|
||||
top_p = top_p_usual
|
||||
@ -81,6 +87,7 @@ class TOKENIZER():
|
||||
out = torch.multinomial(probs, num_samples=1)[0]
|
||||
return out
|
||||
|
||||
|
||||
def MaybeIsPrime(number):
|
||||
if FermatPrimalityTest(number) and MillerRabinPrimalityTest(number):
|
||||
return True
|
||||
@ -121,7 +128,9 @@ def MillerRabinPrimalityTest(number):
|
||||
if (randomNumberWithPower != 1) and (randomNumberWithPower != number - 1):
|
||||
iterationNumber = 1
|
||||
|
||||
while (iterationNumber <= timesTwoDividNumber - 1) and (randomNumberWithPower != number - 1):
|
||||
while (iterationNumber <= timesTwoDividNumber - 1) and (
|
||||
randomNumberWithPower != number - 1
|
||||
):
|
||||
randomNumberWithPower = pow(randomNumberWithPower, 2, number)
|
||||
iterationNumber = iterationNumber + 1
|
||||
if randomNumberWithPower != (number - 1):
|
@ -184,7 +184,7 @@ if __name__ == "__main__":
|
||||
args.num_sanity_val_steps = 0
|
||||
args.check_val_every_n_epoch = int(1e20)
|
||||
args.log_every_n_steps = int(1e20)
|
||||
args.max_epochs = args.epoch_count # continue forever
|
||||
args.max_epochs = args.epoch_count # -1 continue forever
|
||||
args.betas = (args.beta1, args.beta2)
|
||||
args.real_bsz = int(args.num_nodes) * int(args.devices) * args.micro_bsz
|
||||
os.environ["RWKV_T_MAX"] = str(args.ctx_len)
|
||||
@ -373,7 +373,7 @@ if __name__ == "__main__":
|
||||
for param in module.parameters():
|
||||
param.requires_grad = True
|
||||
elif enable_time_finetune and any(
|
||||
n.startswith("time") for n, _ in module.named_parameters()
|
||||
n.startswith("time") for n, _ in module.named_parameters()
|
||||
):
|
||||
for pname, param in module.named_parameters():
|
||||
if pname.startswith("time"):
|
||||
@ -381,7 +381,7 @@ if __name__ == "__main__":
|
||||
param.requires_grad = True
|
||||
|
||||
if (
|
||||
len(args.load_model) == 0 or args.my_pile_stage == 1
|
||||
len(args.load_model) == 0 or args.my_pile_stage == 1
|
||||
): # shall we build the initial weights?
|
||||
init_weight_name = f"{args.proj_dir}/rwkv-init.pth"
|
||||
generate_init_weight(model, init_weight_name) # save initial weights
|
||||
@ -423,8 +423,8 @@ if __name__ == "__main__":
|
||||
)
|
||||
|
||||
if (
|
||||
args.lr_init > 1e-4
|
||||
or trainer.world_size * args.micro_bsz * trainer.accumulate_grad_batches < 8
|
||||
args.lr_init > 1e-4
|
||||
or trainer.world_size * args.micro_bsz * trainer.accumulate_grad_batches < 8
|
||||
):
|
||||
if "I_KNOW_WHAT_IM_DOING" in os.environ:
|
||||
if trainer.global_rank == 0:
|
||||
@ -459,10 +459,10 @@ if __name__ == "__main__":
|
||||
|
||||
if "deepspeed" in args.strategy:
|
||||
trainer.strategy.config["zero_optimization"]["allgather_bucket_size"] = (
|
||||
args.ds_bucket_mb * 1000 * 1000
|
||||
args.ds_bucket_mb * 1000 * 1000
|
||||
)
|
||||
trainer.strategy.config["zero_optimization"]["reduce_bucket_size"] = (
|
||||
args.ds_bucket_mb * 1000 * 1000
|
||||
args.ds_bucket_mb * 1000 * 1000
|
||||
)
|
||||
|
||||
# must set shuffle=False, persistent_workers=False (because worker is in another thread)
|
202
finetune/lora/v5/cuda/wkv5_cuda.cu
vendored
Normal file
202
finetune/lora/v5/cuda/wkv5_cuda.cu
vendored
Normal file
@ -0,0 +1,202 @@
|
||||
#include <stdio.h>
|
||||
#include <assert.h>
|
||||
#include "ATen/ATen.h"
|
||||
typedef at::BFloat16 bf16;
|
||||
|
||||
template <typename F>
|
||||
__global__ void kernel_forward(const int B, const int T, const int C, const int H,
|
||||
const F *__restrict__ const _r, const F *__restrict__ const _k, const F *__restrict__ const _v, const float *__restrict__ _w, const F *__restrict__ _u,
|
||||
F *__restrict__ const _y)
|
||||
{
|
||||
const int b = blockIdx.x / H;
|
||||
const int h = blockIdx.x % H;
|
||||
const int i = threadIdx.x;
|
||||
_w += h*_N_;
|
||||
_u += h*_N_;
|
||||
|
||||
__shared__ float r[_N_], k[_N_], u[_N_], w[_N_];
|
||||
float state[_N_] = {0};
|
||||
|
||||
__syncthreads();
|
||||
w[i] = _w[i];
|
||||
u[i] = float(_u[i]);
|
||||
__syncthreads();
|
||||
|
||||
for (int t = b*T*C + h*_N_ + i; t < (b+1)*T*C + h*_N_ + i; t += C)
|
||||
{
|
||||
__syncthreads();
|
||||
r[i] = float(_r[t]);
|
||||
k[i] = float(_k[t]);
|
||||
__syncthreads();
|
||||
|
||||
const float v = float(_v[t]);
|
||||
float y = 0;
|
||||
|
||||
#pragma unroll
|
||||
for (int j = 0; j < _N_; j+=4)
|
||||
{
|
||||
const float4& r_ = (float4&)(r[j]);
|
||||
const float4& k_ = (float4&)(k[j]);
|
||||
const float4& w_ = (float4&)(w[j]);
|
||||
const float4& u_ = (float4&)(u[j]);
|
||||
float4& s = (float4&)(state[j]);
|
||||
float4 x;
|
||||
|
||||
x.x = k_.x * v;
|
||||
x.y = k_.y * v;
|
||||
x.z = k_.z * v;
|
||||
x.w = k_.w * v;
|
||||
|
||||
y += r_.x * (u_.x * x.x + s.x);
|
||||
y += r_.y * (u_.y * x.y + s.y);
|
||||
y += r_.z * (u_.z * x.z + s.z);
|
||||
y += r_.w * (u_.w * x.w + s.w);
|
||||
|
||||
s.x = s.x * w_.x + x.x;
|
||||
s.y = s.y * w_.y + x.y;
|
||||
s.z = s.z * w_.z + x.z;
|
||||
s.w = s.w * w_.w + x.w;
|
||||
}
|
||||
_y[t] = F(y);
|
||||
}
|
||||
}
|
||||
|
||||
template <typename F>
|
||||
__global__ void kernel_backward(const int B, const int T, const int C, const int H,
|
||||
const F *__restrict__ const _r, const F *__restrict__ const _k, const F *__restrict__ const _v, const float *__restrict__ _w, const float *__restrict__ __w, const F *__restrict__ _u, const F *__restrict__ const _gy,
|
||||
F *__restrict__ const _gr, F *__restrict__ const _gk, F *__restrict__ const _gv, F *__restrict__ const _gw, F *__restrict__ const _gu)
|
||||
{
|
||||
const int b = blockIdx.x / H;
|
||||
const int h = blockIdx.x % H;
|
||||
const int i = threadIdx.x;
|
||||
_w += h*_N_;
|
||||
_u += h*_N_;
|
||||
__w += h*_N_;
|
||||
|
||||
__shared__ float w_[_N_], u_[_N_];
|
||||
__shared__ float r[_N_], k[_N_], v[_N_], gy[_N_];
|
||||
__syncthreads();
|
||||
w_[i] = _w[i];
|
||||
u_[i] = float(_u[i]);
|
||||
__syncthreads();
|
||||
|
||||
const float w = w_[i];
|
||||
const float ww = __w[i];
|
||||
const float u = u_[i];
|
||||
|
||||
float state[_N_] = {0}, saaaa[_N_] = {0}, sbbbb[_N_] = {0}, scccc[_N_] = {0}, sdddd[_N_] = {0};
|
||||
|
||||
float gw = 0, gu = 0;
|
||||
const int t000 = b*T*C + h*_N_ + i;
|
||||
const int t111 = (b+1)*T*C + h*_N_ + i;
|
||||
const int t222 = t111 - 2*C;
|
||||
|
||||
for (int t = t000; t < t111; t += C)
|
||||
{
|
||||
__syncthreads();
|
||||
v[i] = float(_v[t]);
|
||||
gy[i] = float(_gy[t]);
|
||||
__syncthreads();
|
||||
|
||||
const float k = float(_k[t]);
|
||||
float gr = 0, gu_ = 0;
|
||||
|
||||
#pragma unroll
|
||||
for (int j = 0; j < _N_; j++)
|
||||
{
|
||||
float& s = state[j];
|
||||
float x = k * v[j];
|
||||
|
||||
gr += (u * x + s) * gy[j];
|
||||
gu_ += x * gy[j];
|
||||
s = s * w + x;
|
||||
}
|
||||
_gr[t] = F(gr);
|
||||
gu += float(_r[t]) * gu_;
|
||||
}
|
||||
_gu[b*C + h*_N_ + i] = F(gu);
|
||||
|
||||
for (int t = t000; t < t222; t += C)
|
||||
{
|
||||
__syncthreads();
|
||||
v[i] = float(_v[t]);
|
||||
gy[i] = float(_gy[t + 2*C]);
|
||||
__syncthreads();
|
||||
|
||||
const float k = float(_k[t]);
|
||||
float gw_ = 0;
|
||||
|
||||
#pragma unroll
|
||||
for (int j = 0; j < _N_; j++)
|
||||
{
|
||||
float& s = saaaa[j];
|
||||
float& s2 = sbbbb[j];
|
||||
float x = k * v[j];
|
||||
|
||||
float tmp = w * (x + s);
|
||||
s = tmp;
|
||||
s2 = tmp + w * s2;
|
||||
gw_ += s2 * gy[j];
|
||||
}
|
||||
gw += float(_r[t + 2*C]) * gw_;
|
||||
}
|
||||
_gw[b*C + h*_N_ + i] = F(ww * gw);
|
||||
|
||||
for (int t = t111 - C; t >= t000; t -= C)
|
||||
{
|
||||
__syncthreads();
|
||||
v[i] = float(_v[t]);
|
||||
gy[i] = float(_gy[t]);
|
||||
__syncthreads();
|
||||
|
||||
const float rr = float(_r[t]);
|
||||
float gk = 0;
|
||||
|
||||
#pragma unroll
|
||||
for (int j = 0; j < _N_; j++)
|
||||
{
|
||||
float& s = scccc[j];
|
||||
float x = rr * gy[j];
|
||||
|
||||
gk += (u * x + s) * v[j];
|
||||
s = x + s * w;
|
||||
}
|
||||
_gk[t] = F(gk);
|
||||
}
|
||||
|
||||
for (int t = t111 - C; t >= t000; t -= C)
|
||||
{
|
||||
__syncthreads();
|
||||
r[i] = float(_r[t]);
|
||||
k[i] = float(_k[t]);
|
||||
__syncthreads();
|
||||
|
||||
const float gyy = float(_gy[t]);
|
||||
float gv = 0;
|
||||
|
||||
#pragma unroll
|
||||
for (int j = 0; j < _N_; j++)
|
||||
{
|
||||
float& s = sdddd[j];
|
||||
float x = gyy * r[j];
|
||||
|
||||
gv += (u_[j] * x + s) * k[j];
|
||||
s = x + s * w_[j];
|
||||
}
|
||||
_gv[t] = F(gv);
|
||||
}
|
||||
}
|
||||
|
||||
void cuda_forward(int B, int T, int C, int H, bf16 *r, bf16 *k, bf16 *v, float *w, bf16 *u, bf16 *y)
|
||||
{
|
||||
assert(H*_N_ == C);
|
||||
assert(_N_%4 == 0);
|
||||
kernel_forward<<<dim3(B * H), dim3(_N_)>>>(B, T, C, H, r, k, v, w, u, y);
|
||||
}
|
||||
|
||||
void cuda_backward(int B, int T, int C, int H, bf16 *r, bf16 *k, bf16 *v, float *w, float *ww, bf16 *u, bf16 *gy, bf16 *gr, bf16 *gk, bf16 *gv, bf16 *gw, bf16 *gu)
|
||||
{
|
||||
assert(H*_N_ == C);
|
||||
assert(_N_%4 == 0);
|
||||
kernel_backward<<<dim3(B * H), dim3(_N_)>>>(B, T, C, H, r, k, v, w, ww, u, gy, gr, gk, gv, gw, gu);
|
||||
}
|
22
finetune/lora/v5/cuda/wkv5_op.cpp
vendored
Normal file
22
finetune/lora/v5/cuda/wkv5_op.cpp
vendored
Normal file
@ -0,0 +1,22 @@
|
||||
#include <torch/extension.h>
|
||||
#include "ATen/ATen.h"
|
||||
typedef at::BFloat16 bf16;
|
||||
|
||||
void cuda_forward(int B, int T, int C, int H, bf16 *r, bf16 *k, bf16 *v, float *w, bf16 *u, bf16 *y);
|
||||
void cuda_backward(int B, int T, int C, int H, bf16 *r, bf16 *k, bf16 *v, float *w, float *ww, bf16 *u, bf16 *gy, bf16 *gr, bf16 *gk, bf16 *gv, bf16 *gw, bf16 *gu);
|
||||
|
||||
void forward(int64_t B, int64_t T, int64_t C, int64_t H, torch::Tensor &r, torch::Tensor &k, torch::Tensor &v, torch::Tensor &w, torch::Tensor &u, torch::Tensor &y) {
|
||||
cuda_forward(B, T, C, H, r.data_ptr<bf16>(), k.data_ptr<bf16>(), v.data_ptr<bf16>(), w.data_ptr<float>(), u.data_ptr<bf16>(), y.data_ptr<bf16>());
|
||||
}
|
||||
void backward(int64_t B, int64_t T, int64_t C, int64_t H, torch::Tensor &r, torch::Tensor &k, torch::Tensor &v, torch::Tensor &w, torch::Tensor &ww, torch::Tensor &u, torch::Tensor &gy, torch::Tensor &gr, torch::Tensor &gk, torch::Tensor &gv, torch::Tensor &gw, torch::Tensor &gu) {
|
||||
cuda_backward(B, T, C, H, r.data_ptr<bf16>(), k.data_ptr<bf16>(), v.data_ptr<bf16>(), w.data_ptr<float>(), ww.data_ptr<float>(), u.data_ptr<bf16>(), gy.data_ptr<bf16>(), gr.data_ptr<bf16>(), gk.data_ptr<bf16>(), gv.data_ptr<bf16>(), gw.data_ptr<bf16>(), gu.data_ptr<bf16>());
|
||||
}
|
||||
PYBIND11_MODULE(TORCH_EXTENSION_NAME, m) {
|
||||
m.def("forward", &forward, "wkv5 forward");
|
||||
m.def("backward", &backward, "wkv5 backward");
|
||||
}
|
||||
|
||||
TORCH_LIBRARY(wkv5, m) {
|
||||
m.def("forward", forward);
|
||||
m.def("backward", backward);
|
||||
}
|
0
finetune/lora/v5/src/__init__.py
vendored
Normal file
0
finetune/lora/v5/src/__init__.py
vendored
Normal file
303
finetune/lora/v5/src/binidx.py
vendored
Normal file
303
finetune/lora/v5/src/binidx.py
vendored
Normal file
@ -0,0 +1,303 @@
|
||||
from lib2to3.pgen2 import token
|
||||
import os
|
||||
import torch
|
||||
import numpy as np
|
||||
import shutil
|
||||
import struct
|
||||
from functools import lru_cache
|
||||
from itertools import accumulate
|
||||
|
||||
|
||||
def print_rank_0(*message):
|
||||
pass
|
||||
# """If distributed is initialized print only on rank 0."""
|
||||
# if torch.distributed.is_initialized():
|
||||
# if torch.distributed.get_rank() == 0:
|
||||
# print(*message, flush=True)
|
||||
# else:
|
||||
# print(*message, flush=True)
|
||||
|
||||
|
||||
def _warmup_mmap_file(path):
|
||||
pass
|
||||
# with open(path, "rb") as stream:
|
||||
# while stream.read(100 * 1024 * 1024):
|
||||
# pass
|
||||
|
||||
|
||||
dtypes = {
|
||||
1: np.uint8,
|
||||
2: np.int8,
|
||||
3: np.int16,
|
||||
4: np.int32,
|
||||
5: np.int64,
|
||||
6: float,
|
||||
7: np.double,
|
||||
8: np.uint16,
|
||||
}
|
||||
|
||||
|
||||
def code(dtype):
|
||||
for k in dtypes.keys():
|
||||
if dtypes[k] == dtype:
|
||||
return k
|
||||
raise ValueError(dtype)
|
||||
|
||||
|
||||
def index_file_path(prefix_path):
|
||||
return prefix_path + ".idx"
|
||||
|
||||
|
||||
def data_file_path(prefix_path):
|
||||
return prefix_path + ".bin"
|
||||
|
||||
|
||||
class MMapIndexedDataset(torch.utils.data.Dataset):
|
||||
class Index(object):
|
||||
_HDR_MAGIC = b"MMIDIDX\x00\x00"
|
||||
|
||||
@classmethod
|
||||
def writer(cls, path, dtype):
|
||||
class _Writer(object):
|
||||
def __enter__(self):
|
||||
self._file = open(path, "wb")
|
||||
|
||||
# Write Magic string so we can check the file format then opening it again.
|
||||
self._file.write(cls._HDR_MAGIC)
|
||||
# Write version number
|
||||
# Little endian unsigned 64 Bit integer
|
||||
self._file.write(struct.pack("<Q", 1))
|
||||
# Little endian unsigned 8 Bit integer
|
||||
self._file.write(struct.pack("<B", code(dtype)))
|
||||
|
||||
return self
|
||||
|
||||
@staticmethod
|
||||
def _get_pointers(sizes):
|
||||
dtype_size = dtype().itemsize
|
||||
address = 0
|
||||
pointers = []
|
||||
|
||||
for size in sizes:
|
||||
pointers.append(address)
|
||||
address += size * dtype_size
|
||||
|
||||
return pointers
|
||||
|
||||
def write(self, sizes, doc_idx):
|
||||
pointers = self._get_pointers(sizes)
|
||||
|
||||
# Little endian unsigned 64 Bit integer
|
||||
self._file.write(struct.pack("<Q", len(sizes)))
|
||||
# Little endian unsigned 64 Bit integer
|
||||
self._file.write(struct.pack("<Q", len(doc_idx)))
|
||||
|
||||
sizes = np.array(sizes, dtype=np.int32)
|
||||
self._file.write(sizes.tobytes(order="C"))
|
||||
del sizes
|
||||
|
||||
pointers = np.array(pointers, dtype=np.int64)
|
||||
self._file.write(pointers.tobytes(order="C"))
|
||||
del pointers
|
||||
|
||||
doc_idx = np.array(doc_idx, dtype=np.int64)
|
||||
self._file.write(doc_idx.tobytes(order="C"))
|
||||
|
||||
def __exit__(self, exc_type, exc_val, exc_tb):
|
||||
self._file.close()
|
||||
|
||||
return _Writer()
|
||||
|
||||
def __init__(self, path, skip_warmup=False):
|
||||
with open(path, "rb") as stream:
|
||||
magic_test = stream.read(9)
|
||||
assert self._HDR_MAGIC == magic_test, (
|
||||
"Index file doesn't match expected format. "
|
||||
"Make sure that --dataset-impl is configured properly."
|
||||
)
|
||||
# Little endian unsigned 64 Bit integer
|
||||
version = struct.unpack("<Q", stream.read(8))
|
||||
assert (1,) == version
|
||||
|
||||
# Little endian unsigned 8 Bit integer
|
||||
(dtype_code,) = struct.unpack("<B", stream.read(1))
|
||||
self._dtype = dtypes[dtype_code]
|
||||
self._dtype_size = self._dtype().itemsize
|
||||
|
||||
self._len = struct.unpack("<Q", stream.read(8))[0]
|
||||
self._doc_count = struct.unpack("<Q", stream.read(8))[0]
|
||||
offset = stream.tell()
|
||||
|
||||
if not skip_warmup:
|
||||
print_rank_0(" warming up index mmap file...")
|
||||
_warmup_mmap_file(path)
|
||||
|
||||
self._bin_buffer_mmap = np.memmap(path, mode="r", order="C")
|
||||
self._bin_buffer = memoryview(self._bin_buffer_mmap)
|
||||
print_rank_0(" reading sizes...")
|
||||
self._sizes = np.frombuffer(
|
||||
self._bin_buffer, dtype=np.int32, count=self._len, offset=offset
|
||||
)
|
||||
print_rank_0(" reading pointers...")
|
||||
self._pointers = np.frombuffer(
|
||||
self._bin_buffer,
|
||||
dtype=np.int64,
|
||||
count=self._len,
|
||||
offset=offset + self._sizes.nbytes,
|
||||
)
|
||||
print_rank_0(" reading document index...")
|
||||
self._doc_idx = np.frombuffer(
|
||||
self._bin_buffer,
|
||||
dtype=np.int64,
|
||||
count=self._doc_count,
|
||||
offset=offset + self._sizes.nbytes + self._pointers.nbytes,
|
||||
)
|
||||
|
||||
def __del__(self):
|
||||
self._bin_buffer_mmap._mmap.close()
|
||||
del self._bin_buffer_mmap
|
||||
|
||||
@property
|
||||
def dtype(self):
|
||||
return self._dtype
|
||||
|
||||
@property
|
||||
def sizes(self):
|
||||
return self._sizes
|
||||
|
||||
@property
|
||||
def doc_idx(self):
|
||||
return self._doc_idx
|
||||
|
||||
@lru_cache(maxsize=8)
|
||||
def __getitem__(self, i):
|
||||
return self._pointers[i], self._sizes[i]
|
||||
|
||||
def __len__(self):
|
||||
return self._len
|
||||
|
||||
def __init__(self, path, skip_warmup=False):
|
||||
super().__init__()
|
||||
|
||||
self._path = None
|
||||
self._index = None
|
||||
self._bin_buffer = None
|
||||
|
||||
self._do_init(path, skip_warmup)
|
||||
|
||||
def __getstate__(self):
|
||||
return self._path
|
||||
|
||||
def __setstate__(self, state):
|
||||
self._do_init(state)
|
||||
|
||||
def _do_init(self, path, skip_warmup):
|
||||
self._path = path
|
||||
self._index = self.Index(index_file_path(self._path), skip_warmup)
|
||||
|
||||
if not skip_warmup:
|
||||
print_rank_0(" warming up data mmap file...")
|
||||
_warmup_mmap_file(data_file_path(self._path))
|
||||
print_rank_0(" creating numpy buffer of mmap...")
|
||||
self._bin_buffer_mmap = np.memmap(
|
||||
data_file_path(self._path), mode="r", order="C"
|
||||
)
|
||||
print_rank_0(" creating memory view of numpy buffer...")
|
||||
self._bin_buffer = memoryview(self._bin_buffer_mmap)
|
||||
|
||||
def __del__(self):
|
||||
self._bin_buffer_mmap._mmap.close()
|
||||
del self._bin_buffer_mmap
|
||||
del self._index
|
||||
|
||||
def __len__(self):
|
||||
return len(self._index)
|
||||
|
||||
# @lru_cache(maxsize=8)
|
||||
def __getitem__(self, idx):
|
||||
if isinstance(idx, int):
|
||||
ptr, size = self._index[idx]
|
||||
np_array = np.frombuffer(
|
||||
self._bin_buffer, dtype=self._index.dtype, count=size, offset=ptr
|
||||
)
|
||||
return np_array
|
||||
elif isinstance(idx, slice):
|
||||
start, stop, step = idx.indices(len(self))
|
||||
if step != 1:
|
||||
raise ValueError("Slices into indexed_dataset must be contiguous")
|
||||
ptr = self._index._pointers[start]
|
||||
sizes = self._index._sizes[idx]
|
||||
offsets = list(accumulate(sizes))
|
||||
total_size = sum(sizes)
|
||||
np_array = np.frombuffer(
|
||||
self._bin_buffer, dtype=self._index.dtype, count=total_size, offset=ptr
|
||||
)
|
||||
sents = np.split(np_array, offsets[:-1])
|
||||
return sents
|
||||
|
||||
def get(self, idx, offset=0, length=None):
|
||||
"""Retrieves a single item from the dataset with the option to only
|
||||
return a portion of the item.
|
||||
|
||||
get(idx) is the same as [idx] but get() does not support slicing.
|
||||
"""
|
||||
ptr, size = self._index[idx]
|
||||
if length is None:
|
||||
length = size - offset
|
||||
ptr += offset * np.dtype(self._index.dtype).itemsize
|
||||
np_array = np.frombuffer(
|
||||
self._bin_buffer, dtype=self._index.dtype, count=length, offset=ptr
|
||||
)
|
||||
return np_array
|
||||
|
||||
def pad(self, idx, length=None):
|
||||
ptr, size = self._index[idx]
|
||||
try:
|
||||
np_array = np.frombuffer(
|
||||
self._bin_buffer, dtype=self._index.dtype, count=length, offset=ptr
|
||||
)
|
||||
except:
|
||||
np_array = np.frombuffer(
|
||||
self._bin_buffer, dtype=self._index.dtype, count=size, offset=ptr
|
||||
)
|
||||
ptr0, _ = self._index[0]
|
||||
np_array0 = np.frombuffer(
|
||||
self._bin_buffer,
|
||||
dtype=self._index.dtype,
|
||||
count=length - size,
|
||||
offset=ptr0,
|
||||
)
|
||||
np_array = np.append(np_array, np_array0)
|
||||
return np_array
|
||||
|
||||
def only(self, idx):
|
||||
ptr, size = self._index[idx]
|
||||
np_array = np.frombuffer(
|
||||
self._bin_buffer, dtype=self._index.dtype, count=size, offset=ptr
|
||||
)
|
||||
|
||||
return np_array
|
||||
|
||||
@property
|
||||
def sizes(self):
|
||||
return self._index.sizes
|
||||
|
||||
@property
|
||||
def doc_idx(self):
|
||||
return self._index.doc_idx
|
||||
|
||||
def get_doc_idx(self):
|
||||
return self._index._doc_idx
|
||||
|
||||
def set_doc_idx(self, doc_idx_):
|
||||
self._index._doc_idx = doc_idx_
|
||||
|
||||
@property
|
||||
def supports_prefetch(self):
|
||||
return False
|
||||
|
||||
@staticmethod
|
||||
def exists(path):
|
||||
return os.path.exists(index_file_path(path)) and os.path.exists(
|
||||
data_file_path(path)
|
||||
)
|
241
finetune/lora/v5/src/dataset.py
vendored
Normal file
241
finetune/lora/v5/src/dataset.py
vendored
Normal file
@ -0,0 +1,241 @@
|
||||
########################################################################################################
|
||||
# The RWKV Language Model - https://github.com/BlinkDL/RWKV-LM
|
||||
########################################################################################################
|
||||
|
||||
import json, math, random, os, sys
|
||||
import numpy as np
|
||||
import torch
|
||||
from torch.utils.data import Dataset
|
||||
from pytorch_lightning.utilities import rank_zero_info
|
||||
from .binidx import MMapIndexedDataset
|
||||
from .utils import MaybeIsPrime
|
||||
|
||||
|
||||
class MyDataset(Dataset):
|
||||
def __init__(self, args):
|
||||
self.args = args
|
||||
|
||||
if args.data_type == "binidx":
|
||||
self.vocab_size = args.vocab_size
|
||||
rank_zero_info(
|
||||
f"Current vocab size = {self.vocab_size} (make sure it's correct)"
|
||||
)
|
||||
|
||||
if args.my_pile_version == 1:
|
||||
self.data = MMapIndexedDataset(args.data_file)
|
||||
self.data_size = (
|
||||
len(self.data._bin_buffer) // self.data._index._dtype_size
|
||||
)
|
||||
rank_zero_info(f"Data has {self.data_size} tokens.")
|
||||
elif args.my_pile_version == 2:
|
||||
data_list = (
|
||||
open(args.data_file, "r", encoding="utf-8")
|
||||
.read()
|
||||
.strip()
|
||||
.split("\n")
|
||||
)
|
||||
data_list = [i.strip().split(" ") for i in data_list]
|
||||
self.data = []
|
||||
self.data_size = int(data_list[-1][-1])
|
||||
rank_zero_info(f"Data has {self.data_size} chunks.")
|
||||
for d in data_list:
|
||||
data = MMapIndexedDataset(d[0])
|
||||
data_size = len(data._bin_buffer) // data._index._dtype_size
|
||||
assert (data_size - args.ctx_len) == int(d[1])
|
||||
self.data += [[int(d[-1]), int(d[1]), data]]
|
||||
# rank_zero_info(self.data)
|
||||
|
||||
if args.my_qa_mask > 0:
|
||||
# self.data_pile = MMapIndexedDataset('/fsx/pile/pile_20B_tokenizer_text_document')
|
||||
self.data_pile = MMapIndexedDataset(
|
||||
"/fsx/pile_deduped/pile_0.87_deduped_text_document"
|
||||
)
|
||||
self.data_pile_size = (
|
||||
len(self.data_pile._bin_buffer) // self.data._index._dtype_size
|
||||
)
|
||||
else:
|
||||
self.data_pile = None
|
||||
self.data_pile_size = 0
|
||||
|
||||
if args.my_pile_stage > 0:
|
||||
# assert self.data_size == 332115325534 and self.vocab_size == 50277
|
||||
self.samples_per_epoch = args.epoch_steps * args.real_bsz
|
||||
assert self.samples_per_epoch == 40320
|
||||
rank_zero_info(
|
||||
f"########## Pile 20b-tokenized stage {args.my_pile_stage} ##########"
|
||||
)
|
||||
dataset_slot = self.data_size // args.ctx_len
|
||||
if args.my_pile_stage != 4:
|
||||
assert MaybeIsPrime(args.magic_prime)
|
||||
assert args.magic_prime % 3 == 2
|
||||
assert (
|
||||
args.magic_prime / dataset_slot > 0.99
|
||||
and args.magic_prime / dataset_slot <= 1
|
||||
)
|
||||
elif args.data_type == "numpy":
|
||||
self.data = np.load(args.data_file).astype("int")
|
||||
self.vocab_size = args.vocab_size
|
||||
rank_zero_info(
|
||||
f"Current vocab size = {self.vocab_size} (make sure it's correct)"
|
||||
)
|
||||
self.data_size = len(self.data)
|
||||
rank_zero_info(f"Data has {self.data_size} tokens.")
|
||||
elif args.data_type == "uint16":
|
||||
self.data = (
|
||||
np.fromfile(args.data_file, dtype=np.uint16)
|
||||
.astype("int32")
|
||||
.reshape(-1, args.my_sample_len)
|
||||
)
|
||||
self.vocab_size = args.vocab_size
|
||||
rank_zero_info(
|
||||
f"Current vocab size = {self.vocab_size} (make sure it's correct)"
|
||||
)
|
||||
self.data_size = self.data.shape[0]
|
||||
rank_zero_info(f"Data has {self.data_size} samples.")
|
||||
else:
|
||||
if args.data_type == "dummy":
|
||||
rank_zero_info("Building dummy data...")
|
||||
self.data = ""
|
||||
for i in range(100000):
|
||||
aa = (i) % 10000
|
||||
bb = (i * i) % 10000
|
||||
cc = aa + bb
|
||||
self.data += f".{aa}+{bb}={cc}."
|
||||
else:
|
||||
self.data = open(args.data_file, "r", encoding=args.data_type).read()
|
||||
rank_zero_info("Building token list...")
|
||||
unique = sorted(list(set(self.data)))
|
||||
self.vocab_size = len(unique)
|
||||
# rank_zero_info()
|
||||
# for u in unique:
|
||||
# print(u, end=' ')
|
||||
# rank_zero_info('\n\n')
|
||||
xx = 0
|
||||
xxObj = {}
|
||||
for u in unique:
|
||||
xxObj[xx] = u
|
||||
xx += 1
|
||||
with open(
|
||||
f"{args.proj_dir}/vocab.json", "w", encoding="utf-8"
|
||||
) as vocab_file:
|
||||
vocab_file.write(json.dumps(xxObj, ensure_ascii=False))
|
||||
self.data_size = len(self.data)
|
||||
rank_zero_info(
|
||||
f"Data has {self.data_size} tokens, {self.vocab_size} vocab size."
|
||||
)
|
||||
self.stoi = {ch: i for i, ch in enumerate(unique)}
|
||||
self.itos = {i: ch for i, ch in enumerate(unique)}
|
||||
|
||||
def __len__(self):
|
||||
return self.args.epoch_steps * self.args.micro_bsz
|
||||
|
||||
def __getitem__(self, idx):
|
||||
args = self.args
|
||||
rank = self.global_rank
|
||||
epoch = self.real_epoch
|
||||
world_size = self.world_size
|
||||
# print(f"epoch {epoch} idx {idx} rank {rank}/{world_size}")
|
||||
|
||||
if args.data_type == "uint16":
|
||||
i = np.random.randint(0, self.data_size - 1)
|
||||
dix = self.data[i]
|
||||
x = torch.tensor(dix[:-1], dtype=torch.long)
|
||||
y = torch.tensor(dix[1:], dtype=torch.long)
|
||||
else:
|
||||
ctx_len = args.ctx_len
|
||||
req_len = ctx_len + 1
|
||||
magic_prime = args.magic_prime
|
||||
data = self.data
|
||||
|
||||
if args.my_pile_stage > 0:
|
||||
ii = 1 + epoch * self.samples_per_epoch + (idx * world_size) + rank
|
||||
|
||||
if args.my_qa_mask > 0:
|
||||
ii_orig = ii
|
||||
if ii % 2 == 0:
|
||||
ii = -1
|
||||
data = self.data_pile
|
||||
else:
|
||||
ii = ii // 2
|
||||
if data == self.data_pile:
|
||||
i = np.random.randint(0, self.data_pile_size - req_len)
|
||||
else:
|
||||
if args.my_pile_stage == 4 or ii < args.my_random_steps:
|
||||
# cheat: pick a random spot in dataset
|
||||
if args.my_pile_version == 1:
|
||||
i = np.random.randint(0, self.data_size - req_len)
|
||||
else:
|
||||
i = np.random.randint(0, self.data_size)
|
||||
else:
|
||||
ii = ii - args.my_random_steps
|
||||
factor = (math.sqrt(5) - 1) / 2
|
||||
factor = int(magic_prime * factor)
|
||||
i = ((factor * ii * ii * ii) % magic_prime) * ctx_len
|
||||
i = i + args.my_pile_shift
|
||||
# print(f"epoch {epoch} idx {idx} rank {rank}/{world_size} ii {ii} pos {round(i / self.data_size, 3)}")
|
||||
else:
|
||||
# cheat: pick a random spot in dataset
|
||||
i = np.random.randint(0, self.data_size - req_len)
|
||||
|
||||
if args.data_type == "binidx":
|
||||
if args.my_pile_version == 1:
|
||||
dix = data.get(idx=0, offset=i, length=req_len).astype(int)
|
||||
else:
|
||||
# self.data : cutoff, chunk_count, data
|
||||
for j in range(len(data)):
|
||||
if i < data[j][0]:
|
||||
ii = i
|
||||
i = (i - (data[j - 1][0] if j > 0 else 0)) % data[j][1]
|
||||
dix = (
|
||||
data[j][2]
|
||||
.get(idx=0, offset=i, length=req_len)
|
||||
.astype(int)
|
||||
)
|
||||
# print(ii, j, i)
|
||||
break
|
||||
elif args.data_type == "numpy":
|
||||
dix = data[i : i + req_len]
|
||||
else:
|
||||
dix = [self.stoi[s] for s in data[i : i + req_len]]
|
||||
|
||||
if args.my_qa_mask == 1:
|
||||
if data == self.data_pile:
|
||||
z = [1] * ctx_len
|
||||
else:
|
||||
z = [0] * ctx_len
|
||||
z_sum = 0
|
||||
isGood = False
|
||||
for i in range(3, ctx_len):
|
||||
if (
|
||||
dix[i] == 27
|
||||
and dix[i - 1] == 34
|
||||
and dix[i - 2] == 187
|
||||
and dix[i - 3] == 187
|
||||
):
|
||||
isGood = True
|
||||
if dix[i] == 0:
|
||||
isGood = False
|
||||
if isGood:
|
||||
z[i] = 1
|
||||
z_sum += 1
|
||||
if z_sum == 0:
|
||||
z = [1] * ctx_len
|
||||
i = np.random.randint(0, self.data_pile_size - req_len)
|
||||
dix = self.data_pile.get(
|
||||
idx=0, offset=i, length=req_len
|
||||
).astype(int)
|
||||
z = torch.tensor(z, dtype=torch.bfloat16)
|
||||
|
||||
x = torch.tensor(dix[:-1], dtype=torch.long)
|
||||
y = torch.tensor(dix[1:], dtype=torch.long)
|
||||
|
||||
# if ii_orig < 50:
|
||||
# # if rank == 1:
|
||||
# print('rank', rank, 'i', ii_orig, ii, i, 'x', x[:5], '...', x[-5:])
|
||||
# else:
|
||||
# exit(0)
|
||||
|
||||
if args.my_qa_mask == 1:
|
||||
return x, y, z
|
||||
|
||||
return x, y
|
819
finetune/lora/v5/src/model.py
vendored
Normal file
819
finetune/lora/v5/src/model.py
vendored
Normal file
@ -0,0 +1,819 @@
|
||||
########################################################################################################
|
||||
# The RWKV Language Model - https://github.com/BlinkDL/RWKV-LM
|
||||
########################################################################################################
|
||||
import functools
|
||||
import os, math, gc, importlib
|
||||
import torch
|
||||
|
||||
# torch._C._jit_set_profiling_executor(True)
|
||||
# torch._C._jit_set_profiling_mode(True)
|
||||
import torch.nn as nn
|
||||
from torch.utils.checkpoint import checkpoint as torch_checkpoint
|
||||
from torch.nn import functional as F
|
||||
import pytorch_lightning as pl
|
||||
from pytorch_lightning.utilities import rank_zero_info, rank_zero_only
|
||||
from pytorch_lightning.strategies import DeepSpeedStrategy
|
||||
|
||||
if importlib.util.find_spec("deepspeed"):
|
||||
import deepspeed
|
||||
from deepspeed.ops.adam import DeepSpeedCPUAdam, FusedAdam
|
||||
|
||||
|
||||
# from deepspeed.runtime.fp16.onebit.zoadam import ZeroOneAdam
|
||||
|
||||
# lora-config
|
||||
LORA_CONFIG = {
|
||||
"r": 0,
|
||||
"alpha": 0,
|
||||
"dropout": 0,
|
||||
"parts": {"att", "ln", "time"},
|
||||
}
|
||||
|
||||
try:
|
||||
print("RWKV_MY_TESTING", os.environ["RWKV_MY_TESTING"])
|
||||
except:
|
||||
os.environ["RWKV_MY_TESTING"] = ""
|
||||
|
||||
|
||||
def __nop(ob):
|
||||
return ob
|
||||
|
||||
|
||||
MyModule = nn.Module
|
||||
MyFunction = __nop
|
||||
if os.environ["RWKV_JIT_ON"] == "1":
|
||||
MyModule = torch.jit.ScriptModule
|
||||
MyFunction = torch.jit.script_method
|
||||
|
||||
|
||||
########################################################################################################
|
||||
# CUDA Kernel
|
||||
########################################################################################################
|
||||
|
||||
from torch.utils.cpp_extension import load
|
||||
|
||||
HEAD_SIZE = int(os.environ["RWKV_HEAD_SIZE_A"])
|
||||
wkv5_cuda = load(
|
||||
name="wkv5",
|
||||
sources=[
|
||||
"finetune/lora/v5/cuda/wkv5_op.cpp",
|
||||
f"finetune/lora/v5/cuda/wkv5_cuda.cu",
|
||||
],
|
||||
verbose=True,
|
||||
extra_cuda_cflags=[
|
||||
"-res-usage",
|
||||
"--use_fast_math",
|
||||
"-O3",
|
||||
"-Xptxas -O3",
|
||||
"--extra-device-vectorization",
|
||||
f"-D_N_={HEAD_SIZE}",
|
||||
],
|
||||
)
|
||||
|
||||
|
||||
class WKV_5(torch.autograd.Function):
|
||||
@staticmethod
|
||||
def forward(ctx, B, T, C, H, r, k, v, w, u):
|
||||
with torch.no_grad():
|
||||
assert r.dtype == torch.bfloat16
|
||||
assert k.dtype == torch.bfloat16
|
||||
assert v.dtype == torch.bfloat16
|
||||
assert w.dtype == torch.bfloat16
|
||||
assert u.dtype == torch.bfloat16
|
||||
assert HEAD_SIZE == C // H
|
||||
ctx.B = B
|
||||
ctx.T = T
|
||||
ctx.C = C
|
||||
ctx.H = H
|
||||
assert r.is_contiguous()
|
||||
assert k.is_contiguous()
|
||||
assert v.is_contiguous()
|
||||
assert w.is_contiguous()
|
||||
assert u.is_contiguous()
|
||||
ew = (-torch.exp(w.float())).contiguous()
|
||||
eew = (torch.exp(ew)).contiguous()
|
||||
ctx.save_for_backward(r, k, v, eew, ew, u)
|
||||
y = torch.empty(
|
||||
(B, T, C),
|
||||
device=r.device,
|
||||
dtype=torch.bfloat16,
|
||||
memory_format=torch.contiguous_format,
|
||||
) # .uniform_(-1, 1)
|
||||
wkv5_cuda.forward(B, T, C, H, r, k, v, eew, u, y)
|
||||
return y
|
||||
|
||||
@staticmethod
|
||||
def backward(ctx, gy):
|
||||
with torch.no_grad():
|
||||
assert gy.dtype == torch.bfloat16
|
||||
B = ctx.B
|
||||
T = ctx.T
|
||||
C = ctx.C
|
||||
H = ctx.H
|
||||
assert gy.is_contiguous()
|
||||
r, k, v, eew, ew, u = ctx.saved_tensors
|
||||
gr = torch.empty(
|
||||
(B, T, C),
|
||||
device=gy.device,
|
||||
requires_grad=False,
|
||||
dtype=torch.bfloat16,
|
||||
memory_format=torch.contiguous_format,
|
||||
) # .uniform_(-1, 1)
|
||||
gk = torch.empty(
|
||||
(B, T, C),
|
||||
device=gy.device,
|
||||
requires_grad=False,
|
||||
dtype=torch.bfloat16,
|
||||
memory_format=torch.contiguous_format,
|
||||
) # .uniform_(-1, 1)
|
||||
gv = torch.empty(
|
||||
(B, T, C),
|
||||
device=gy.device,
|
||||
requires_grad=False,
|
||||
dtype=torch.bfloat16,
|
||||
memory_format=torch.contiguous_format,
|
||||
) # .uniform_(-1, 1)
|
||||
gw = torch.empty(
|
||||
(B, C),
|
||||
device=gy.device,
|
||||
requires_grad=False,
|
||||
dtype=torch.bfloat16,
|
||||
memory_format=torch.contiguous_format,
|
||||
) # .uniform_(-1, 1)
|
||||
gu = torch.empty(
|
||||
(B, C),
|
||||
device=gy.device,
|
||||
requires_grad=False,
|
||||
dtype=torch.bfloat16,
|
||||
memory_format=torch.contiguous_format,
|
||||
) # .uniform_(-1, 1)
|
||||
wkv5_cuda.backward(B, T, C, H, r, k, v, eew, ew, u, gy, gr, gk, gv, gw, gu)
|
||||
gw = torch.sum(gw, 0).view(H, C // H)
|
||||
gu = torch.sum(gu, 0).view(H, C // H)
|
||||
return (None, None, None, None, gr, gk, gv, gw, gu)
|
||||
|
||||
|
||||
def RUN_CUDA_RWKV5(B, T, C, H, r, k, v, w, u):
|
||||
return WKV_5.apply(B, T, C, H, r, k, v, w, u)
|
||||
|
||||
|
||||
#################################################################
|
||||
class LoraLinear(nn.Module):
|
||||
def __init__(self, in_features: int, out_features: int, bias: bool):
|
||||
super().__init__()
|
||||
|
||||
self.weight = nn.Parameter(torch.empty((out_features, in_features)))
|
||||
assert bias == False, "Biased LoraLinear not supported"
|
||||
|
||||
r, alpha, dropout = (
|
||||
LORA_CONFIG["r"],
|
||||
LORA_CONFIG["alpha"],
|
||||
LORA_CONFIG["dropout"],
|
||||
)
|
||||
self.lora_A = nn.Parameter(torch.empty(r, in_features))
|
||||
self.lora_B = nn.Parameter(torch.empty(out_features, r))
|
||||
self.lora_dropout = nn.Dropout(dropout)
|
||||
self.scaling = alpha / r
|
||||
|
||||
nn.init.kaiming_uniform_(self.weight, a=math.sqrt(5))
|
||||
nn.init.kaiming_uniform_(self.lora_A, a=math.sqrt(5))
|
||||
nn.init.zeros_(self.lora_B)
|
||||
|
||||
def forward(self, x):
|
||||
return F.linear(x, self.weight) + self.scaling * F.linear(
|
||||
F.linear(self.lora_dropout(x), self.lora_A), self.lora_B
|
||||
)
|
||||
|
||||
|
||||
@functools.wraps(LoraLinear)
|
||||
def make_linear_att(*args, **kwargs):
|
||||
if "att" in LORA_CONFIG["parts"] and LORA_CONFIG["r"] > 0:
|
||||
return LoraLinear(*args, **kwargs)
|
||||
else:
|
||||
return nn.Linear(*args, **kwargs)
|
||||
|
||||
|
||||
@functools.wraps(LoraLinear)
|
||||
def make_linear_ffn(*args, **kwargs):
|
||||
if "ffn" in LORA_CONFIG["parts"] and LORA_CONFIG["r"] > 0:
|
||||
return LoraLinear(*args, **kwargs)
|
||||
else:
|
||||
return nn.Linear(*args, **kwargs)
|
||||
|
||||
|
||||
########################################################################################################
|
||||
|
||||
|
||||
class RWKV_TimeMix_RWKV5(MyModule):
|
||||
def __init__(self, args, layer_id):
|
||||
super().__init__()
|
||||
self.args = args
|
||||
self.layer_id = layer_id
|
||||
|
||||
self.head_size = args.head_size_a
|
||||
assert HEAD_SIZE == self.head_size # change HEAD_SIZE to match args.head_size_a
|
||||
self.n_head = args.dim_att // self.head_size
|
||||
assert args.dim_att % self.n_head == 0
|
||||
self.head_size_divisor = args.head_size_divisor
|
||||
|
||||
with torch.no_grad():
|
||||
ratio_0_to_1 = layer_id / (args.n_layer - 1) # 0 to 1
|
||||
ratio_1_to_almost0 = 1.0 - (layer_id / args.n_layer) # 1 to ~0
|
||||
ddd = torch.ones(1, 1, args.n_embd)
|
||||
for i in range(args.n_embd):
|
||||
ddd[0, 0, i] = i / args.n_embd
|
||||
|
||||
# fancy time_mix
|
||||
self.time_mix_k = nn.Parameter(torch.pow(ddd, ratio_1_to_almost0))
|
||||
self.time_mix_v = nn.Parameter(
|
||||
torch.pow(ddd, ratio_1_to_almost0) + 0.3 * ratio_0_to_1
|
||||
)
|
||||
self.time_mix_r = nn.Parameter(torch.pow(ddd, 0.5 * ratio_1_to_almost0))
|
||||
self.time_mix_g = nn.Parameter(torch.pow(ddd, 0.5 * ratio_1_to_almost0))
|
||||
|
||||
# fancy time_decay
|
||||
decay_speed = torch.ones(args.dim_att)
|
||||
for n in range(args.dim_att):
|
||||
decay_speed[n] = -6 + 5 * (n / (args.dim_att - 1)) ** (
|
||||
0.7 + 1.3 * ratio_0_to_1
|
||||
)
|
||||
self.time_decay = nn.Parameter(
|
||||
decay_speed.reshape(self.n_head, self.head_size)
|
||||
)
|
||||
# print(layer_id, self.time_decay.flatten()[:3].cpu().numpy(), '...', self.time_decay.flatten()[-3:].cpu().numpy())
|
||||
|
||||
tmp = torch.zeros(args.dim_att)
|
||||
for n in range(args.dim_att):
|
||||
zigzag = ((n + 1) % 3 - 1) * 0.1
|
||||
tmp[n] = ratio_0_to_1 * (1 - (n / (args.dim_att - 1))) + zigzag
|
||||
|
||||
self.time_faaaa = nn.Parameter(tmp.reshape(self.n_head, self.head_size))
|
||||
|
||||
self.time_shift = nn.ZeroPad2d((0, 0, 1, -1))
|
||||
|
||||
self.receptance = make_linear_att(args.n_embd, args.dim_att, bias=False)
|
||||
self.key = make_linear_att(args.n_embd, args.dim_att, bias=False)
|
||||
self.value = make_linear_att(args.n_embd, args.dim_att, bias=False)
|
||||
|
||||
self.output = nn.Linear(args.dim_att, args.n_embd, bias=False)
|
||||
self.gate = make_linear_att(args.n_embd, args.dim_att, bias=False)
|
||||
self.ln_x = nn.GroupNorm(self.n_head, args.dim_att)
|
||||
|
||||
@MyFunction
|
||||
def jit_func(self, x):
|
||||
B, T, C = x.size()
|
||||
|
||||
xx = self.time_shift(
|
||||
x
|
||||
) # Mix x with the previous timestep to produce xk, xv, xr
|
||||
xk = x * self.time_mix_k + xx * (1 - self.time_mix_k)
|
||||
xv = x * self.time_mix_v + xx * (1 - self.time_mix_v)
|
||||
xr = x * self.time_mix_r + xx * (1 - self.time_mix_r)
|
||||
xg = x * self.time_mix_g + xx * (1 - self.time_mix_g)
|
||||
|
||||
r = self.receptance(xr)
|
||||
k = self.key(xk)
|
||||
v = self.value(xv)
|
||||
g = F.silu(self.gate(xg))
|
||||
|
||||
return r, k, v, g
|
||||
|
||||
@MyFunction
|
||||
def jit_func_2(self, x, g):
|
||||
B, T, C = x.size()
|
||||
x = x.view(B * T, C)
|
||||
x = self.ln_x(x / self.head_size_divisor).view(B, T, C)
|
||||
x = self.output(x * g)
|
||||
return x
|
||||
|
||||
def forward(self, x):
|
||||
B, T, C = x.size()
|
||||
H = self.n_head
|
||||
r, k, v, g = self.jit_func(x)
|
||||
x = RUN_CUDA_RWKV5(B, T, C, H, r, k, v, w=self.time_decay, u=self.time_faaaa)
|
||||
|
||||
return self.jit_func_2(x, g)
|
||||
|
||||
|
||||
########################################################################################################
|
||||
|
||||
|
||||
class RWKV_ChannelMix(MyModule):
|
||||
def __init__(self, args, layer_id):
|
||||
super().__init__()
|
||||
self.args = args
|
||||
self.layer_id = layer_id
|
||||
self.time_shift = nn.ZeroPad2d((0, 0, 1, -1))
|
||||
|
||||
with torch.no_grad(): # fancy init of time_mix
|
||||
ratio_1_to_almost0 = 1.0 - (layer_id / args.n_layer) # 1 to ~0
|
||||
ddd = torch.ones(1, 1, args.n_embd)
|
||||
for i in range(args.n_embd):
|
||||
ddd[0, 0, i] = i / args.n_embd
|
||||
self.time_mix_k = nn.Parameter(torch.pow(ddd, ratio_1_to_almost0))
|
||||
self.time_mix_r = nn.Parameter(torch.pow(ddd, ratio_1_to_almost0))
|
||||
|
||||
self.key = make_linear_ffn(args.n_embd, args.dim_ffn, bias=False)
|
||||
self.receptance = make_linear_ffn(args.n_embd, args.n_embd, bias=False)
|
||||
self.value = make_linear_ffn(args.dim_ffn, args.n_embd, bias=False)
|
||||
|
||||
@MyFunction
|
||||
def forward(self, x):
|
||||
xx = self.time_shift(x)
|
||||
xk = x * self.time_mix_k + xx * (1 - self.time_mix_k)
|
||||
xr = x * self.time_mix_r + xx * (1 - self.time_mix_r)
|
||||
k = self.key(xk)
|
||||
k = torch.relu(k) ** 2
|
||||
kv = self.value(k)
|
||||
return torch.sigmoid(self.receptance(xr)) * kv
|
||||
|
||||
|
||||
class MishGLU(MyModule):
|
||||
def __init__(self, args, layer_id):
|
||||
super().__init__()
|
||||
self.args = args
|
||||
self.layer_id = layer_id
|
||||
self.time_shift = nn.ZeroPad2d((0, 0, 1, -1))
|
||||
|
||||
with torch.no_grad():
|
||||
ratio_1_to_almost0 = 1.0 - (layer_id / args.n_layer)
|
||||
|
||||
x = torch.ones(1, 1, args.n_embd)
|
||||
for i in range(args.n_embd):
|
||||
x[0, 0, i] = i / args.n_embd
|
||||
|
||||
self.time_mix_k = nn.Parameter(torch.pow(x, ratio_1_to_almost0))
|
||||
self.time_mix_r = nn.Parameter(torch.pow(x, ratio_1_to_almost0))
|
||||
self.aa = nn.Linear(args.n_embd, args.dim_ffn, bias=False)
|
||||
self.bb = nn.Linear(args.n_embd, args.dim_ffn, bias=False)
|
||||
self.value = nn.Linear(args.dim_ffn, args.n_embd, bias=False)
|
||||
|
||||
@MyFunction
|
||||
def forward(self, x):
|
||||
xx = self.time_shift(x)
|
||||
xa = x * self.time_mix_k + xx * (1 - self.time_mix_k)
|
||||
xb = x * self.time_mix_r + xx * (1 - self.time_mix_r)
|
||||
a = self.aa(xa)
|
||||
b = self.bb(xb)
|
||||
return self.value(a * F.mish(b))
|
||||
|
||||
|
||||
########################################################################################################
|
||||
# The RWKV Model with our blocks
|
||||
########################################################################################################
|
||||
|
||||
|
||||
class Block(nn.Module):
|
||||
def __init__(self, args, layer_id):
|
||||
super().__init__()
|
||||
self.args = args
|
||||
self.layer_id = layer_id
|
||||
|
||||
self.ln1 = nn.LayerNorm(args.n_embd)
|
||||
self.ln2 = nn.LayerNorm(args.n_embd)
|
||||
|
||||
if self.layer_id == 0:
|
||||
self.ln0 = nn.LayerNorm(args.n_embd)
|
||||
if args.my_pos_emb > 0:
|
||||
self.pos_emb_x = nn.Parameter(
|
||||
torch.zeros((1, args.my_pos_emb, args.n_embd))
|
||||
)
|
||||
self.pos_emb_y = nn.Parameter(
|
||||
torch.zeros((args.my_pos_emb, 1, args.n_embd))
|
||||
)
|
||||
|
||||
if self.layer_id == 0 and self.args.pre_ffn > 0:
|
||||
self.ffnPre = RWKV_ChannelMix(args, 0)
|
||||
else:
|
||||
self.att = RWKV_TimeMix_RWKV5(args, layer_id)
|
||||
|
||||
if "g" in os.environ["RWKV_MY_TESTING"]:
|
||||
self.ffn = MishGLU(args, layer_id)
|
||||
else:
|
||||
self.ffn = RWKV_ChannelMix(args, layer_id)
|
||||
|
||||
if args.tiny_att_dim > 0 and self.layer_id == args.tiny_att_layer:
|
||||
self.tiny_ln = nn.LayerNorm(args.n_embd)
|
||||
self.tiny_q = nn.Linear(args.n_embd, args.tiny_att_dim, bias=False)
|
||||
self.tiny_k = nn.Linear(args.n_embd, args.tiny_att_dim, bias=False)
|
||||
self.tiny_v = nn.Linear(args.n_embd, args.n_embd, bias=False)
|
||||
self.register_buffer(
|
||||
"tiny_mask", torch.tril(torch.ones(args.ctx_len, args.ctx_len))
|
||||
)
|
||||
|
||||
if args.dropout > 0:
|
||||
self.drop0 = nn.Dropout(p=args.dropout)
|
||||
self.drop1 = nn.Dropout(p=args.dropout)
|
||||
|
||||
def forward(self, x, x_emb=None):
|
||||
args = self.args
|
||||
B, T, C = x.size()
|
||||
if self.layer_id == 0:
|
||||
x = self.ln0(x)
|
||||
if args.my_pos_emb > 0:
|
||||
pos_emb = (self.pos_emb_x + self.pos_emb_y).reshape(T + 1, -1)[:-1, :]
|
||||
x = x + pos_emb
|
||||
|
||||
if self.args.dropout == 0:
|
||||
if self.layer_id == 0 and args.pre_ffn > 0:
|
||||
x = x + self.ffnPre(self.ln1(x))
|
||||
else:
|
||||
x = x + self.att(self.ln1(x))
|
||||
x = x + self.ffn(self.ln2(x))
|
||||
else:
|
||||
if self.layer_id == 0 and args.pre_ffn > 0:
|
||||
x = self.drop0(x + self.ffnPre(self.ln1(x)))
|
||||
else:
|
||||
x = self.drop0(x + self.att(self.ln1(x)))
|
||||
x = self.drop1(x + self.ffn(self.ln2(x)))
|
||||
|
||||
if args.tiny_att_dim > 0 and self.layer_id == args.tiny_att_layer:
|
||||
xx = self.tiny_ln(x)
|
||||
q = self.tiny_q(xx)[:, :T, :]
|
||||
k = self.tiny_k(xx)[:, :T, :]
|
||||
c = (q @ k.transpose(-2, -1)) * (args.tiny_att_dim ** (-0.5))
|
||||
c = c.masked_fill(self.tiny_mask[:T, :T] == 0, 0)
|
||||
x = x + c @ self.tiny_v(x_emb)
|
||||
return x
|
||||
|
||||
|
||||
class L2Wrap(torch.autograd.Function):
|
||||
@staticmethod
|
||||
def forward(ctx, loss, y):
|
||||
ctx.save_for_backward(y)
|
||||
return loss
|
||||
|
||||
@staticmethod
|
||||
def backward(ctx, grad_output):
|
||||
y = ctx.saved_tensors[0]
|
||||
# to encourage the logits to be close to 0
|
||||
factor = 1e-4 / (y.shape[0] * y.shape[1])
|
||||
maxx, ids = torch.max(y, -1, keepdim=True)
|
||||
gy = torch.zeros_like(y)
|
||||
gy.scatter_(-1, ids, maxx * factor)
|
||||
return (grad_output, gy)
|
||||
|
||||
|
||||
class RWKV(pl.LightningModule):
|
||||
def __init__(self, args):
|
||||
super().__init__()
|
||||
self.args = args
|
||||
if not hasattr(args, "dim_att"):
|
||||
args.dim_att = args.n_embd
|
||||
if not hasattr(args, "dim_ffn"):
|
||||
args.dim_ffn = args.n_embd * 4
|
||||
if not hasattr(args, "tiny_att_layer"):
|
||||
args.tiny_att_layer = -1
|
||||
if not hasattr(args, "tiny_att_dim"):
|
||||
args.tiny_att_dim = -1
|
||||
assert args.n_embd % 32 == 0
|
||||
assert args.dim_att % 32 == 0
|
||||
assert args.dim_ffn % 32 == 0
|
||||
|
||||
self.emb = nn.Embedding(args.vocab_size, args.n_embd)
|
||||
|
||||
self.blocks = nn.ModuleList([Block(args, i) for i in range(args.n_layer)])
|
||||
|
||||
self.ln_out = nn.LayerNorm(args.n_embd)
|
||||
self.head = nn.Linear(args.n_embd, args.vocab_size, bias=False)
|
||||
|
||||
if args.head_qk > 0:
|
||||
self.head_q = nn.Linear(args.n_embd, args.head_qk, bias=False)
|
||||
self.head_k = nn.Linear(args.n_embd, args.head_qk, bias=False)
|
||||
self.register_buffer(
|
||||
"copy_mask", torch.tril(torch.ones(args.ctx_len, args.ctx_len))
|
||||
)
|
||||
if args.dropout > 0:
|
||||
self.drop0 = nn.Dropout(p=args.dropout)
|
||||
|
||||
def configure_optimizers(self):
|
||||
args = self.args
|
||||
|
||||
lr_decay = set()
|
||||
lr_1x = set()
|
||||
lr_2x = set()
|
||||
lr_3x = set()
|
||||
for n, p in self.named_parameters():
|
||||
if ("time_mix" in n) and (args.layerwise_lr > 0):
|
||||
if args.my_pile_stage == 2:
|
||||
lr_2x.add(n)
|
||||
else:
|
||||
lr_1x.add(n)
|
||||
elif ("time_decay" in n) and (args.layerwise_lr > 0):
|
||||
if args.my_pile_stage == 2:
|
||||
lr_3x.add(n)
|
||||
else:
|
||||
lr_2x.add(n)
|
||||
elif ("time_faaaa" in n) and (args.layerwise_lr > 0):
|
||||
if args.my_pile_stage == 2:
|
||||
lr_2x.add(n)
|
||||
else:
|
||||
lr_1x.add(n)
|
||||
elif ("time_first" in n) and (args.layerwise_lr > 0):
|
||||
lr_3x.add(n)
|
||||
elif (len(p.squeeze().shape) >= 2) and (args.weight_decay > 0):
|
||||
lr_decay.add(n)
|
||||
else:
|
||||
lr_1x.add(n)
|
||||
|
||||
lr_decay = sorted(list(lr_decay))
|
||||
lr_1x = sorted(list(lr_1x))
|
||||
lr_2x = sorted(list(lr_2x))
|
||||
lr_3x = sorted(list(lr_3x))
|
||||
# print('decay', lr_decay)
|
||||
# print('1x', lr_1x)
|
||||
# print('2x', lr_2x)
|
||||
# print('3x', lr_3x)
|
||||
param_dict = {n: p for n, p in self.named_parameters()}
|
||||
|
||||
if args.layerwise_lr > 0:
|
||||
if args.my_pile_stage == 2:
|
||||
optim_groups = [
|
||||
{
|
||||
"params": [param_dict[n] for n in lr_1x],
|
||||
"weight_decay": 0.0,
|
||||
"my_lr_scale": 1.0,
|
||||
},
|
||||
{
|
||||
"params": [param_dict[n] for n in lr_2x],
|
||||
"weight_decay": 0.0,
|
||||
"my_lr_scale": 5.0,
|
||||
}, # test: 2e-3 / args.lr_init},
|
||||
{
|
||||
"params": [param_dict[n] for n in lr_3x],
|
||||
"weight_decay": 0.0,
|
||||
"my_lr_scale": 5.0,
|
||||
}, # test: 3e-3 / args.lr_init},
|
||||
]
|
||||
else:
|
||||
optim_groups = [
|
||||
{
|
||||
"params": [param_dict[n] for n in lr_1x],
|
||||
"weight_decay": 0.0,
|
||||
"my_lr_scale": 1.0,
|
||||
},
|
||||
{
|
||||
"params": [param_dict[n] for n in lr_2x],
|
||||
"weight_decay": 0.0,
|
||||
"my_lr_scale": 2.0,
|
||||
},
|
||||
{
|
||||
"params": [param_dict[n] for n in lr_3x],
|
||||
"weight_decay": 0.0,
|
||||
"my_lr_scale": 3.0,
|
||||
},
|
||||
]
|
||||
else:
|
||||
optim_groups = [
|
||||
{
|
||||
"params": [param_dict[n] for n in lr_1x],
|
||||
"weight_decay": 0.0,
|
||||
"my_lr_scale": 1.0,
|
||||
}
|
||||
]
|
||||
|
||||
if args.weight_decay > 0:
|
||||
optim_groups += [
|
||||
{
|
||||
"params": [param_dict[n] for n in lr_decay],
|
||||
"weight_decay": args.weight_decay,
|
||||
"my_lr_scale": 1.0,
|
||||
}
|
||||
]
|
||||
if self.deepspeed_offload:
|
||||
return DeepSpeedCPUAdam(
|
||||
optim_groups,
|
||||
lr=self.args.lr_init,
|
||||
betas=self.args.betas,
|
||||
eps=self.args.adam_eps,
|
||||
bias_correction=True,
|
||||
adamw_mode=True,
|
||||
amsgrad=False,
|
||||
)
|
||||
return FusedAdam(
|
||||
optim_groups,
|
||||
lr=self.args.lr_init,
|
||||
betas=self.args.betas,
|
||||
eps=self.args.adam_eps,
|
||||
bias_correction=True,
|
||||
adam_w_mode=True,
|
||||
amsgrad=False,
|
||||
)
|
||||
else:
|
||||
if self.deepspeed_offload:
|
||||
return DeepSpeedCPUAdam(
|
||||
optim_groups,
|
||||
lr=self.args.lr_init,
|
||||
betas=self.args.betas,
|
||||
eps=self.args.adam_eps,
|
||||
bias_correction=True,
|
||||
adamw_mode=False,
|
||||
weight_decay=0,
|
||||
amsgrad=False,
|
||||
)
|
||||
return FusedAdam(
|
||||
optim_groups,
|
||||
lr=self.args.lr_init,
|
||||
betas=self.args.betas,
|
||||
eps=self.args.adam_eps,
|
||||
bias_correction=True,
|
||||
adam_w_mode=False,
|
||||
weight_decay=0,
|
||||
amsgrad=False,
|
||||
)
|
||||
# return ZeroOneAdam(optim_groups, lr=self.args.lr_init, betas=self.args.betas, eps=self.args.adam_eps, bias_correction=True, weight_decay=0, amsgrad=False, cuda_aware=False)
|
||||
|
||||
@property
|
||||
def deepspeed_offload(self) -> bool:
|
||||
strategy = self.trainer.strategy
|
||||
if isinstance(strategy, DeepSpeedStrategy):
|
||||
cfg = strategy.config["zero_optimization"]
|
||||
return cfg.get("offload_optimizer") or cfg.get("offload_param")
|
||||
return False
|
||||
|
||||
def forward(self, idx):
|
||||
args = self.args
|
||||
B, T = idx.size()
|
||||
assert T <= args.ctx_len, "Cannot forward, model ctx_len is exhausted."
|
||||
|
||||
x = self.emb(idx)
|
||||
x_emb = x
|
||||
|
||||
if args.dropout > 0:
|
||||
x = self.drop0(x)
|
||||
if args.tiny_att_dim > 0:
|
||||
for block in self.blocks:
|
||||
if args.grad_cp == 1:
|
||||
if args.lora:
|
||||
x = torch_checkpoint(block, x, x_emb, use_reentrant=False)
|
||||
else:
|
||||
x = deepspeed.checkpointing.checkpoint(block, x, x_emb)
|
||||
else:
|
||||
x = block(x, x_emb)
|
||||
else:
|
||||
for block in self.blocks:
|
||||
if args.grad_cp == 1:
|
||||
if args.lora:
|
||||
x = torch_checkpoint(block, x, x_emb, use_reentrant=False)
|
||||
else:
|
||||
x = deepspeed.checkpointing.checkpoint(block, x)
|
||||
else:
|
||||
x = block(x)
|
||||
|
||||
x = self.ln_out(x)
|
||||
|
||||
if args.head_qk > 0:
|
||||
q = self.head_q(x)[:, :T, :]
|
||||
k = self.head_k(x)[:, :T, :]
|
||||
c = (q @ k.transpose(-2, -1)) * (1.0 / args.head_qk)
|
||||
c = c.masked_fill(self.copy_mask[:T, :T] == 0, 0)
|
||||
|
||||
if "32" in os.environ["RWKV_FLOAT_MODE"]:
|
||||
c = c @ F.one_hot(idx, num_classes=args.vocab_size)
|
||||
elif os.environ["RWKV_FLOAT_MODE"] == "fp16":
|
||||
c = c @ F.one_hot(idx, num_classes=args.vocab_size).half()
|
||||
elif os.environ["RWKV_FLOAT_MODE"] == "bf16":
|
||||
c = c @ F.one_hot(idx, num_classes=args.vocab_size).bfloat16()
|
||||
|
||||
x = self.head(x) + c
|
||||
else:
|
||||
x = self.head(x)
|
||||
|
||||
return x
|
||||
|
||||
def training_step(self, batch, batch_idx):
|
||||
args = self.args
|
||||
if args.my_qa_mask != 1:
|
||||
idx, targets = batch
|
||||
logits = self(idx)
|
||||
loss = F.cross_entropy(logits.view(-1, logits.size(-1)), targets.view(-1))
|
||||
# if '0' in os.environ["RWKV_MY_TESTING"]:
|
||||
# print('logits', logits)
|
||||
# torch.set_printoptions(threshold=10000)
|
||||
# print('idx', idx)
|
||||
# exit(0)
|
||||
else:
|
||||
idx, targets, mask = batch
|
||||
mask = mask.view(-1)
|
||||
sum_mask = torch.sum(mask).item()
|
||||
# if sum_mask == 0:
|
||||
# return torch.tensor([0.0], requires_grad=True)
|
||||
|
||||
logits = self(idx)
|
||||
if sum_mask == mask.shape[0]:
|
||||
loss = F.cross_entropy(
|
||||
logits.view(-1, logits.size(-1)), targets.view(-1)
|
||||
)
|
||||
# print('rank', self.global_rank, 'loss', loss.item())
|
||||
else:
|
||||
loss = F.cross_entropy(
|
||||
logits.view(-1, logits.size(-1)), targets.view(-1), reduction="none"
|
||||
)
|
||||
# loss_raw = loss
|
||||
loss = torch.sum(loss * mask) / sum_mask
|
||||
|
||||
# torch.set_printoptions(threshold=10000)
|
||||
# if True: #self.global_rank == 1:
|
||||
# tmp = ''
|
||||
# sss = 0
|
||||
# ccc = 0
|
||||
# for i in range(mask.shape[0]):
|
||||
# if mask[i] > 0:
|
||||
# tmp += str(idx.view(-1)[i].item()) + ','
|
||||
# sss += loss_raw.view(-1)[i].float().item()
|
||||
# ccc += 1
|
||||
# print('rank', self.global_rank, 'loss', loss.item(), 'lavg', sss / ccc)#, 'tmp', tmp, 'input', idx)
|
||||
return L2Wrap.apply(loss, logits)
|
||||
|
||||
def training_step_end(self, batch_parts):
|
||||
if pl.__version__[0] != "2":
|
||||
all = self.all_gather(batch_parts)
|
||||
if self.trainer.is_global_zero:
|
||||
self.trainer.my_loss_all = all
|
||||
|
||||
def generate_init_weight(self):
|
||||
print(
|
||||
f"""
|
||||
############################################################################
|
||||
#
|
||||
# Init model weight (slow for large models)...
|
||||
#
|
||||
############################################################################
|
||||
"""
|
||||
)
|
||||
m = {}
|
||||
for n in self.state_dict():
|
||||
p = self.state_dict()[n]
|
||||
shape = p.shape
|
||||
|
||||
gain = 1.0
|
||||
scale = 1.0
|
||||
if (
|
||||
"ln_" in n
|
||||
or ".ln" in n
|
||||
or "time_" in n
|
||||
or "_mask" in n
|
||||
or "pos_emb" in n
|
||||
or ".mask." in n
|
||||
):
|
||||
if "ln_x.weight" in n:
|
||||
layer_scale = (1 + int(n.split(".")[1])) / self.args.n_layer
|
||||
m[n] = (p * 0.0) + (layer_scale**0.7)
|
||||
else:
|
||||
m[n] = p
|
||||
else:
|
||||
if n == "emb.weight":
|
||||
scale = -1 * self.args.lr_init
|
||||
else:
|
||||
if shape[0] > shape[1]:
|
||||
gain = math.sqrt(shape[0] / shape[1])
|
||||
|
||||
zero = [
|
||||
".att.output.",
|
||||
".ffn.value.",
|
||||
".ffn.receptance.",
|
||||
".ffnPre.value.",
|
||||
".ffnPre.receptance.",
|
||||
"head_q.",
|
||||
".oo.",
|
||||
".rr.",
|
||||
]
|
||||
|
||||
for kk in zero:
|
||||
if kk in n:
|
||||
scale = 0
|
||||
if n == "head.weight":
|
||||
scale = 0.5
|
||||
if "head_k." in n:
|
||||
scale = 0.1
|
||||
if "head_q." in n:
|
||||
scale = 0
|
||||
|
||||
print(
|
||||
f"{str(shape[0]).ljust(5)} {str(shape[1]).ljust(5)} {str(scale).ljust(4)} {n}"
|
||||
)
|
||||
|
||||
if self.args.accelerator.upper() == "GPU":
|
||||
m[n] = torch.empty((shape[0], shape[1]), device="cuda")
|
||||
else:
|
||||
m[n] = torch.empty((shape[0], shape[1]))
|
||||
|
||||
if scale == 0:
|
||||
nn.init.zeros_(m[n])
|
||||
elif scale < 0:
|
||||
nn.init.uniform_(m[n], a=scale, b=-scale)
|
||||
else:
|
||||
nn.init.orthogonal_(m[n], gain=gain * scale)
|
||||
|
||||
m[n] = m[n].cpu()
|
||||
if os.environ["RWKV_FLOAT_MODE"] == "fp16":
|
||||
m[n] = m[n].half()
|
||||
elif os.environ["RWKV_FLOAT_MODE"] == "bf16":
|
||||
m[n] = m[n].bfloat16()
|
||||
|
||||
# if n == "emb.weight":
|
||||
# print(m[n])
|
||||
|
||||
gc.collect()
|
||||
torch.cuda.empty_cache()
|
||||
return m
|
310
finetune/lora/v5/src/trainer.py
vendored
Normal file
310
finetune/lora/v5/src/trainer.py
vendored
Normal file
@ -0,0 +1,310 @@
|
||||
import os, math, time, datetime, subprocess
|
||||
import torch
|
||||
from torch.utils.data import DataLoader
|
||||
import pytorch_lightning as pl
|
||||
from pytorch_lightning.utilities import rank_zero_info, rank_zero_only
|
||||
from .model import LORA_CONFIG
|
||||
|
||||
|
||||
def my_save(args, trainer, dd, ff):
|
||||
if "14b-run1" in ff:
|
||||
fn = ff.split("/")[-1]
|
||||
fff = "/dev/shm/" + fn
|
||||
torch.save(dd, fff)
|
||||
subprocess.Popen(f" aws s3 mv {fff} s3://rwkv-14b-4k/{fn} --quiet", shell=True)
|
||||
elif ("world/14b" in ff) or ("world/7b" in ff):
|
||||
aa = ff.split("/")[1]
|
||||
fn = ff.split("/")[-1]
|
||||
fff = f"/dev/shm/{aa}-{fn}"
|
||||
torch.save(dd, fff)
|
||||
subprocess.Popen(
|
||||
f" aws s3 mv {fff} s3://rwkv-world/{aa}-{fn} --quiet", shell=True
|
||||
)
|
||||
else:
|
||||
if "deepspeed_stage_3" in args.strategy:
|
||||
trainer.save_checkpoint(ff, weights_only=True)
|
||||
else:
|
||||
torch.save(dd, ff)
|
||||
|
||||
|
||||
class train_callback(pl.Callback):
|
||||
def __init__(self, args):
|
||||
super().__init__()
|
||||
self.args = args
|
||||
|
||||
def on_train_batch_start(self, trainer, pl_module, batch, batch_idx):
|
||||
args = self.args
|
||||
# if args.cuda_cleanup > 0:
|
||||
# torch.cuda.empty_cache()
|
||||
real_step = trainer.global_step + args.epoch_begin * args.epoch_steps
|
||||
|
||||
# LR schedule
|
||||
w_step = args.warmup_steps
|
||||
if args.lr_final == args.lr_init or args.epoch_count == 0:
|
||||
lr = args.lr_init
|
||||
else:
|
||||
decay_step = real_step - args.my_pile_edecay * args.epoch_steps
|
||||
decay_total = (args.epoch_count - args.my_pile_edecay) * args.epoch_steps
|
||||
progress = (decay_step - w_step + 1) / (decay_total - w_step)
|
||||
progress = min(1, max(0, progress))
|
||||
|
||||
if args.lr_final == 0 or args.lr_init == 0: # linear decay
|
||||
lr = args.lr_init + (args.lr_final - args.lr_init) * progress
|
||||
else: # exp decay
|
||||
lr = args.lr_init * math.exp(
|
||||
math.log(args.lr_final / args.lr_init) * pow(progress, 1)
|
||||
)
|
||||
# if trainer.is_global_zero:
|
||||
# print(trainer.global_step, decay_step, decay_total, w_step, progress, lr)
|
||||
|
||||
if args.my_exit_tokens != 0: # cosine decay
|
||||
real_tokens = real_step * args.ctx_len * args.real_bsz
|
||||
warmup_tokens = w_step * args.ctx_len * args.real_bsz
|
||||
progress = (real_tokens - warmup_tokens) / (
|
||||
abs(args.my_exit_tokens) - warmup_tokens
|
||||
)
|
||||
progress = max(0, min(1, progress))
|
||||
lr_final_factor = args.lr_final / args.lr_init
|
||||
lr_mult = (0.5 + lr_final_factor / 2) + (
|
||||
0.5 - lr_final_factor / 2
|
||||
) * math.cos(math.pi * progress)
|
||||
if args.my_exit_tokens > 0:
|
||||
lr = args.lr_init * lr_mult
|
||||
else:
|
||||
lr = (lr + args.lr_init * lr_mult) / 2
|
||||
if progress >= 1:
|
||||
if (trainer.is_global_zero) or ("deepspeed_stage_3" in args.strategy):
|
||||
my_save(
|
||||
args,
|
||||
trainer,
|
||||
pl_module.state_dict(),
|
||||
f"{args.proj_dir}/rwkv-final.pth",
|
||||
)
|
||||
exit(0)
|
||||
if trainer.global_step < w_step:
|
||||
lr = lr * (0.2 + 0.8 * trainer.global_step / w_step)
|
||||
|
||||
if args.weight_decay_final > 0:
|
||||
wd_now = args.weight_decay * math.exp(
|
||||
math.log(args.weight_decay_final / args.weight_decay) * progress
|
||||
)
|
||||
else:
|
||||
wd_now = args.weight_decay
|
||||
|
||||
for param_group in trainer.optimizers[0].param_groups:
|
||||
if param_group["weight_decay"] > 0:
|
||||
param_group["weight_decay"] = wd_now
|
||||
if args.layerwise_lr > 0:
|
||||
param_group["lr"] = lr * param_group["my_lr_scale"]
|
||||
# print(param_group["lr"], param_group["my_lr_scale"])
|
||||
else:
|
||||
param_group["lr"] = lr
|
||||
|
||||
trainer.my_lr = lr
|
||||
trainer.my_wd = wd_now
|
||||
# rank_zero_info(f"{real_step} {lr}")
|
||||
|
||||
if trainer.global_step == 0:
|
||||
if trainer.is_global_zero: # logging
|
||||
trainer.my_loss_sum = 0
|
||||
trainer.my_loss_count = 0
|
||||
trainer.my_log = open(args.proj_dir + "/train_log.txt", "a")
|
||||
trainer.my_log.write(
|
||||
f"NEW RUN {args.my_timestamp}\n{vars(self.args)}\n"
|
||||
)
|
||||
try:
|
||||
print(f"\n{trainer.strategy.config}\n")
|
||||
trainer.my_log.write(f"{trainer.strategy.config}\n")
|
||||
except:
|
||||
pass
|
||||
trainer.my_log.flush()
|
||||
if len(args.wandb) > 0:
|
||||
print("Login to wandb...")
|
||||
import wandb
|
||||
|
||||
wandb.init(
|
||||
project=args.wandb,
|
||||
name=args.run_name + " " + args.my_timestamp,
|
||||
config=args,
|
||||
save_code=False,
|
||||
)
|
||||
trainer.my_wandb = wandb
|
||||
|
||||
def on_train_batch_end(self, trainer, pl_module, outputs, batch, batch_idx):
|
||||
args = self.args
|
||||
token_per_step = args.ctx_len * args.real_bsz
|
||||
real_step = trainer.global_step + args.epoch_begin * args.epoch_steps
|
||||
if trainer.is_global_zero: # logging
|
||||
t_now = time.time_ns()
|
||||
kt_s = 0
|
||||
try:
|
||||
t_cost = (t_now - trainer.my_time_ns) / 1e9
|
||||
kt_s = token_per_step / t_cost / 1000
|
||||
self.log("REAL it/s", 1.0 / t_cost, prog_bar=True, on_step=True)
|
||||
self.log("Kt/s", kt_s, prog_bar=True, on_step=True)
|
||||
except:
|
||||
pass
|
||||
trainer.my_time_ns = t_now
|
||||
if pl.__version__[0] == "2":
|
||||
trainer.my_loss = outputs["loss"]
|
||||
else:
|
||||
trainer.my_loss = trainer.my_loss_all.float().mean().item()
|
||||
trainer.my_loss_sum += trainer.my_loss
|
||||
trainer.my_loss_count += 1
|
||||
trainer.my_epoch_loss = trainer.my_loss_sum / trainer.my_loss_count
|
||||
self.log("lr", trainer.my_lr, prog_bar=True, on_step=True)
|
||||
self.log("loss", trainer.my_epoch_loss, prog_bar=True, on_step=True)
|
||||
# self.log("s", real_step, prog_bar=True, on_step=True)
|
||||
|
||||
if len(args.wandb) > 0:
|
||||
lll = {
|
||||
"loss": trainer.my_loss,
|
||||
"lr": trainer.my_lr,
|
||||
"wd": trainer.my_wd,
|
||||
"Gtokens": real_step * token_per_step / 1e9,
|
||||
}
|
||||
if kt_s > 0:
|
||||
lll["kt/s"] = kt_s
|
||||
trainer.my_wandb.log(lll, step=int(real_step))
|
||||
if (trainer.is_global_zero) or (
|
||||
"deepspeed_stage_3" in args.strategy
|
||||
): # save pth
|
||||
if args.magic_prime > 0:
|
||||
expand_factor = 2 if args.my_qa_mask > 0 else 1
|
||||
if int(real_step) == int(
|
||||
args.magic_prime * expand_factor // args.real_bsz
|
||||
) - 1 + int(args.my_random_steps):
|
||||
to_save_dict = pl_module.state_dict()
|
||||
my_save(
|
||||
args,
|
||||
trainer,
|
||||
to_save_dict,
|
||||
f"{args.proj_dir}/rwkv-final.pth",
|
||||
)
|
||||
# if args.batch_save==batch_idx :
|
||||
# to_save_dict = pl_module.state_dict()
|
||||
# for name, state in to_save_dict.items():
|
||||
# if 'img' in name:
|
||||
# to_save_dict[name] = state
|
||||
# try:
|
||||
# my_save(
|
||||
# args, trainer,
|
||||
# to_save_dict,
|
||||
# f"{args.proj_dir}/rwkv-{args.epoch_begin + trainer.current_epoch}-{batch_idx}.pth",
|
||||
# )
|
||||
# except Exception as e:
|
||||
# print('Error\n\n', e, '\n\n')
|
||||
|
||||
def on_train_epoch_start(self, trainer, pl_module):
|
||||
args = self.args
|
||||
if pl.__version__[0] == "2":
|
||||
dataset = trainer.train_dataloader.dataset
|
||||
else:
|
||||
dataset = trainer.train_dataloader.dataset.datasets
|
||||
assert "MyDataset" in str(dataset)
|
||||
dataset.global_rank = trainer.global_rank
|
||||
dataset.real_epoch = int(args.epoch_begin + trainer.current_epoch)
|
||||
dataset.world_size = trainer.world_size
|
||||
# print(f'########## world_size {dataset.world_size} global_rank {dataset.global_rank} real_epoch {dataset.real_epoch} ##########')
|
||||
|
||||
def on_train_epoch_end(self, trainer, pl_module):
|
||||
args = self.args
|
||||
to_save_dict = {}
|
||||
if (trainer.is_global_zero) or (
|
||||
"deepspeed_stage_3" in args.strategy
|
||||
): # save pth
|
||||
if (
|
||||
args.epoch_save > 0 and trainer.current_epoch % args.epoch_save == 0
|
||||
) or (trainer.current_epoch == args.epoch_count - 1):
|
||||
if args.data_type == "wds_img":
|
||||
raw_dict = pl_module.state_dict()
|
||||
for k in raw_dict:
|
||||
if k.startswith("encoder.") or k.startswith("decoder."):
|
||||
to_save_dict[k] = raw_dict[k]
|
||||
else:
|
||||
to_save_dict = pl_module.state_dict()
|
||||
|
||||
if args.data_type == "img" and not args.lora:
|
||||
for name, state in to_save_dict.items():
|
||||
if "img" in name:
|
||||
to_save_dict[name] = state
|
||||
|
||||
if args.lora:
|
||||
enable_time_finetune = "time" in LORA_CONFIG["parts"]
|
||||
enable_ln_finetune = "ln" in LORA_CONFIG["parts"]
|
||||
lora_dict = {}
|
||||
for name, state in to_save_dict.items():
|
||||
if "img" in name:
|
||||
lora_dict[name] = state
|
||||
if (
|
||||
".lora_" in name
|
||||
or (enable_time_finetune and ".time_" in name)
|
||||
or (enable_ln_finetune and ".ln" in name)
|
||||
):
|
||||
lora_dict[name] = state
|
||||
to_save_dict = lora_dict
|
||||
|
||||
try:
|
||||
my_save(
|
||||
args,
|
||||
trainer,
|
||||
to_save_dict,
|
||||
f"{args.proj_dir}/rwkv-{args.epoch_begin + trainer.current_epoch}.pth",
|
||||
)
|
||||
except Exception as e:
|
||||
print("Error\n\n", e, "\n\n")
|
||||
|
||||
if trainer.is_global_zero: # logging
|
||||
trainer.my_log.write(
|
||||
f"{args.epoch_begin + trainer.current_epoch} {trainer.my_epoch_loss:.6f} {math.exp(trainer.my_epoch_loss):.4f} {trainer.my_lr:.8f} {datetime.datetime.now()} {trainer.current_epoch}\n"
|
||||
)
|
||||
trainer.my_log.flush()
|
||||
|
||||
trainer.my_loss_sum = 0
|
||||
trainer.my_loss_count = 0
|
||||
if (args.epoch_begin + trainer.current_epoch) >= args.my_exit:
|
||||
exit(0)
|
||||
|
||||
|
||||
@rank_zero_only
|
||||
def generate_init_weight(model, init_weight_name):
|
||||
mm = model.generate_init_weight()
|
||||
|
||||
if model.args.my_pile_stage == 1:
|
||||
if len(model.args.load_model) > 0:
|
||||
print(f"Combine weights from {model.args.load_model}...")
|
||||
load_dict = torch.load(model.args.load_model, map_location="cpu")
|
||||
for k in load_dict:
|
||||
try:
|
||||
assert k in mm
|
||||
except:
|
||||
print("missing", k)
|
||||
exit(0)
|
||||
src = load_dict[k]
|
||||
try:
|
||||
mm[k] = src.reshape(mm[k].shape)
|
||||
except:
|
||||
tmp = mm[k].squeeze().clone()
|
||||
print(k, src.shape, "-->", mm[k].shape)
|
||||
ss = src.shape[0]
|
||||
dd = tmp.shape[0]
|
||||
for i in range(dd):
|
||||
pos = i / dd * ss
|
||||
if pos >= ss - 1:
|
||||
tmp[i] = src[ss - 1]
|
||||
else:
|
||||
p0 = int(math.floor(pos))
|
||||
ii = pos - p0
|
||||
tmp[i] = src[p0] * (1 - ii) + src[p0 + 1] * (ii)
|
||||
mm[k] = tmp.reshape(mm[k].shape)
|
||||
sss = src.squeeze().float().cpu().numpy()
|
||||
print(sss[:10], "...", sss[-10:])
|
||||
mmm = mm[k].squeeze().float().cpu().numpy()
|
||||
print(mmm[:10], "...", mmm[-10:])
|
||||
|
||||
print(f"Save to {init_weight_name}...")
|
||||
torch.save(mm, init_weight_name)
|
||||
|
||||
if model.args.my_pile_stage == 1:
|
||||
print("Done. Now go for stage 2.")
|
||||
exit(0)
|
139
finetune/lora/v5/src/utils.py
vendored
Normal file
139
finetune/lora/v5/src/utils.py
vendored
Normal file
@ -0,0 +1,139 @@
|
||||
import json, time, random, os
|
||||
import numpy as np
|
||||
import torch
|
||||
from torch.nn import functional as F
|
||||
|
||||
time_slot = {}
|
||||
time_ref = time.time_ns()
|
||||
|
||||
|
||||
def record_time(name):
|
||||
if name not in time_slot:
|
||||
time_slot[name] = 1e20
|
||||
tt = (time.time_ns() - time_ref) / 1e9
|
||||
if tt < time_slot[name]:
|
||||
time_slot[name] = tt
|
||||
|
||||
|
||||
class TOKENIZER:
|
||||
def __init__(self, WORD_NAME, UNKNOWN_CHAR="\ue083"):
|
||||
if "list" in str(type(WORD_NAME)):
|
||||
self.charMode = False
|
||||
if WORD_NAME[0] == WORD_NAME[1]:
|
||||
from transformers import PreTrainedTokenizerFast
|
||||
|
||||
self.tokenizer = PreTrainedTokenizerFast(tokenizer_file=WORD_NAME[0])
|
||||
else:
|
||||
from transformers import GPT2TokenizerFast
|
||||
|
||||
self.tokenizer = GPT2TokenizerFast(WORD_NAME[0], WORD_NAME[1])
|
||||
self.vocab_size = len(self.tokenizer)
|
||||
else:
|
||||
self.charMode = True
|
||||
with open(WORD_NAME + ".json", "r", encoding="utf-16") as result_file:
|
||||
self.word_table = json.load(result_file)
|
||||
|
||||
self.vocab_size = len(self.word_table)
|
||||
|
||||
self.stoi = {v: int(k) for k, v in self.word_table.items()}
|
||||
self.itos = {int(k): v for k, v in self.word_table.items()}
|
||||
|
||||
self.UNKNOWN_CHAR = self.stoi[UNKNOWN_CHAR]
|
||||
|
||||
def refine_context(self, context):
|
||||
context = context.strip().split("\n")
|
||||
for c in range(len(context)):
|
||||
context[c] = context[c].strip().strip("\u3000").strip("\r")
|
||||
context = list(filter(lambda c: c != "", context))
|
||||
context = "\n" + ("\n".join(context)).strip()
|
||||
if context == "":
|
||||
context = "\n"
|
||||
return context
|
||||
|
||||
def sample_logits(
|
||||
self, out, x, ctx_len, temperature=1.0, top_p_usual=None, top_p_newline=None
|
||||
):
|
||||
# out[self.UNKNOWN_CHAR] = -float('Inf')
|
||||
lastChar = int(x[-1])
|
||||
|
||||
probs = F.softmax(out, dim=-1)
|
||||
|
||||
if self.charMode:
|
||||
if self.itos[lastChar] == "\n":
|
||||
top_p = top_p_newline
|
||||
else:
|
||||
top_p = top_p_usual
|
||||
else:
|
||||
top_p = top_p_usual
|
||||
|
||||
if os.environ["RWKV_RUN_DEVICE"] == "cpu":
|
||||
probs = probs.numpy()
|
||||
sorted_probs = np.sort(probs)[::-1]
|
||||
cumulative_probs = np.cumsum(sorted_probs)
|
||||
cutoff = float(sorted_probs[np.argmax(cumulative_probs > top_p)])
|
||||
probs[probs < cutoff] = 0
|
||||
if temperature != 1.0:
|
||||
probs = probs.pow(1.0 / temperature)
|
||||
probs = probs / np.sum(probs)
|
||||
out = np.random.choice(a=len(probs), p=probs)
|
||||
return out
|
||||
else:
|
||||
sorted_probs = torch.sort(probs, descending=True)[0]
|
||||
cumulative_probs = torch.cumsum(sorted_probs, dim=-1).cpu().numpy()
|
||||
cutoff = float(sorted_probs[np.argmax(cumulative_probs > top_p)])
|
||||
probs[probs < cutoff] = 0
|
||||
if temperature != 1.0:
|
||||
probs = probs.pow(1.0 / temperature)
|
||||
out = torch.multinomial(probs, num_samples=1)[0]
|
||||
return out
|
||||
|
||||
|
||||
def MaybeIsPrime(number):
|
||||
if FermatPrimalityTest(number) and MillerRabinPrimalityTest(number):
|
||||
return True
|
||||
else:
|
||||
return False
|
||||
|
||||
|
||||
def FermatPrimalityTest(number):
|
||||
if number > 1:
|
||||
for time in range(3):
|
||||
randomNumber = random.randint(2, number) - 1
|
||||
if pow(randomNumber, number - 1, number) != 1:
|
||||
return False
|
||||
return True
|
||||
else:
|
||||
return False
|
||||
|
||||
|
||||
def MillerRabinPrimalityTest(number):
|
||||
if number == 2:
|
||||
return True
|
||||
elif number == 1 or number % 2 == 0:
|
||||
return False
|
||||
oddPartOfNumber = number - 1
|
||||
timesTwoDividNumber = 0
|
||||
while oddPartOfNumber % 2 == 0:
|
||||
oddPartOfNumber = oddPartOfNumber // 2
|
||||
timesTwoDividNumber = timesTwoDividNumber + 1
|
||||
|
||||
for time in range(3):
|
||||
while True:
|
||||
randomNumber = random.randint(2, number) - 1
|
||||
if randomNumber != 0 and randomNumber != 1:
|
||||
break
|
||||
|
||||
randomNumberWithPower = pow(randomNumber, oddPartOfNumber, number)
|
||||
|
||||
if (randomNumberWithPower != 1) and (randomNumberWithPower != number - 1):
|
||||
iterationNumber = 1
|
||||
|
||||
while (iterationNumber <= timesTwoDividNumber - 1) and (
|
||||
randomNumberWithPower != number - 1
|
||||
):
|
||||
randomNumberWithPower = pow(randomNumberWithPower, 2, number)
|
||||
iterationNumber = iterationNumber + 1
|
||||
if randomNumberWithPower != (number - 1):
|
||||
return False
|
||||
|
||||
return True
|
436
finetune/lora/v5/train.py
vendored
Normal file
436
finetune/lora/v5/train.py
vendored
Normal file
@ -0,0 +1,436 @@
|
||||
########################################################################################################
|
||||
# The RWKV Language Model - https://github.com/BlinkDL/RWKV-LM
|
||||
########################################################################################################
|
||||
|
||||
import logging
|
||||
|
||||
logging.basicConfig(level=logging.INFO)
|
||||
|
||||
if __name__ == "__main__":
|
||||
from argparse import ArgumentParser
|
||||
from pytorch_lightning import Trainer
|
||||
from pytorch_lightning.utilities import rank_zero_info, rank_zero_only
|
||||
import pytorch_lightning as pl
|
||||
|
||||
rank_zero_info("########## work in progress ##########")
|
||||
|
||||
parser = ArgumentParser()
|
||||
|
||||
parser.add_argument("--load_model", default="", type=str) # full path, with .pth
|
||||
parser.add_argument(
|
||||
"--wandb", default="", type=str
|
||||
) # wandb project name. if "" then don't use wandb
|
||||
parser.add_argument("--proj_dir", default="out", type=str)
|
||||
parser.add_argument("--random_seed", default="-1", type=int)
|
||||
|
||||
parser.add_argument("--data_file", default="", type=str)
|
||||
parser.add_argument("--data_type", default="utf-8", type=str)
|
||||
parser.add_argument(
|
||||
"--vocab_size", default=0, type=int
|
||||
) # vocab_size = 0 means auto (for char-level LM and .txt data)
|
||||
|
||||
parser.add_argument("--ctx_len", default=1024, type=int)
|
||||
parser.add_argument(
|
||||
"--epoch_steps", default=1000, type=int
|
||||
) # a mini "epoch" has [epoch_steps] steps
|
||||
parser.add_argument(
|
||||
"--epoch_count", default=500, type=int
|
||||
) # train for this many "epochs". will continue afterwards with lr = lr_final
|
||||
parser.add_argument(
|
||||
"--epoch_begin", default=0, type=int
|
||||
) # if you load a model trained for x "epochs", set epoch_begin = x
|
||||
parser.add_argument(
|
||||
"--epoch_save", default=5, type=int
|
||||
) # save the model every [epoch_save] "epochs"
|
||||
|
||||
parser.add_argument(
|
||||
"--micro_bsz", default=12, type=int
|
||||
) # micro batch size (batch size per GPU)
|
||||
parser.add_argument("--n_layer", default=6, type=int)
|
||||
parser.add_argument("--n_embd", default=512, type=int)
|
||||
parser.add_argument("--dim_att", default=0, type=int)
|
||||
parser.add_argument("--dim_ffn", default=0, type=int)
|
||||
parser.add_argument(
|
||||
"--pre_ffn", default=0, type=int
|
||||
) # replace first att layer by ffn (sometimes better)
|
||||
parser.add_argument("--head_qk", default=0, type=int) # my headQK trick
|
||||
parser.add_argument("--tiny_att_dim", default=0, type=int) # tiny attention dim
|
||||
parser.add_argument(
|
||||
"--tiny_att_layer", default=-999, type=int
|
||||
) # tiny attention @ which layer
|
||||
|
||||
parser.add_argument(
|
||||
"--lr_init", default=6e-4, type=float
|
||||
) # 6e-4 for L12-D768, 4e-4 for L24-D1024, 3e-4 for L24-D2048
|
||||
parser.add_argument("--lr_final", default=1e-5, type=float)
|
||||
parser.add_argument(
|
||||
"--warmup_steps", default=-1, type=int
|
||||
) # try 50 if you load a model
|
||||
parser.add_argument("--beta1", default=0.9, type=float)
|
||||
parser.add_argument(
|
||||
"--beta2", default=0.99, type=float
|
||||
) # use 0.999 when your model is close to convergence
|
||||
parser.add_argument("--adam_eps", default=1e-8, type=float)
|
||||
parser.add_argument(
|
||||
"--grad_cp", default=0, type=int
|
||||
) # gradient checkpt: saves VRAM, but slower
|
||||
parser.add_argument(
|
||||
"--dropout", default=0, type=float
|
||||
) # try 0.01 / 0.02 / 0.05 / 0.1
|
||||
parser.add_argument(
|
||||
"--weight_decay", default=0, type=float
|
||||
) # try 0.1 / 0.01 / 0.001
|
||||
parser.add_argument("--weight_decay_final", default=-1, type=float)
|
||||
|
||||
parser.add_argument(
|
||||
"--my_pile_version", default=1, type=int
|
||||
) # my special pile version
|
||||
parser.add_argument("--my_pile_stage", default=0, type=int) # my special pile mode
|
||||
parser.add_argument(
|
||||
"--my_pile_shift", default=-1, type=int
|
||||
) # my special pile mode - text shift
|
||||
parser.add_argument("--my_pile_edecay", default=0, type=int)
|
||||
parser.add_argument(
|
||||
"--layerwise_lr", default=1, type=int
|
||||
) # layerwise lr for faster convergence (but slower it/s)
|
||||
parser.add_argument(
|
||||
"--ds_bucket_mb", default=200, type=int
|
||||
) # deepspeed bucket size in MB. 200 seems enough
|
||||
# parser.add_argument("--cuda_cleanup", default=0, type=int) # extra cuda cleanup (sometimes helpful)
|
||||
|
||||
parser.add_argument("--my_sample_len", default=0, type=int)
|
||||
parser.add_argument("--my_ffn_shift", default=1, type=int)
|
||||
parser.add_argument("--my_att_shift", default=1, type=int)
|
||||
parser.add_argument(
|
||||
"--head_size_a", default=64, type=int
|
||||
) # can try larger values for larger models
|
||||
parser.add_argument("--head_size_divisor", default=8, type=int)
|
||||
parser.add_argument("--my_pos_emb", default=0, type=int)
|
||||
parser.add_argument("--load_partial", default=0, type=int)
|
||||
parser.add_argument("--magic_prime", default=0, type=int)
|
||||
parser.add_argument("--my_qa_mask", default=0, type=int)
|
||||
parser.add_argument("--my_random_steps", default=0, type=int)
|
||||
parser.add_argument("--my_testing", default="", type=str)
|
||||
parser.add_argument("--my_exit", default=99999999, type=int)
|
||||
parser.add_argument("--my_exit_tokens", default=0, type=int)
|
||||
|
||||
# LORA
|
||||
parser.add_argument("--emb", action="store_true")
|
||||
parser.add_argument("--lora", action="store_true")
|
||||
parser.add_argument("--lora_load", default="", type=str)
|
||||
parser.add_argument("--lora_r", default=8, type=int)
|
||||
parser.add_argument("--lora_alpha", default=32, type=float)
|
||||
parser.add_argument("--lora_dropout", default=0.01, type=float)
|
||||
parser.add_argument("--lora_parts", default="att,ln,time", type=str)
|
||||
|
||||
if pl.__version__[0] == "2":
|
||||
parser.add_argument("--accelerator", default="gpu", type=str)
|
||||
parser.add_argument("--strategy", default="auto", type=str)
|
||||
parser.add_argument("--devices", default=1, type=int)
|
||||
parser.add_argument("--num_nodes", default=1, type=int)
|
||||
parser.add_argument("--precision", default="fp16", type=str)
|
||||
parser.add_argument("--accumulate_grad_batches", default=1, type=int)
|
||||
else:
|
||||
parser = Trainer.add_argparse_args(parser)
|
||||
args = parser.parse_args()
|
||||
|
||||
########################################################################################################
|
||||
|
||||
import os, warnings, math, datetime, sys, time
|
||||
import numpy as np
|
||||
import torch
|
||||
from torch.utils.data import DataLoader
|
||||
|
||||
if "deepspeed" in args.strategy:
|
||||
import deepspeed
|
||||
from pytorch_lightning import seed_everything
|
||||
|
||||
if args.random_seed >= 0:
|
||||
print(
|
||||
f"########## WARNING: GLOBAL SEED {args.random_seed} THIS WILL AFFECT MULTIGPU SAMPLING ##########\n"
|
||||
* 3
|
||||
)
|
||||
seed_everything(args.random_seed)
|
||||
|
||||
np.set_printoptions(precision=4, suppress=True, linewidth=200)
|
||||
warnings.filterwarnings(
|
||||
"ignore", ".*Consider increasing the value of the `num_workers` argument*"
|
||||
)
|
||||
warnings.filterwarnings(
|
||||
"ignore", ".*The progress bar already tracks a metric with the*"
|
||||
)
|
||||
# os.environ["WDS_SHOW_SEED"] = "1"
|
||||
|
||||
args.my_timestamp = datetime.datetime.today().strftime("%Y-%m-%d-%H-%M-%S")
|
||||
args.enable_checkpointing = False
|
||||
args.replace_sampler_ddp = False
|
||||
args.logger = False
|
||||
args.gradient_clip_val = 1.0
|
||||
args.num_sanity_val_steps = 0
|
||||
args.check_val_every_n_epoch = int(1e20)
|
||||
args.log_every_n_steps = int(1e20)
|
||||
args.max_epochs = args.epoch_count # -1 continue forever
|
||||
args.betas = (args.beta1, args.beta2)
|
||||
args.real_bsz = int(args.num_nodes) * int(args.devices) * args.micro_bsz
|
||||
os.environ["RWKV_MY_TESTING"] = args.my_testing
|
||||
os.environ["RWKV_HEAD_SIZE_A"] = str(args.head_size_a)
|
||||
if args.dim_att <= 0:
|
||||
args.dim_att = args.n_embd
|
||||
if args.dim_ffn <= 0:
|
||||
args.dim_ffn = int((args.n_embd * 3.5) // 32 * 32) # default = 3.5x emb size
|
||||
|
||||
if args.data_type == "wds_img":
|
||||
args.run_name = f"v{args.my_img_version}-{args.my_img_size}-{args.my_img_bit}bit-{args.my_img_clip}x{args.my_img_clip_scale}"
|
||||
args.proj_dir = f"{args.proj_dir}-{args.run_name}"
|
||||
else:
|
||||
args.run_name = (
|
||||
f"{args.vocab_size} ctx{args.ctx_len} L{args.n_layer} D{args.n_embd}"
|
||||
)
|
||||
if not os.path.exists(args.proj_dir):
|
||||
os.makedirs(args.proj_dir)
|
||||
|
||||
if args.my_pile_stage > 0:
|
||||
magic_prime_bak = args.magic_prime
|
||||
|
||||
if args.my_pile_shift < 0:
|
||||
args.my_pile_shift = 0
|
||||
|
||||
if magic_prime_bak > 0:
|
||||
args.magic_prime = magic_prime_bak
|
||||
if args.my_qa_mask == 2:
|
||||
args.epoch_count = 2 * args.magic_prime // 40320
|
||||
else:
|
||||
args.epoch_count = args.magic_prime // 40320
|
||||
|
||||
args.epoch_steps = 40320 // args.real_bsz
|
||||
assert args.epoch_steps * args.real_bsz == 40320
|
||||
# if args.my_pile_stage == 2:
|
||||
# assert args.lr_final == args.lr_init
|
||||
if args.my_pile_stage >= 2: # find latest saved model
|
||||
list_p = []
|
||||
for p in os.listdir(args.proj_dir):
|
||||
if p.startswith("rwkv") and p.endswith(".pth"):
|
||||
p = ((p.split("-"))[1].split("."))[0]
|
||||
if p != "final":
|
||||
if p == "init":
|
||||
p = -1
|
||||
else:
|
||||
p = int(p)
|
||||
list_p += [p]
|
||||
list_p.sort()
|
||||
max_p = list_p[-1]
|
||||
if len(list_p) > 1:
|
||||
args.my_pile_prev_p = list_p[-2] # in case max_p is corrupted
|
||||
if max_p == -1:
|
||||
args.load_model = f"{args.proj_dir}/rwkv-init.pth"
|
||||
else:
|
||||
args.load_model = f"{args.proj_dir}/rwkv-{max_p}.pth"
|
||||
if args.warmup_steps < 0:
|
||||
if args.my_pile_stage == 2:
|
||||
args.warmup_steps = 10
|
||||
else:
|
||||
args.warmup_steps = 30
|
||||
args.epoch_begin = max_p + 1
|
||||
|
||||
samples_per_epoch = args.epoch_steps * args.real_bsz
|
||||
tokens_per_epoch = samples_per_epoch * args.ctx_len
|
||||
try:
|
||||
deepspeed_version = deepspeed.__version__
|
||||
except:
|
||||
deepspeed_version = None
|
||||
pass
|
||||
rank_zero_info(
|
||||
f"""
|
||||
############################################################################
|
||||
#
|
||||
# RWKV-5 {args.precision.upper()} on {args.num_nodes}x{args.devices} {args.accelerator.upper()}, bsz {args.num_nodes}x{args.devices}x{args.micro_bsz}={args.real_bsz}, {args.strategy} {'with grad_cp' if args.grad_cp > 0 else ''}
|
||||
#
|
||||
# Data = {args.data_file} ({args.data_type}), ProjDir = {args.proj_dir}
|
||||
#
|
||||
# Epoch = {args.epoch_begin} to {args.epoch_begin + args.epoch_count - 1}, save every {args.epoch_save} epoch
|
||||
#
|
||||
# Each "epoch" = {args.epoch_steps} steps, {samples_per_epoch} samples, {tokens_per_epoch} tokens
|
||||
#
|
||||
# Model = {args.n_layer} n_layer, {args.n_embd} n_embd, {args.ctx_len} ctx_len
|
||||
#
|
||||
# Adam = lr {args.lr_init} to {args.lr_final}, warmup {args.warmup_steps} steps, beta {args.betas}, eps {args.adam_eps}
|
||||
#
|
||||
# Found torch {torch.__version__}, recommend 1.13.1+cu117 or newer
|
||||
# Found deepspeed {deepspeed_version}, recommend 0.7.0 (faster than newer versions)
|
||||
# Found pytorch_lightning {pl.__version__}, recommend 1.9.5
|
||||
#
|
||||
############################################################################
|
||||
"""
|
||||
)
|
||||
rank_zero_info(str(vars(args)) + "\n")
|
||||
|
||||
assert args.data_type in ["utf-8", "utf-16le", "numpy", "binidx", "dummy", "uint16"]
|
||||
|
||||
if args.lr_final == 0 or args.lr_init == 0:
|
||||
rank_zero_info(
|
||||
"\n\nNote: lr_final = 0 or lr_init = 0. Using linear LR schedule instead.\n\n"
|
||||
)
|
||||
|
||||
assert args.precision in ["fp32", "tf32", "fp16", "bf16"]
|
||||
os.environ["RWKV_FLOAT_MODE"] = args.precision
|
||||
if args.precision == "fp32":
|
||||
for i in range(10):
|
||||
rank_zero_info(
|
||||
"\n\nNote: you are using fp32 (very slow). Try bf16 / tf32 for faster training.\n\n"
|
||||
)
|
||||
if args.precision == "fp16":
|
||||
rank_zero_info(
|
||||
"\n\nNote: you are using fp16 (might overflow). Try bf16 / tf32 for stable training.\n\n"
|
||||
)
|
||||
|
||||
os.environ["RWKV_JIT_ON"] = "0"
|
||||
if "deepspeed_stage_3" in args.strategy:
|
||||
os.environ["RWKV_JIT_ON"] = "0"
|
||||
|
||||
torch.backends.cudnn.benchmark = True
|
||||
torch.backends.cudnn.enabled = True
|
||||
if args.precision == "fp32":
|
||||
torch.backends.cudnn.allow_tf32 = False
|
||||
torch.backends.cuda.matmul.allow_tf32 = False
|
||||
else:
|
||||
torch.backends.cudnn.allow_tf32 = True
|
||||
torch.backends.cuda.matmul.allow_tf32 = True
|
||||
|
||||
if "32" in args.precision:
|
||||
args.precision = 32
|
||||
elif args.precision == "fp16":
|
||||
args.precision = 16
|
||||
else:
|
||||
args.precision = "bf16"
|
||||
|
||||
########################################################################################################
|
||||
|
||||
from src.trainer import train_callback, generate_init_weight
|
||||
from src.dataset import MyDataset
|
||||
|
||||
train_data = MyDataset(args)
|
||||
args.vocab_size = train_data.vocab_size
|
||||
|
||||
from src.model import RWKV, LORA_CONFIG, LoraLinear
|
||||
|
||||
if args.lora:
|
||||
assert args.lora_r > 0, "LoRA should have its `r` > 0"
|
||||
LORA_CONFIG["r"] = args.lora_r
|
||||
LORA_CONFIG["alpha"] = args.lora_alpha
|
||||
LORA_CONFIG["dropout"] = args.lora_dropout
|
||||
LORA_CONFIG["parts"] = set(str(args.lora_parts).split(","))
|
||||
enable_time_finetune = "time" in LORA_CONFIG["parts"]
|
||||
enable_ln_finetune = "ln" in LORA_CONFIG["parts"]
|
||||
model = RWKV(args)
|
||||
# only train lora parameters
|
||||
if args.lora:
|
||||
model.requires_grad_(False)
|
||||
for name, module in model.named_modules():
|
||||
if any(n.startswith("lora_") for n, _ in module.named_parameters()):
|
||||
print(f" LoRA additionally training module {name}")
|
||||
for pname, param in module.named_parameters():
|
||||
param.requires_grad = "lora_" in pname
|
||||
elif enable_ln_finetune and ".ln" in name:
|
||||
print(f" LoRA additionally training module {name}")
|
||||
for param in module.parameters():
|
||||
param.requires_grad = True
|
||||
elif enable_time_finetune and any(
|
||||
n.startswith("time") for n, _ in module.named_parameters()
|
||||
):
|
||||
for pname, param in module.named_parameters():
|
||||
if pname.startswith("time"):
|
||||
print(f" LoRA additionally training parameter {pname}")
|
||||
param.requires_grad = True
|
||||
|
||||
if (
|
||||
len(args.load_model) == 0 or args.my_pile_stage == 1
|
||||
): # shall we build the initial weights?
|
||||
init_weight_name = f"{args.proj_dir}/rwkv-init.pth"
|
||||
generate_init_weight(model, init_weight_name) # save initial weights
|
||||
args.load_model = init_weight_name
|
||||
|
||||
rank_zero_info(f"########## Loading {args.load_model}... ##########")
|
||||
try:
|
||||
load_dict = torch.load(args.load_model, map_location="cpu")
|
||||
load_keys = list(load_dict.keys())
|
||||
for k in load_keys:
|
||||
if k.startswith("_forward_module."):
|
||||
load_dict[k.replace("_forward_module.", "")] = load_dict[k]
|
||||
del load_dict[k]
|
||||
except:
|
||||
rank_zero_info(f"Bad checkpoint {args.load_model}")
|
||||
if args.my_pile_stage >= 2: # try again using another checkpoint
|
||||
max_p = args.my_pile_prev_p
|
||||
if max_p == -1:
|
||||
args.load_model = f"{args.proj_dir}/rwkv-init.pth"
|
||||
else:
|
||||
args.load_model = f"{args.proj_dir}/rwkv-{max_p}.pth"
|
||||
args.epoch_begin = max_p + 1
|
||||
rank_zero_info(f"Trying {args.load_model}")
|
||||
load_dict = torch.load(args.load_model, map_location="cpu")
|
||||
|
||||
if args.load_partial == 1:
|
||||
load_keys = load_dict.keys()
|
||||
for k in model.state_dict():
|
||||
if k not in load_keys:
|
||||
load_dict[k] = model.state_dict()[k]
|
||||
# model.load_state_dict(load_dict)
|
||||
|
||||
model.load_state_dict(load_dict, strict=(not args.lora))
|
||||
if os.path.isfile(args.lora_load):
|
||||
model.load_state_dict(
|
||||
torch.load(args.lora_load, map_location="cpu"), strict=False
|
||||
)
|
||||
|
||||
if pl.__version__[0] == "2":
|
||||
trainer = Trainer(
|
||||
accelerator=args.accelerator,
|
||||
strategy=args.strategy,
|
||||
devices=args.devices,
|
||||
num_nodes=args.num_nodes,
|
||||
precision=args.precision,
|
||||
logger=args.logger,
|
||||
callbacks=[train_callback(args)],
|
||||
max_epochs=args.max_epochs,
|
||||
check_val_every_n_epoch=args.check_val_every_n_epoch,
|
||||
num_sanity_val_steps=args.num_sanity_val_steps,
|
||||
log_every_n_steps=args.log_every_n_steps,
|
||||
enable_checkpointing=args.enable_checkpointing,
|
||||
accumulate_grad_batches=args.accumulate_grad_batches,
|
||||
gradient_clip_val=args.gradient_clip_val,
|
||||
)
|
||||
else:
|
||||
trainer = Trainer.from_argparse_args(
|
||||
args,
|
||||
callbacks=[train_callback(args)],
|
||||
)
|
||||
|
||||
if trainer.global_rank == 0:
|
||||
for n in model.state_dict():
|
||||
shape = model.state_dict()[n].shape
|
||||
shape = [i for i in shape if i != 1]
|
||||
if len(shape) > 1:
|
||||
print(f"{str(shape[0]).ljust(5)} {str(shape[1]).ljust(5)} {n}")
|
||||
else:
|
||||
print(f"{str(shape[0]).ljust(5)} {n}")
|
||||
|
||||
if "deepspeed" in args.strategy:
|
||||
trainer.strategy.config["zero_optimization"]["allgather_bucket_size"] = (
|
||||
args.ds_bucket_mb * 1000 * 1000
|
||||
)
|
||||
trainer.strategy.config["zero_optimization"]["reduce_bucket_size"] = (
|
||||
args.ds_bucket_mb * 1000 * 1000
|
||||
)
|
||||
|
||||
# must set shuffle=False, persistent_workers=False (because worker is in another thread)
|
||||
data_loader = DataLoader(
|
||||
train_data,
|
||||
shuffle=False,
|
||||
pin_memory=True,
|
||||
batch_size=args.micro_bsz,
|
||||
num_workers=1,
|
||||
persistent_workers=False,
|
||||
drop_last=True,
|
||||
)
|
||||
|
||||
trainer.fit(model, data_loader)
|
202
finetune/lora/v6/cuda/wkv5_cuda.cu
vendored
Normal file
202
finetune/lora/v6/cuda/wkv5_cuda.cu
vendored
Normal file
@ -0,0 +1,202 @@
|
||||
#include <stdio.h>
|
||||
#include <assert.h>
|
||||
#include "ATen/ATen.h"
|
||||
typedef at::BFloat16 bf16;
|
||||
|
||||
template <typename F>
|
||||
__global__ void kernel_forward(const int B, const int T, const int C, const int H,
|
||||
const F *__restrict__ const _r, const F *__restrict__ const _k, const F *__restrict__ const _v, const float *__restrict__ _w, const F *__restrict__ _u,
|
||||
F *__restrict__ const _y)
|
||||
{
|
||||
const int b = blockIdx.x / H;
|
||||
const int h = blockIdx.x % H;
|
||||
const int i = threadIdx.x;
|
||||
_w += h*_N_;
|
||||
_u += h*_N_;
|
||||
|
||||
__shared__ float r[_N_], k[_N_], u[_N_], w[_N_];
|
||||
float state[_N_] = {0};
|
||||
|
||||
__syncthreads();
|
||||
w[i] = _w[i];
|
||||
u[i] = float(_u[i]);
|
||||
__syncthreads();
|
||||
|
||||
for (int t = b*T*C + h*_N_ + i; t < (b+1)*T*C + h*_N_ + i; t += C)
|
||||
{
|
||||
__syncthreads();
|
||||
r[i] = float(_r[t]);
|
||||
k[i] = float(_k[t]);
|
||||
__syncthreads();
|
||||
|
||||
const float v = float(_v[t]);
|
||||
float y = 0;
|
||||
|
||||
#pragma unroll
|
||||
for (int j = 0; j < _N_; j+=4)
|
||||
{
|
||||
const float4& r_ = (float4&)(r[j]);
|
||||
const float4& k_ = (float4&)(k[j]);
|
||||
const float4& w_ = (float4&)(w[j]);
|
||||
const float4& u_ = (float4&)(u[j]);
|
||||
float4& s = (float4&)(state[j]);
|
||||
float4 x;
|
||||
|
||||
x.x = k_.x * v;
|
||||
x.y = k_.y * v;
|
||||
x.z = k_.z * v;
|
||||
x.w = k_.w * v;
|
||||
|
||||
y += r_.x * (u_.x * x.x + s.x);
|
||||
y += r_.y * (u_.y * x.y + s.y);
|
||||
y += r_.z * (u_.z * x.z + s.z);
|
||||
y += r_.w * (u_.w * x.w + s.w);
|
||||
|
||||
s.x = s.x * w_.x + x.x;
|
||||
s.y = s.y * w_.y + x.y;
|
||||
s.z = s.z * w_.z + x.z;
|
||||
s.w = s.w * w_.w + x.w;
|
||||
}
|
||||
_y[t] = F(y);
|
||||
}
|
||||
}
|
||||
|
||||
template <typename F>
|
||||
__global__ void kernel_backward(const int B, const int T, const int C, const int H,
|
||||
const F *__restrict__ const _r, const F *__restrict__ const _k, const F *__restrict__ const _v, const float *__restrict__ _w, const float *__restrict__ __w, const F *__restrict__ _u, const F *__restrict__ const _gy,
|
||||
F *__restrict__ const _gr, F *__restrict__ const _gk, F *__restrict__ const _gv, F *__restrict__ const _gw, F *__restrict__ const _gu)
|
||||
{
|
||||
const int b = blockIdx.x / H;
|
||||
const int h = blockIdx.x % H;
|
||||
const int i = threadIdx.x;
|
||||
_w += h*_N_;
|
||||
_u += h*_N_;
|
||||
__w += h*_N_;
|
||||
|
||||
__shared__ float w_[_N_], u_[_N_];
|
||||
__shared__ float r[_N_], k[_N_], v[_N_], gy[_N_];
|
||||
__syncthreads();
|
||||
w_[i] = _w[i];
|
||||
u_[i] = float(_u[i]);
|
||||
__syncthreads();
|
||||
|
||||
const float w = w_[i];
|
||||
const float ww = __w[i];
|
||||
const float u = u_[i];
|
||||
|
||||
float state[_N_] = {0}, saaaa[_N_] = {0}, sbbbb[_N_] = {0}, scccc[_N_] = {0}, sdddd[_N_] = {0};
|
||||
|
||||
float gw = 0, gu = 0;
|
||||
const int t000 = b*T*C + h*_N_ + i;
|
||||
const int t111 = (b+1)*T*C + h*_N_ + i;
|
||||
const int t222 = t111 - 2*C;
|
||||
|
||||
for (int t = t000; t < t111; t += C)
|
||||
{
|
||||
__syncthreads();
|
||||
v[i] = float(_v[t]);
|
||||
gy[i] = float(_gy[t]);
|
||||
__syncthreads();
|
||||
|
||||
const float k = float(_k[t]);
|
||||
float gr = 0, gu_ = 0;
|
||||
|
||||
#pragma unroll
|
||||
for (int j = 0; j < _N_; j++)
|
||||
{
|
||||
float& s = state[j];
|
||||
float x = k * v[j];
|
||||
|
||||
gr += (u * x + s) * gy[j];
|
||||
gu_ += x * gy[j];
|
||||
s = s * w + x;
|
||||
}
|
||||
_gr[t] = F(gr);
|
||||
gu += float(_r[t]) * gu_;
|
||||
}
|
||||
_gu[b*C + h*_N_ + i] = F(gu);
|
||||
|
||||
for (int t = t000; t < t222; t += C)
|
||||
{
|
||||
__syncthreads();
|
||||
v[i] = float(_v[t]);
|
||||
gy[i] = float(_gy[t + 2*C]);
|
||||
__syncthreads();
|
||||
|
||||
const float k = float(_k[t]);
|
||||
float gw_ = 0;
|
||||
|
||||
#pragma unroll
|
||||
for (int j = 0; j < _N_; j++)
|
||||
{
|
||||
float& s = saaaa[j];
|
||||
float& s2 = sbbbb[j];
|
||||
float x = k * v[j];
|
||||
|
||||
float tmp = w * (x + s);
|
||||
s = tmp;
|
||||
s2 = tmp + w * s2;
|
||||
gw_ += s2 * gy[j];
|
||||
}
|
||||
gw += float(_r[t + 2*C]) * gw_;
|
||||
}
|
||||
_gw[b*C + h*_N_ + i] = F(ww * gw);
|
||||
|
||||
for (int t = t111 - C; t >= t000; t -= C)
|
||||
{
|
||||
__syncthreads();
|
||||
v[i] = float(_v[t]);
|
||||
gy[i] = float(_gy[t]);
|
||||
__syncthreads();
|
||||
|
||||
const float rr = float(_r[t]);
|
||||
float gk = 0;
|
||||
|
||||
#pragma unroll
|
||||
for (int j = 0; j < _N_; j++)
|
||||
{
|
||||
float& s = scccc[j];
|
||||
float x = rr * gy[j];
|
||||
|
||||
gk += (u * x + s) * v[j];
|
||||
s = x + s * w;
|
||||
}
|
||||
_gk[t] = F(gk);
|
||||
}
|
||||
|
||||
for (int t = t111 - C; t >= t000; t -= C)
|
||||
{
|
||||
__syncthreads();
|
||||
r[i] = float(_r[t]);
|
||||
k[i] = float(_k[t]);
|
||||
__syncthreads();
|
||||
|
||||
const float gyy = float(_gy[t]);
|
||||
float gv = 0;
|
||||
|
||||
#pragma unroll
|
||||
for (int j = 0; j < _N_; j++)
|
||||
{
|
||||
float& s = sdddd[j];
|
||||
float x = gyy * r[j];
|
||||
|
||||
gv += (u_[j] * x + s) * k[j];
|
||||
s = x + s * w_[j];
|
||||
}
|
||||
_gv[t] = F(gv);
|
||||
}
|
||||
}
|
||||
|
||||
void cuda_forward(int B, int T, int C, int H, bf16 *r, bf16 *k, bf16 *v, float *w, bf16 *u, bf16 *y)
|
||||
{
|
||||
assert(H*_N_ == C);
|
||||
assert(_N_%4 == 0);
|
||||
kernel_forward<<<dim3(B * H), dim3(_N_)>>>(B, T, C, H, r, k, v, w, u, y);
|
||||
}
|
||||
|
||||
void cuda_backward(int B, int T, int C, int H, bf16 *r, bf16 *k, bf16 *v, float *w, float *ww, bf16 *u, bf16 *gy, bf16 *gr, bf16 *gk, bf16 *gv, bf16 *gw, bf16 *gu)
|
||||
{
|
||||
assert(H*_N_ == C);
|
||||
assert(_N_%4 == 0);
|
||||
kernel_backward<<<dim3(B * H), dim3(_N_)>>>(B, T, C, H, r, k, v, w, ww, u, gy, gr, gk, gv, gw, gu);
|
||||
}
|
22
finetune/lora/v6/cuda/wkv5_op.cpp
vendored
Normal file
22
finetune/lora/v6/cuda/wkv5_op.cpp
vendored
Normal file
@ -0,0 +1,22 @@
|
||||
#include <torch/extension.h>
|
||||
#include "ATen/ATen.h"
|
||||
typedef at::BFloat16 bf16;
|
||||
|
||||
void cuda_forward(int B, int T, int C, int H, bf16 *r, bf16 *k, bf16 *v, float *w, bf16 *u, bf16 *y);
|
||||
void cuda_backward(int B, int T, int C, int H, bf16 *r, bf16 *k, bf16 *v, float *w, float *ww, bf16 *u, bf16 *gy, bf16 *gr, bf16 *gk, bf16 *gv, bf16 *gw, bf16 *gu);
|
||||
|
||||
void forward(int64_t B, int64_t T, int64_t C, int64_t H, torch::Tensor &r, torch::Tensor &k, torch::Tensor &v, torch::Tensor &w, torch::Tensor &u, torch::Tensor &y) {
|
||||
cuda_forward(B, T, C, H, r.data_ptr<bf16>(), k.data_ptr<bf16>(), v.data_ptr<bf16>(), w.data_ptr<float>(), u.data_ptr<bf16>(), y.data_ptr<bf16>());
|
||||
}
|
||||
void backward(int64_t B, int64_t T, int64_t C, int64_t H, torch::Tensor &r, torch::Tensor &k, torch::Tensor &v, torch::Tensor &w, torch::Tensor &ww, torch::Tensor &u, torch::Tensor &gy, torch::Tensor &gr, torch::Tensor &gk, torch::Tensor &gv, torch::Tensor &gw, torch::Tensor &gu) {
|
||||
cuda_backward(B, T, C, H, r.data_ptr<bf16>(), k.data_ptr<bf16>(), v.data_ptr<bf16>(), w.data_ptr<float>(), ww.data_ptr<float>(), u.data_ptr<bf16>(), gy.data_ptr<bf16>(), gr.data_ptr<bf16>(), gk.data_ptr<bf16>(), gv.data_ptr<bf16>(), gw.data_ptr<bf16>(), gu.data_ptr<bf16>());
|
||||
}
|
||||
PYBIND11_MODULE(TORCH_EXTENSION_NAME, m) {
|
||||
m.def("forward", &forward, "wkv5 forward");
|
||||
m.def("backward", &backward, "wkv5 backward");
|
||||
}
|
||||
|
||||
TORCH_LIBRARY(wkv5, m) {
|
||||
m.def("forward", forward);
|
||||
m.def("backward", backward);
|
||||
}
|
242
finetune/lora/v6/cuda/wkv6_cuda.cu
vendored
Normal file
242
finetune/lora/v6/cuda/wkv6_cuda.cu
vendored
Normal file
@ -0,0 +1,242 @@
|
||||
#include <stdio.h>
|
||||
#include <assert.h>
|
||||
#include "ATen/ATen.h"
|
||||
typedef at::BFloat16 bf16;
|
||||
|
||||
template <typename F>
|
||||
__global__ void kernel_forward(const int B, const int T, const int C, const int H,
|
||||
const F *__restrict__ const _r, const F *__restrict__ const _k, const F *__restrict__ const _v, const float *__restrict__ _w, const F *__restrict__ _u,
|
||||
F *__restrict__ const _y)
|
||||
{
|
||||
const int b = blockIdx.x / H;
|
||||
const int h = blockIdx.x % H;
|
||||
const int i = threadIdx.x;
|
||||
_u += h*_N_;
|
||||
|
||||
__shared__ float r[_N_], k[_N_], u[_N_], w[_N_];
|
||||
float state[_N_] = {0};
|
||||
|
||||
__syncthreads();
|
||||
u[i] = float(_u[i]);
|
||||
__syncthreads();
|
||||
|
||||
for (int t = b*T*C + h*_N_ + i; t < (b+1)*T*C + h*_N_ + i; t += C)
|
||||
{
|
||||
__syncthreads();
|
||||
w[i] = exp(_w[t]);
|
||||
r[i] = float(_r[t]);
|
||||
k[i] = float(_k[t]);
|
||||
__syncthreads();
|
||||
|
||||
const float v = float(_v[t]);
|
||||
float y = 0;
|
||||
|
||||
#pragma unroll
|
||||
for (int j = 0; j < _N_; j+=4)
|
||||
{
|
||||
const float4& r_ = (float4&)(r[j]);
|
||||
const float4& k_ = (float4&)(k[j]);
|
||||
const float4& w_ = (float4&)(w[j]);
|
||||
const float4& u_ = (float4&)(u[j]);
|
||||
float4& s = (float4&)(state[j]);
|
||||
float4 x;
|
||||
|
||||
x.x = k_.x * v;
|
||||
x.y = k_.y * v;
|
||||
x.z = k_.z * v;
|
||||
x.w = k_.w * v;
|
||||
|
||||
y += r_.x * (u_.x * x.x + s.x);
|
||||
y += r_.y * (u_.y * x.y + s.y);
|
||||
y += r_.z * (u_.z * x.z + s.z);
|
||||
y += r_.w * (u_.w * x.w + s.w);
|
||||
|
||||
s.x = s.x * w_.x + x.x;
|
||||
s.y = s.y * w_.y + x.y;
|
||||
s.z = s.z * w_.z + x.z;
|
||||
s.w = s.w * w_.w + x.w;
|
||||
}
|
||||
_y[t] = F(y);
|
||||
}
|
||||
}
|
||||
|
||||
template <typename F>
|
||||
__global__ void kernel_backward_111(const int B, const int T, const int C, const int H,
|
||||
const F *__restrict__ const _r, const F *__restrict__ const _k, const F *__restrict__ const _v, const float *__restrict__ _w, const F *__restrict__ _u, const F *__restrict__ const _gy,
|
||||
F *__restrict__ const _gr, F *__restrict__ const _gk, F *__restrict__ const _gv, F *__restrict__ const _gu)
|
||||
{
|
||||
const int b = blockIdx.x / H;
|
||||
const int h = blockIdx.x % H;
|
||||
const int i = threadIdx.x;
|
||||
_u += h*_N_;
|
||||
|
||||
__shared__ float u_[_N_];
|
||||
__shared__ float r[_N_], k[_N_], v[_N_], w_[_N_], gy[_N_];
|
||||
__syncthreads();
|
||||
u_[i] = float(_u[i]);
|
||||
__syncthreads();
|
||||
|
||||
const float u = u_[i];
|
||||
|
||||
float state[_N_] = {0}, scccc[_N_] = {0}, sdddd[_N_] = {0};
|
||||
|
||||
const int t_0 = b*T*C + h*_N_ + i;
|
||||
const int t_T_1 = t_0 + (T-1)*C;
|
||||
const int t_T = t_0 + T*C;
|
||||
|
||||
float gu = 0;
|
||||
for (int t = t_0; t < t_T; t += C)
|
||||
{
|
||||
__syncthreads();
|
||||
v[i] = float(_v[t]);
|
||||
gy[i] = float(_gy[t]);
|
||||
__syncthreads();
|
||||
|
||||
const float k = float(_k[t]);
|
||||
const float w = exp(_w[t]);
|
||||
float gr = 0, gu_ = 0;
|
||||
|
||||
#pragma unroll
|
||||
for (int j = 0; j < _N_; j++)
|
||||
{
|
||||
float& s = state[j];
|
||||
float x = k * v[j];
|
||||
|
||||
gr += (u * x + s) * gy[j];
|
||||
gu_ += x * gy[j];
|
||||
s = s * w + x;
|
||||
}
|
||||
_gr[t] = F(gr);
|
||||
gu += float(_r[t]) * gu_;
|
||||
}
|
||||
_gu[b*C + h*_N_ + i] = F(gu);
|
||||
|
||||
for (int t = t_T_1; t >= t_0; t -= C)
|
||||
{
|
||||
__syncthreads();
|
||||
v[i] = float(_v[t]);
|
||||
gy[i] = float(_gy[t]);
|
||||
__syncthreads();
|
||||
|
||||
const float rr = float(_r[t]);
|
||||
const float w = exp(_w[t]);
|
||||
float gk = 0;
|
||||
|
||||
#pragma unroll
|
||||
for (int j = 0; j < _N_; j++)
|
||||
{
|
||||
float& s = scccc[j];
|
||||
float x = rr * gy[j];
|
||||
|
||||
gk += (u * x + s) * v[j];
|
||||
s = x + s * w;
|
||||
}
|
||||
_gk[t] = F(gk);
|
||||
}
|
||||
|
||||
for (int t = t_T_1; t >= t_0; t -= C)
|
||||
{
|
||||
__syncthreads();
|
||||
r[i] = float(_r[t]);
|
||||
k[i] = float(_k[t]);
|
||||
w_[i] = exp(_w[t]);
|
||||
__syncthreads();
|
||||
|
||||
const float gyy = float(_gy[t]);
|
||||
float gv = 0;
|
||||
|
||||
#pragma unroll
|
||||
for (int j = 0; j < _N_; j++)
|
||||
{
|
||||
float& s = sdddd[j];
|
||||
float x = gyy * r[j];
|
||||
|
||||
gv += (u_[j] * x + s) * k[j];
|
||||
s = x + s * w_[j];
|
||||
}
|
||||
_gv[t] = F(gv);
|
||||
}
|
||||
}
|
||||
|
||||
template <typename F>
|
||||
__global__ void kernel_backward_222(const int B, const int T, const int C, const int H,
|
||||
const F *__restrict__ const _r, const F *__restrict__ const _k, const F *__restrict__ const _v, const float *__restrict__ _w, const F *__restrict__ _u, const F *__restrict__ const _gy,
|
||||
F *__restrict__ const _gw)
|
||||
{
|
||||
const int b = blockIdx.x / H;
|
||||
const int h = blockIdx.x % H;
|
||||
const int i = threadIdx.x;
|
||||
|
||||
__shared__ float v[_N_], gy[_N_];
|
||||
float saaaa[_N_] = {0}, sbbbb[_T_-2] = {0}, scccc[_N_] = {0};
|
||||
|
||||
const int t_0 = b*T*C + h*_N_ + i;
|
||||
const int t_1 = t_0 + C;
|
||||
const int t_2 = t_0 + 2*C;
|
||||
const int t_T_1 = t_0 + (T-1)*C;
|
||||
|
||||
for (int t = t_T_1; t > t_1; t -= C)
|
||||
{
|
||||
__syncthreads();
|
||||
gy[i] = float(_gy[t]);
|
||||
v[i] = float(_v[t-2*C]);
|
||||
__syncthreads();
|
||||
|
||||
const float r = float(_r[t]);
|
||||
const float w = exp(_w[t-C]);
|
||||
float sum = 0.0f;
|
||||
|
||||
#pragma unroll
|
||||
for (int j = 0; j < _N_; j++)
|
||||
{
|
||||
float& s = saaaa[j];
|
||||
float x = r * gy[j];
|
||||
s = (s + x) * w;
|
||||
sum += s * v[j];
|
||||
}
|
||||
sbbbb[(t-t_2)/C] = sum * float(_k[t-2*C]);
|
||||
}
|
||||
|
||||
float sss = sbbbb[0];
|
||||
_gw[t_0] = 0;
|
||||
_gw[t_1] = F(sss * _w[t_1]);
|
||||
|
||||
for (int t = t_2; t < t_T_1; t += C)
|
||||
{
|
||||
__syncthreads();
|
||||
gy[i] = float(_gy[t]);
|
||||
v[i] = float(_v[t-2*C]);
|
||||
__syncthreads();
|
||||
|
||||
const float w = exp(_w[t-C]);
|
||||
const float k = float(_k[t-2*C]);
|
||||
float sum = 0.0f;
|
||||
|
||||
#pragma unroll
|
||||
for (int j = 0; j < _N_; j++)
|
||||
{
|
||||
float& s = scccc[j];
|
||||
float x = k * v[j];
|
||||
s = (s + x) * w;
|
||||
sum += s * gy[j];
|
||||
}
|
||||
sss += sbbbb[(t-t_1)/C] - (sum * float(_r[t]));
|
||||
_gw[t] = F(sss * _w[t]);
|
||||
}
|
||||
_gw[t_T_1] = 0;
|
||||
}
|
||||
|
||||
void cuda_forward(int B, int T, int C, int H, bf16 *r, bf16 *k, bf16 *v, float *w, bf16 *u, bf16 *y)
|
||||
{
|
||||
assert(H*_N_ == C);
|
||||
assert(_N_%4 == 0);
|
||||
kernel_forward<<<dim3(B * H), dim3(_N_)>>>(B, T, C, H, r, k, v, w, u, y);
|
||||
}
|
||||
|
||||
void cuda_backward(int B, int T, int C, int H, bf16 *r, bf16 *k, bf16 *v, float *w, bf16 *u, bf16 *gy, bf16 *gr, bf16 *gk, bf16 *gv, bf16 *gw, bf16 *gu)
|
||||
{
|
||||
assert(H*_N_ == C);
|
||||
assert(_N_%4 == 0);
|
||||
kernel_backward_111<<<dim3(B * H), dim3(_N_)>>>(B, T, C, H, r, k, v, w, u, gy, gr, gk, gv, gu);
|
||||
kernel_backward_222<<<dim3(B * H), dim3(_N_)>>>(B, T, C, H, r, k, v, w, u, gy, gw);
|
||||
}
|
22
finetune/lora/v6/cuda/wkv6_op.cpp
vendored
Normal file
22
finetune/lora/v6/cuda/wkv6_op.cpp
vendored
Normal file
@ -0,0 +1,22 @@
|
||||
#include <torch/extension.h>
|
||||
#include "ATen/ATen.h"
|
||||
typedef at::BFloat16 bf16;
|
||||
|
||||
void cuda_forward(int B, int T, int C, int H, bf16 *r, bf16 *k, bf16 *v, float *w, bf16 *u, bf16 *y);
|
||||
void cuda_backward(int B, int T, int C, int H, bf16 *r, bf16 *k, bf16 *v, float *w, bf16 *u, bf16 *gy, bf16 *gr, bf16 *gk, bf16 *gv, bf16 *gw, bf16 *gu);
|
||||
|
||||
void forward(int64_t B, int64_t T, int64_t C, int64_t H, torch::Tensor &r, torch::Tensor &k, torch::Tensor &v, torch::Tensor &w, torch::Tensor &u, torch::Tensor &y) {
|
||||
cuda_forward(B, T, C, H, r.data_ptr<bf16>(), k.data_ptr<bf16>(), v.data_ptr<bf16>(), w.data_ptr<float>(), u.data_ptr<bf16>(), y.data_ptr<bf16>());
|
||||
}
|
||||
void backward(int64_t B, int64_t T, int64_t C, int64_t H, torch::Tensor &r, torch::Tensor &k, torch::Tensor &v, torch::Tensor &w, torch::Tensor &u, torch::Tensor &gy, torch::Tensor &gr, torch::Tensor &gk, torch::Tensor &gv, torch::Tensor &gw, torch::Tensor &gu) {
|
||||
cuda_backward(B, T, C, H, r.data_ptr<bf16>(), k.data_ptr<bf16>(), v.data_ptr<bf16>(), w.data_ptr<float>(), u.data_ptr<bf16>(), gy.data_ptr<bf16>(), gr.data_ptr<bf16>(), gk.data_ptr<bf16>(), gv.data_ptr<bf16>(), gw.data_ptr<bf16>(), gu.data_ptr<bf16>());
|
||||
}
|
||||
PYBIND11_MODULE(TORCH_EXTENSION_NAME, m) {
|
||||
m.def("forward", &forward, "wkv6 forward");
|
||||
m.def("backward", &backward, "wkv6 backward");
|
||||
}
|
||||
|
||||
TORCH_LIBRARY(wkv6, m) {
|
||||
m.def("forward", forward);
|
||||
m.def("backward", backward);
|
||||
}
|
311
finetune/lora/v6/cuda/wkv6infctx_cuda.cu
vendored
Normal file
311
finetune/lora/v6/cuda/wkv6infctx_cuda.cu
vendored
Normal file
@ -0,0 +1,311 @@
|
||||
#include <stdio.h>
|
||||
#include <assert.h>
|
||||
#include "ATen/ATen.h"
|
||||
typedef at::BFloat16 bf16;
|
||||
|
||||
template <typename F>
|
||||
__global__ void kernel_forward(const int B, const int T, const int C, const int H,
|
||||
const F *__restrict__ const _r, const F *__restrict__ const _k, const F *__restrict__ const _v, const F *__restrict__ _w, const F *__restrict__ _u, F *__restrict__ _s,
|
||||
F *__restrict__ const _y)
|
||||
{
|
||||
const int b = blockIdx.x / H;
|
||||
const int h = blockIdx.x % H;
|
||||
const int i = threadIdx.x;
|
||||
_u += h*_N_;
|
||||
_s += h*_N_*_N_ + i*_N_;
|
||||
|
||||
__shared__ float r[_N_], k[_N_], u[_N_], w[_N_];
|
||||
float state[_N_];
|
||||
|
||||
__syncthreads();
|
||||
u[i] = float(_u[i]);
|
||||
__syncthreads();
|
||||
for (int j = 0; j < _N_; j++) {
|
||||
state[j] = float(_s[j]);
|
||||
}
|
||||
|
||||
for (int t = b*T*C + h*_N_ + i; t < (b+1)*T*C + h*_N_ + i; t += C)
|
||||
{
|
||||
__syncthreads();
|
||||
w[i] = __expf(-__expf(float(_w[t])));
|
||||
r[i] = float(_r[t]);
|
||||
k[i] = float(_k[t]);
|
||||
__syncthreads();
|
||||
|
||||
const float v = float(_v[t]);
|
||||
float y = 0;
|
||||
|
||||
#pragma unroll
|
||||
for (int j = 0; j < _N_; j+=4)
|
||||
{
|
||||
const float4& r_ = (float4&)(r[j]);
|
||||
const float4& k_ = (float4&)(k[j]);
|
||||
const float4& w_ = (float4&)(w[j]);
|
||||
const float4& u_ = (float4&)(u[j]);
|
||||
float4& s = (float4&)(state[j]);
|
||||
float4 x;
|
||||
|
||||
x.x = k_.x * v;
|
||||
x.y = k_.y * v;
|
||||
x.z = k_.z * v;
|
||||
x.w = k_.w * v;
|
||||
|
||||
y += r_.x * (u_.x * x.x + s.x);
|
||||
y += r_.y * (u_.y * x.y + s.y);
|
||||
y += r_.z * (u_.z * x.z + s.z);
|
||||
y += r_.w * (u_.w * x.w + s.w);
|
||||
|
||||
s.x = s.x * w_.x + x.x;
|
||||
s.y = s.y * w_.y + x.y;
|
||||
s.z = s.z * w_.z + x.z;
|
||||
s.w = s.w * w_.w + x.w;
|
||||
}
|
||||
_y[t] = F(y);
|
||||
}
|
||||
#pragma unroll
|
||||
for (int j = 0; j < _N_; j++)
|
||||
_s[j] = F(state[j]);
|
||||
}
|
||||
|
||||
template <typename F>
|
||||
__global__ void kernel_backward_111(const int B, const int T, const int C, const int H,
|
||||
const F *__restrict__ const _r, const F *__restrict__ const _k, const F *__restrict__ const _v, const F *__restrict__ _w, const F *__restrict__ _u, const F *__restrict__ _s, const F *__restrict__ const _gy,
|
||||
F *__restrict__ const _gr, F *__restrict__ const _gk, F *__restrict__ const _gv, F *__restrict__ const _gu, F *__restrict__ const _gs)
|
||||
{
|
||||
const int b = blockIdx.x / H;
|
||||
const int h = blockIdx.x % H;
|
||||
const int i = threadIdx.x;
|
||||
_u += h*_N_;
|
||||
_s += h*_N_*_N_ + i;
|
||||
|
||||
__shared__ float u_[_N_];
|
||||
__shared__ float r[_N_], k[_N_], v[_N_], w_[_N_], gy[_N_];
|
||||
__syncthreads();
|
||||
u_[i] = float(_u[i]);
|
||||
__syncthreads();
|
||||
|
||||
const float u = u_[i];
|
||||
|
||||
float state[_N_], scccc[_N_] = {0}, sdddd[_N_] = {0}, sssss[_N_] = {0}, swwww[_N_];
|
||||
for (int j = 0; j < _N_; j++) {
|
||||
state[j] = float(_s[j*_N_]);
|
||||
swwww[j] = 1.0;
|
||||
}
|
||||
|
||||
const int t_0 = b*T*C + h*_N_ + i;
|
||||
const int t_T_1 = t_0 + (T-1)*C;
|
||||
const int t_T = t_0 + T*C;
|
||||
|
||||
float gu = 0;
|
||||
for (int t = t_0; t < t_T; t += C)
|
||||
{
|
||||
__syncthreads();
|
||||
v[i] = float(_v[t]);
|
||||
gy[i] = float(_gy[t]);
|
||||
__syncthreads();
|
||||
|
||||
const float k = float(_k[t]);
|
||||
const float w = __expf(-__expf(float(_w[t])));
|
||||
float gr = 0, gu_ = 0;
|
||||
|
||||
#pragma unroll
|
||||
for (int j = 0; j < _N_; j++)
|
||||
{
|
||||
float& s = state[j];
|
||||
float x = k * v[j];
|
||||
|
||||
gr += (u * x + s) * gy[j];
|
||||
gu_ += x * gy[j];
|
||||
s = s * w + x;
|
||||
}
|
||||
_gr[t] = F(gr);
|
||||
gu += float(_r[t]) * gu_;
|
||||
}
|
||||
_gu[b*C + h*_N_ + i] = F(gu);
|
||||
|
||||
for (int t = t_T_1; t >= t_0; t -= C)
|
||||
{
|
||||
__syncthreads();
|
||||
v[i] = float(_v[t]);
|
||||
gy[i] = float(_gy[t]);
|
||||
__syncthreads();
|
||||
|
||||
const float rr = float(_r[t]);
|
||||
const float w = __expf(-__expf(float(_w[t])));
|
||||
float gk = 0;
|
||||
|
||||
#pragma unroll
|
||||
for (int j = 0; j < _N_; j++)
|
||||
{
|
||||
float& s = scccc[j];
|
||||
float x = rr * gy[j];
|
||||
|
||||
gk += (u * x + s) * v[j];
|
||||
s = x + s * w;
|
||||
}
|
||||
_gk[t] = F(gk);
|
||||
}
|
||||
|
||||
for (int t = t_T_1; t >= t_0; t -= C)
|
||||
{
|
||||
__syncthreads();
|
||||
r[i] = float(_r[t]);
|
||||
k[i] = float(_k[t]);
|
||||
w_[i] = __expf(-__expf(float(_w[t])));
|
||||
__syncthreads();
|
||||
|
||||
const float gyy = float(_gy[t]);
|
||||
float gv = 0;
|
||||
|
||||
#pragma unroll
|
||||
for (int j = 0; j < _N_; j++)
|
||||
{
|
||||
float& s = sdddd[j];
|
||||
float x = gyy * r[j];
|
||||
|
||||
gv += (u_[j] * x + s) * k[j];
|
||||
s = x + s * w_[j];
|
||||
}
|
||||
_gv[t] = F(gv);
|
||||
}
|
||||
|
||||
for (int t = t_0; t < t_T; t += C)
|
||||
{
|
||||
__syncthreads();
|
||||
r[i] = float(_r[t]);
|
||||
w_[i] = __expf(-__expf(float(_w[t])));
|
||||
__syncthreads();
|
||||
|
||||
const float gyy = float(_gy[t]);
|
||||
|
||||
#pragma unroll
|
||||
for (int j = 0; j < _N_; j++)
|
||||
{
|
||||
float& w = swwww[j];
|
||||
sssss[j] += gyy * w * r[j];
|
||||
w *= w_[j];
|
||||
}
|
||||
}
|
||||
for (int j = 0; j < _N_; j++)
|
||||
_gs[b*H*_N_*_N_ + h*_N_*_N_ + i*_N_ + j] = F(sssss[j]);
|
||||
}
|
||||
|
||||
template <typename F>
|
||||
__global__ void kernel_backward_222(const int B, const int T, const int C, const int H,
|
||||
const F *__restrict__ const _r, const F *__restrict__ const _k, const F *__restrict__ const _v, const F *__restrict__ _w, const F *__restrict__ _u, const F *__restrict__ _s, const F *__restrict__ const _gy,
|
||||
F *__restrict__ const _gw)
|
||||
{
|
||||
const int b = blockIdx.x / H;
|
||||
const int h = blockIdx.x % H;
|
||||
const int i = threadIdx.x;
|
||||
_s += h*_N_*_N_ + i;
|
||||
|
||||
__shared__ float v[_N_], gy[_N_];
|
||||
float state[_N_], saaaa[_N_] = {0}, sbbbb[_T_-1] = {0}, scccc[_N_] = {0};
|
||||
for (int j = 0; j < _N_; j++) {
|
||||
state[j] = float(_s[j*_N_]);
|
||||
}
|
||||
|
||||
const int t_0 = b*T*C + h*_N_ + i;
|
||||
const int t_1 = t_0 + C;
|
||||
const int t_2 = t_0 + 2*C;
|
||||
const int t_T_1 = t_0 + (T-1)*C;
|
||||
|
||||
for (int t = t_T_1; t > t_1; t -= C)
|
||||
{
|
||||
__syncthreads();
|
||||
gy[i] = float(_gy[t]);
|
||||
v[i] = float(_v[t-2*C]);
|
||||
__syncthreads();
|
||||
|
||||
const float r = float(_r[t]);
|
||||
const float w = __expf(-__expf(float(_w[t-C])));
|
||||
float sum = 0.0f;
|
||||
|
||||
#pragma unroll
|
||||
for (int j = 0; j < _N_; j++)
|
||||
{
|
||||
float& s = saaaa[j];
|
||||
s = (s + r * gy[j]) * w;
|
||||
sum += s * v[j];
|
||||
}
|
||||
sbbbb[(t-t_1)/C] = sum * float(_k[t-2*C]);
|
||||
}
|
||||
{
|
||||
__syncthreads();
|
||||
gy[i] = float(_gy[t_1]);
|
||||
__syncthreads();
|
||||
|
||||
const float r = float(_r[t_1]);
|
||||
const float w = __expf(-__expf(float(_w[t_0])));
|
||||
float sum = 0.0f;
|
||||
|
||||
#pragma unroll
|
||||
for (int j = 0; j < _N_; j++)
|
||||
{
|
||||
float& s = saaaa[j];
|
||||
s = (s + r * gy[j]) * w;
|
||||
sum += s * state[j];
|
||||
}
|
||||
sbbbb[0] = sum;
|
||||
}
|
||||
|
||||
float sss = sbbbb[0];
|
||||
_gw[t_0] = F(sss * -__expf(float(_w[t_0])));
|
||||
|
||||
{
|
||||
__syncthreads();
|
||||
gy[i] = float(_gy[t_1]);
|
||||
__syncthreads();
|
||||
|
||||
const float w = __expf(-__expf(float(_w[t_0])));
|
||||
float sum = 0.0f;
|
||||
|
||||
#pragma unroll
|
||||
for (int j = 0; j < _N_; j++)
|
||||
{
|
||||
float& s = scccc[j];
|
||||
s = (s + state[j]) * w;
|
||||
sum += s * gy[j];
|
||||
}
|
||||
sss += sbbbb[1] - (sum * float(_r[t_1]));
|
||||
_gw[t_1] = F(sss * -__expf(float(_w[t_1])));
|
||||
}
|
||||
for (int t = t_2; t < t_T_1; t += C)
|
||||
{
|
||||
__syncthreads();
|
||||
gy[i] = float(_gy[t]);
|
||||
v[i] = float(_v[t-2*C]);
|
||||
__syncthreads();
|
||||
|
||||
const float w = __expf(-__expf(float(_w[t-C])));
|
||||
const float k = float(_k[t-2*C]);
|
||||
float sum = 0.0f;
|
||||
|
||||
#pragma unroll
|
||||
for (int j = 0; j < _N_; j++)
|
||||
{
|
||||
float& s = scccc[j];
|
||||
s = (s + k * v[j]) * w;
|
||||
sum += s * gy[j];
|
||||
}
|
||||
sss += sbbbb[(t-t_0)/C] - (sum * float(_r[t]));
|
||||
_gw[t] = F(sss * -__expf(float(_w[t])));
|
||||
}
|
||||
_gw[t_T_1] = 0;
|
||||
}
|
||||
|
||||
void cuda_forward(int B, int T, int C, int H, bf16 *r, bf16 *k, bf16 *v, bf16 *w, bf16 *u, bf16 *z, bf16 *y)
|
||||
{
|
||||
assert(H*_N_ == C);
|
||||
assert(_N_%4 == 0);
|
||||
kernel_forward<<<dim3(B * H), dim3(_N_)>>>(B, T, C, H, r, k, v, w, u, z, y);
|
||||
}
|
||||
|
||||
void cuda_backward(int B, int T, int C, int H, bf16 *r, bf16 *k, bf16 *v, bf16 *w, bf16 *u, bf16 *z, bf16 *gy, bf16 *gr, bf16 *gk, bf16 *gv, bf16 *gw, bf16 *gu, bf16 *gs)
|
||||
{
|
||||
assert(H*_N_ == C);
|
||||
assert(_N_%4 == 0);
|
||||
kernel_backward_111<<<dim3(B * H), dim3(_N_)>>>(B, T, C, H, r, k, v, w, u, z, gy, gr, gk, gv, gu, gs);
|
||||
kernel_backward_222<<<dim3(B * H), dim3(_N_)>>>(B, T, C, H, r, k, v, w, u, z, gy, gw);
|
||||
}
|
22
finetune/lora/v6/cuda/wkv6infctx_op.cpp
vendored
Normal file
22
finetune/lora/v6/cuda/wkv6infctx_op.cpp
vendored
Normal file
@ -0,0 +1,22 @@
|
||||
#include <torch/extension.h>
|
||||
#include "ATen/ATen.h"
|
||||
typedef at::BFloat16 bf16;
|
||||
|
||||
void cuda_forward(int B, int T, int C, int H, bf16 *r, bf16 *k, bf16 *v, bf16 *w, bf16 *u, bf16 *s, bf16 *y);
|
||||
void cuda_backward(int B, int T, int C, int H, bf16 *r, bf16 *k, bf16 *v, bf16 *w, bf16 *u, bf16 *s, bf16 *gy, bf16 *gr, bf16 *gk, bf16 *gv, bf16 *gw, bf16 *gu, bf16 *gs);
|
||||
|
||||
void forward(int64_t B, int64_t T, int64_t C, int64_t H, torch::Tensor &r, torch::Tensor &k, torch::Tensor &v, torch::Tensor &w, torch::Tensor &u, torch::Tensor &s, torch::Tensor &y) {
|
||||
cuda_forward(B, T, C, H, r.data_ptr<bf16>(), k.data_ptr<bf16>(), v.data_ptr<bf16>(), w.data_ptr<bf16>(), u.data_ptr<bf16>(), s.data_ptr<bf16>(), y.data_ptr<bf16>());
|
||||
}
|
||||
void backward(int64_t B, int64_t T, int64_t C, int64_t H, torch::Tensor &r, torch::Tensor &k, torch::Tensor &v, torch::Tensor &w, torch::Tensor &u, torch::Tensor &s, torch::Tensor &gy, torch::Tensor &gr, torch::Tensor &gk, torch::Tensor &gv, torch::Tensor &gw, torch::Tensor &gu, torch::Tensor &gs) {
|
||||
cuda_backward(B, T, C, H, r.data_ptr<bf16>(), k.data_ptr<bf16>(), v.data_ptr<bf16>(), w.data_ptr<bf16>(), u.data_ptr<bf16>(), s.data_ptr<bf16>(), gy.data_ptr<bf16>(), gr.data_ptr<bf16>(), gk.data_ptr<bf16>(), gv.data_ptr<bf16>(), gw.data_ptr<bf16>(), gu.data_ptr<bf16>(), gs.data_ptr<bf16>());
|
||||
}
|
||||
PYBIND11_MODULE(TORCH_EXTENSION_NAME, m) {
|
||||
m.def("forward", &forward, "wkv6state forward");
|
||||
m.def("backward", &backward, "wkv6state backward");
|
||||
}
|
||||
|
||||
TORCH_LIBRARY(wkv6state, m) {
|
||||
m.def("forward", forward);
|
||||
m.def("backward", backward);
|
||||
}
|
311
finetune/lora/v6/cuda/wkv6state_cuda.cu
vendored
Normal file
311
finetune/lora/v6/cuda/wkv6state_cuda.cu
vendored
Normal file
@ -0,0 +1,311 @@
|
||||
#include <stdio.h>
|
||||
#include <assert.h>
|
||||
#include "ATen/ATen.h"
|
||||
typedef at::BFloat16 bf16;
|
||||
|
||||
template <typename F>
|
||||
__global__ void kernel_forward(const int B, const int T, const int C, const int H,
|
||||
const F *__restrict__ const _r, const F *__restrict__ const _k, const F *__restrict__ const _v, const F *__restrict__ _w, const F *__restrict__ _u,const F *__restrict__ _s,
|
||||
F *__restrict__ const _y)
|
||||
{
|
||||
const int b = blockIdx.x / H;
|
||||
const int h = blockIdx.x % H;
|
||||
const int i = threadIdx.x;
|
||||
_u += h*_N_;
|
||||
_s += h*_N_*_N_ + i*_N_;
|
||||
|
||||
__shared__ float r[_N_], k[_N_], u[_N_], w[_N_];
|
||||
float state[_N_];
|
||||
|
||||
__syncthreads();
|
||||
u[i] = float(_u[i]);
|
||||
__syncthreads();
|
||||
for (int j = 0; j < _N_; j++) {
|
||||
state[j] = float(_s[j]);
|
||||
}
|
||||
|
||||
for (int t = b*T*C + h*_N_ + i; t < (b+1)*T*C + h*_N_ + i; t += C)
|
||||
{
|
||||
__syncthreads();
|
||||
w[i] = __expf(-__expf(float(_w[t])));
|
||||
r[i] = float(_r[t]);
|
||||
k[i] = float(_k[t]);
|
||||
__syncthreads();
|
||||
|
||||
const float v = float(_v[t]);
|
||||
float y = 0;
|
||||
|
||||
#pragma unroll
|
||||
for (int j = 0; j < _N_; j+=4)
|
||||
{
|
||||
const float4& r_ = (float4&)(r[j]);
|
||||
const float4& k_ = (float4&)(k[j]);
|
||||
const float4& w_ = (float4&)(w[j]);
|
||||
const float4& u_ = (float4&)(u[j]);
|
||||
float4& s = (float4&)(state[j]);
|
||||
float4 x;
|
||||
|
||||
x.x = k_.x * v;
|
||||
x.y = k_.y * v;
|
||||
x.z = k_.z * v;
|
||||
x.w = k_.w * v;
|
||||
|
||||
y += r_.x * (u_.x * x.x + s.x);
|
||||
y += r_.y * (u_.y * x.y + s.y);
|
||||
y += r_.z * (u_.z * x.z + s.z);
|
||||
y += r_.w * (u_.w * x.w + s.w);
|
||||
|
||||
s.x = s.x * w_.x + x.x;
|
||||
s.y = s.y * w_.y + x.y;
|
||||
s.z = s.z * w_.z + x.z;
|
||||
s.w = s.w * w_.w + x.w;
|
||||
}
|
||||
_y[t] = F(y);
|
||||
}
|
||||
// #pragma unroll
|
||||
// for (int j = 0; j < _N_; j++)
|
||||
// _s[j] = F(state[j]);
|
||||
}
|
||||
|
||||
template <typename F>
|
||||
__global__ void kernel_backward_111(const int B, const int T, const int C, const int H,
|
||||
const F *__restrict__ const _r, const F *__restrict__ const _k, const F *__restrict__ const _v, const F *__restrict__ _w, const F *__restrict__ _u, const F *__restrict__ _s, const F *__restrict__ const _gy,
|
||||
F *__restrict__ const _gr, F *__restrict__ const _gk, F *__restrict__ const _gv, F *__restrict__ const _gu, F *__restrict__ const _gs)
|
||||
{
|
||||
const int b = blockIdx.x / H;
|
||||
const int h = blockIdx.x % H;
|
||||
const int i = threadIdx.x;
|
||||
_u += h*_N_;
|
||||
_s += h*_N_*_N_ + i;
|
||||
|
||||
__shared__ float u_[_N_];
|
||||
__shared__ float r[_N_], k[_N_], v[_N_], w_[_N_], gy[_N_];
|
||||
__syncthreads();
|
||||
u_[i] = float(_u[i]);
|
||||
__syncthreads();
|
||||
|
||||
const float u = u_[i];
|
||||
|
||||
float state[_N_], scccc[_N_] = {0}, sdddd[_N_] = {0}, sssss[_N_] = {0}, swwww[_N_];
|
||||
for (int j = 0; j < _N_; j++) {
|
||||
state[j] = float(_s[j*_N_]);
|
||||
swwww[j] = 1.0;
|
||||
}
|
||||
|
||||
const int t_0 = b*T*C + h*_N_ + i;
|
||||
const int t_T_1 = t_0 + (T-1)*C;
|
||||
const int t_T = t_0 + T*C;
|
||||
|
||||
float gu = 0;
|
||||
for (int t = t_0; t < t_T; t += C)
|
||||
{
|
||||
__syncthreads();
|
||||
v[i] = float(_v[t]);
|
||||
gy[i] = float(_gy[t]);
|
||||
__syncthreads();
|
||||
|
||||
const float k = float(_k[t]);
|
||||
const float w = __expf(-__expf(float(_w[t])));
|
||||
float gr = 0, gu_ = 0;
|
||||
|
||||
#pragma unroll
|
||||
for (int j = 0; j < _N_; j++)
|
||||
{
|
||||
float& s = state[j];
|
||||
float x = k * v[j];
|
||||
|
||||
gr += (u * x + s) * gy[j];
|
||||
gu_ += x * gy[j];
|
||||
s = s * w + x;
|
||||
}
|
||||
_gr[t] = F(gr);
|
||||
gu += float(_r[t]) * gu_;
|
||||
}
|
||||
_gu[b*C + h*_N_ + i] = F(gu);
|
||||
|
||||
for (int t = t_T_1; t >= t_0; t -= C)
|
||||
{
|
||||
__syncthreads();
|
||||
v[i] = float(_v[t]);
|
||||
gy[i] = float(_gy[t]);
|
||||
__syncthreads();
|
||||
|
||||
const float rr = float(_r[t]);
|
||||
const float w = __expf(-__expf(float(_w[t])));
|
||||
float gk = 0;
|
||||
|
||||
#pragma unroll
|
||||
for (int j = 0; j < _N_; j++)
|
||||
{
|
||||
float& s = scccc[j];
|
||||
float x = rr * gy[j];
|
||||
|
||||
gk += (u * x + s) * v[j];
|
||||
s = x + s * w;
|
||||
}
|
||||
_gk[t] = F(gk);
|
||||
}
|
||||
|
||||
for (int t = t_T_1; t >= t_0; t -= C)
|
||||
{
|
||||
__syncthreads();
|
||||
r[i] = float(_r[t]);
|
||||
k[i] = float(_k[t]);
|
||||
w_[i] = __expf(-__expf(float(_w[t])));
|
||||
__syncthreads();
|
||||
|
||||
const float gyy = float(_gy[t]);
|
||||
float gv = 0;
|
||||
|
||||
#pragma unroll
|
||||
for (int j = 0; j < _N_; j++)
|
||||
{
|
||||
float& s = sdddd[j];
|
||||
float x = gyy * r[j];
|
||||
|
||||
gv += (u_[j] * x + s) * k[j];
|
||||
s = x + s * w_[j];
|
||||
}
|
||||
_gv[t] = F(gv);
|
||||
}
|
||||
|
||||
for (int t = t_0; t < t_T; t += C)
|
||||
{
|
||||
__syncthreads();
|
||||
r[i] = float(_r[t]);
|
||||
w_[i] = __expf(-__expf(float(_w[t])));
|
||||
__syncthreads();
|
||||
|
||||
const float gyy = float(_gy[t]);
|
||||
|
||||
#pragma unroll
|
||||
for (int j = 0; j < _N_; j++)
|
||||
{
|
||||
float& w = swwww[j];
|
||||
sssss[j] += gyy * w * r[j];
|
||||
w *= w_[j];
|
||||
}
|
||||
}
|
||||
for (int j = 0; j < _N_; j++)
|
||||
_gs[b*H*_N_*_N_ + h*_N_*_N_ + i*_N_ + j] = F(sssss[j]);
|
||||
}
|
||||
|
||||
template <typename F>
|
||||
__global__ void kernel_backward_222(const int B, const int T, const int C, const int H,
|
||||
const F *__restrict__ const _r, const F *__restrict__ const _k, const F *__restrict__ const _v, const F *__restrict__ _w, const F *__restrict__ _u, const F *__restrict__ _s, const F *__restrict__ const _gy,
|
||||
F *__restrict__ const _gw)
|
||||
{
|
||||
const int b = blockIdx.x / H;
|
||||
const int h = blockIdx.x % H;
|
||||
const int i = threadIdx.x;
|
||||
_s += h*_N_*_N_ + i;
|
||||
|
||||
__shared__ float v[_N_], gy[_N_];
|
||||
float state[_N_], saaaa[_N_] = {0}, sbbbb[_T_-1] = {0}, scccc[_N_] = {0};
|
||||
for (int j = 0; j < _N_; j++) {
|
||||
state[j] = float(_s[j*_N_]);
|
||||
}
|
||||
|
||||
const int t_0 = b*T*C + h*_N_ + i;
|
||||
const int t_1 = t_0 + C;
|
||||
const int t_2 = t_0 + 2*C;
|
||||
const int t_T_1 = t_0 + (T-1)*C;
|
||||
|
||||
for (int t = t_T_1; t > t_1; t -= C)
|
||||
{
|
||||
__syncthreads();
|
||||
gy[i] = float(_gy[t]);
|
||||
v[i] = float(_v[t-2*C]);
|
||||
__syncthreads();
|
||||
|
||||
const float r = float(_r[t]);
|
||||
const float w = __expf(-__expf(float(_w[t-C])));
|
||||
float sum = 0.0f;
|
||||
|
||||
#pragma unroll
|
||||
for (int j = 0; j < _N_; j++)
|
||||
{
|
||||
float& s = saaaa[j];
|
||||
s = (s + r * gy[j]) * w;
|
||||
sum += s * v[j];
|
||||
}
|
||||
sbbbb[(t-t_1)/C] = sum * float(_k[t-2*C]);
|
||||
}
|
||||
{
|
||||
__syncthreads();
|
||||
gy[i] = float(_gy[t_1]);
|
||||
__syncthreads();
|
||||
|
||||
const float r = float(_r[t_1]);
|
||||
const float w = __expf(-__expf(float(_w[t_0])));
|
||||
float sum = 0.0f;
|
||||
|
||||
#pragma unroll
|
||||
for (int j = 0; j < _N_; j++)
|
||||
{
|
||||
float& s = saaaa[j];
|
||||
s = (s + r * gy[j]) * w;
|
||||
sum += s * state[j];
|
||||
}
|
||||
sbbbb[0] = sum;
|
||||
}
|
||||
|
||||
float sss = sbbbb[0];
|
||||
_gw[t_0] = F(sss * -__expf(float(_w[t_0])));
|
||||
|
||||
{
|
||||
__syncthreads();
|
||||
gy[i] = float(_gy[t_1]);
|
||||
__syncthreads();
|
||||
|
||||
const float w = __expf(-__expf(float(_w[t_0])));
|
||||
float sum = 0.0f;
|
||||
|
||||
#pragma unroll
|
||||
for (int j = 0; j < _N_; j++)
|
||||
{
|
||||
float& s = scccc[j];
|
||||
s = (s + state[j]) * w;
|
||||
sum += s * gy[j];
|
||||
}
|
||||
sss += sbbbb[1] - (sum * float(_r[t_1]));
|
||||
_gw[t_1] = F(sss * -__expf(float(_w[t_1])));
|
||||
}
|
||||
for (int t = t_2; t < t_T_1; t += C)
|
||||
{
|
||||
__syncthreads();
|
||||
gy[i] = float(_gy[t]);
|
||||
v[i] = float(_v[t-2*C]);
|
||||
__syncthreads();
|
||||
|
||||
const float w = __expf(-__expf(float(_w[t-C])));
|
||||
const float k = float(_k[t-2*C]);
|
||||
float sum = 0.0f;
|
||||
|
||||
#pragma unroll
|
||||
for (int j = 0; j < _N_; j++)
|
||||
{
|
||||
float& s = scccc[j];
|
||||
s = (s + k * v[j]) * w;
|
||||
sum += s * gy[j];
|
||||
}
|
||||
sss += sbbbb[(t-t_0)/C] - (sum * float(_r[t]));
|
||||
_gw[t] = F(sss * -__expf(float(_w[t])));
|
||||
}
|
||||
_gw[t_T_1] = 0;
|
||||
}
|
||||
|
||||
void cuda_forward(int B, int T, int C, int H, bf16 *r, bf16 *k, bf16 *v, bf16 *w, bf16 *u, bf16 *z, bf16 *y)
|
||||
{
|
||||
assert(H*_N_ == C);
|
||||
assert(_N_%4 == 0);
|
||||
kernel_forward<<<dim3(B * H), dim3(_N_)>>>(B, T, C, H, r, k, v, w, u, z, y);
|
||||
}
|
||||
|
||||
void cuda_backward(int B, int T, int C, int H, bf16 *r, bf16 *k, bf16 *v, bf16 *w, bf16 *u, bf16 *z, bf16 *gy, bf16 *gr, bf16 *gk, bf16 *gv, bf16 *gw, bf16 *gu, bf16 *gs)
|
||||
{
|
||||
assert(H*_N_ == C);
|
||||
assert(_N_%4 == 0);
|
||||
kernel_backward_111<<<dim3(B * H), dim3(_N_)>>>(B, T, C, H, r, k, v, w, u, z, gy, gr, gk, gv, gu, gs);
|
||||
kernel_backward_222<<<dim3(B * H), dim3(_N_)>>>(B, T, C, H, r, k, v, w, u, z, gy, gw);
|
||||
}
|
22
finetune/lora/v6/cuda/wkv6state_op.cpp
vendored
Normal file
22
finetune/lora/v6/cuda/wkv6state_op.cpp
vendored
Normal file
@ -0,0 +1,22 @@
|
||||
#include <torch/extension.h>
|
||||
#include "ATen/ATen.h"
|
||||
typedef at::BFloat16 bf16;
|
||||
|
||||
void cuda_forward(int B, int T, int C, int H, bf16 *r, bf16 *k, bf16 *v, bf16 *w, bf16 *u, bf16 *s, bf16 *y);
|
||||
void cuda_backward(int B, int T, int C, int H, bf16 *r, bf16 *k, bf16 *v, bf16 *w, bf16 *u, bf16 *s, bf16 *gy, bf16 *gr, bf16 *gk, bf16 *gv, bf16 *gw, bf16 *gu, bf16 *gs);
|
||||
|
||||
void forward(int64_t B, int64_t T, int64_t C, int64_t H, torch::Tensor &r, torch::Tensor &k, torch::Tensor &v, torch::Tensor &w, torch::Tensor &u, torch::Tensor &s, torch::Tensor &y) {
|
||||
cuda_forward(B, T, C, H, r.data_ptr<bf16>(), k.data_ptr<bf16>(), v.data_ptr<bf16>(), w.data_ptr<bf16>(), u.data_ptr<bf16>(), s.data_ptr<bf16>(), y.data_ptr<bf16>());
|
||||
}
|
||||
void backward(int64_t B, int64_t T, int64_t C, int64_t H, torch::Tensor &r, torch::Tensor &k, torch::Tensor &v, torch::Tensor &w, torch::Tensor &u, torch::Tensor &s, torch::Tensor &gy, torch::Tensor &gr, torch::Tensor &gk, torch::Tensor &gv, torch::Tensor &gw, torch::Tensor &gu, torch::Tensor &gs) {
|
||||
cuda_backward(B, T, C, H, r.data_ptr<bf16>(), k.data_ptr<bf16>(), v.data_ptr<bf16>(), w.data_ptr<bf16>(), u.data_ptr<bf16>(), s.data_ptr<bf16>(), gy.data_ptr<bf16>(), gr.data_ptr<bf16>(), gk.data_ptr<bf16>(), gv.data_ptr<bf16>(), gw.data_ptr<bf16>(), gu.data_ptr<bf16>(), gs.data_ptr<bf16>());
|
||||
}
|
||||
PYBIND11_MODULE(TORCH_EXTENSION_NAME, m) {
|
||||
m.def("forward", &forward, "wkv6state forward");
|
||||
m.def("backward", &backward, "wkv6state backward");
|
||||
}
|
||||
|
||||
TORCH_LIBRARY(wkv6state, m) {
|
||||
m.def("forward", forward);
|
||||
m.def("backward", backward);
|
||||
}
|
16
finetune/lora/v6/demo/demo-lora-merge.sh
vendored
Normal file
16
finetune/lora/v6/demo/demo-lora-merge.sh
vendored
Normal file
@ -0,0 +1,16 @@
|
||||
|
||||
base_model='/home/rwkv/JL/model/rwkv-x060-7b-world-v2.1-36%trained-20240413-ctx4k.pth'
|
||||
lora_init='/home/rwkv/JL/out_model/nf4/init_lora.pth'
|
||||
lora_checkpoint='/home/rwkv/JL/out_model/nf4/rwkv-0.pth'
|
||||
output='/home/rwkv/JL/model/nf4-world.pth'
|
||||
QUANT='nf4' #follow train
|
||||
TYPE='lora'
|
||||
Lora_alpha=128
|
||||
|
||||
python merge/merge.py --base_model $base_model \
|
||||
--lora_init $lora_init \
|
||||
--lora_checkpoint $lora_checkpoint \
|
||||
--output $output \
|
||||
--quant $QUANT \
|
||||
--type $TYPE \
|
||||
--lora_alpha $Lora_alpha
|
27
finetune/lora/v6/demo/demo-lora.sh
vendored
Normal file
27
finetune/lora/v6/demo/demo-lora.sh
vendored
Normal file
@ -0,0 +1,27 @@
|
||||
load_model='/home/rwkv/JL/model/rwkv-x060-7b-world-v2.1-36%trained-20240413-ctx4k.pth'
|
||||
proj_dir='/home/rwkv/JL/out_model/nf4'
|
||||
data_file='/home/rwkv/JL/data/roleplay'
|
||||
|
||||
QUANT='nf4' #4bit nf4 fp4 none
|
||||
|
||||
lora_r=64
|
||||
lora_alpha=128
|
||||
|
||||
n_layer=32
|
||||
n_embd=4096
|
||||
|
||||
micro_bsz=8
|
||||
epoch_save=1
|
||||
epoch_steps=1000
|
||||
ctx_len=1024
|
||||
|
||||
python train.py --load_model $load_model \
|
||||
--proj_dir $proj_dir --data_file $data_file \
|
||||
--data_type binidx --vocab_size 65536 \
|
||||
--ctx_len $ctx_len --epoch_steps $epoch_steps --epoch_count 20 --epoch_begin 0 --epoch_save $epoch_save --micro_bsz $micro_bsz \
|
||||
--n_layer $n_layer --n_embd $n_embd \
|
||||
--pre_ffn 0 --head_qk 0 --lr_init 5e-5 --lr_final 5e-5 --warmup_steps 0 --beta1 0.9 --beta2 0.99 --adam_eps 1e-8 \
|
||||
--accelerator gpu --devices 1 --precision bf16 --strategy deepspeed_stage_1 --grad_cp 1 \
|
||||
--my_testing "x060" \
|
||||
--lora_load rwkv-0 --lora --lora_r $lora_r --lora_alpha $lora_alpha --lora_dropout 0.01 --lora_parts=att,ffn,time,ln \
|
||||
--quant $QUANT
|
15
finetune/lora/v6/demo/demo-pissa-merge.sh
vendored
Normal file
15
finetune/lora/v6/demo/demo-pissa-merge.sh
vendored
Normal file
@ -0,0 +1,15 @@
|
||||
|
||||
|
||||
base_model='/home/rwkv/JL/model/RWKV-x060-World-1B6-v2-20240208-ctx4096.pth'
|
||||
lora_init='/home/rwkv/JL/out_model/nf4/init_lora.pth'
|
||||
lora_checkpoint='/home/rwkv/JL/out_model/nf4/rwkv-0.pth'
|
||||
output='/home/rwkv/JL/model/end-world.pth'
|
||||
QUANT='nf4' #follow train
|
||||
TYPE='pissa'
|
||||
|
||||
python merge/merge.py --base_model $base_model \
|
||||
--lora_init $lora_init \
|
||||
--lora_checkpoint $lora_checkpoint \
|
||||
--output $output \
|
||||
--quant $QUANT \
|
||||
--type $TYPE
|
40
finetune/lora/v6/demo/demo-pissa.sh
vendored
Normal file
40
finetune/lora/v6/demo/demo-pissa.sh
vendored
Normal file
@ -0,0 +1,40 @@
|
||||
|
||||
load_model='/home/rwkv/JL/model/RWKV-x060-World-1B6-v2.1-20240328-ctx4096.pth'
|
||||
proj_dir='/home/rwkv/JL/out_model/nf4'
|
||||
data_file='/home/rwkv/JL/data/end_text_document'
|
||||
|
||||
QUANT='nf4' #4bit nf4 fp4 none
|
||||
svd_niter=4
|
||||
lora_r=64
|
||||
|
||||
n_layer=24
|
||||
n_embd=2048
|
||||
|
||||
micro_bsz=8
|
||||
epoch_save=1
|
||||
epoch_steps=1000
|
||||
ctx_len=1024
|
||||
|
||||
python train.py --load_model $load_model \
|
||||
--proj_dir $proj_dir --data_file $data_file \
|
||||
--data_type binidx --vocab_size 65536 \
|
||||
--ctx_len $ctx_len --epoch_steps $epoch_steps --epoch_count 1 --epoch_begin 0 --epoch_save $epoch_save --micro_bsz $micro_bsz \
|
||||
--n_layer $n_layer --n_embd $n_embd \
|
||||
--pre_ffn 0 --head_qk 0 --lr_init 5e-5 --lr_final 5e-5 --warmup_steps 0 --beta1 0.9 --beta2 0.99 --adam_eps 1e-8 \
|
||||
--accelerator gpu --devices 1 --precision bf16 --strategy deepspeed_stage_1 --grad_cp 1 \
|
||||
--my_testing "x060" \
|
||||
--lora_load rwkv-0 --lora --lora_r $lora_r --lora_alpha 128 --lora_dropout 0.01 --lora_parts=att,ffn,time,ln \
|
||||
--PISSA --svd_niter $svd_niter \
|
||||
--dataload pad
|
||||
|
||||
###remove load_model
|
||||
# python train.py --proj_dir $proj_dir --data_file $data_file \
|
||||
# --data_type binidx --vocab_size 65536 \
|
||||
# --ctx_len $ctx_len --epoch_steps $epoch_steps --epoch_count 20 --epoch_begin 0 --epoch_save $epoch_save --micro_bsz $micro_bsz \
|
||||
# --n_layer $n_layer --n_embd $n_embd \
|
||||
# --pre_ffn 0 --head_qk 0 --lr_init 5e-5 --lr_final 5e-5 --warmup_steps 0 --beta1 0.9 --beta2 0.99 --adam_eps 1e-8 \
|
||||
# --accelerator gpu --devices 1 --precision bf16 --strategy deepspeed_stage_1 --grad_cp 1 \
|
||||
# --my_testing "x060" \
|
||||
# --lora_load rwkv-0 --lora --lora_r $lora_r --lora_alpha 128 --lora_dropout 0.01 --lora_parts=att,ffn,time,ln \
|
||||
# --PISSA --svd_niter $svd_niter \
|
||||
# --quant $QUANT
|
27
finetune/lora/v6/demo/demo-qpissa-pt.sh
vendored
Normal file
27
finetune/lora/v6/demo/demo-qpissa-pt.sh
vendored
Normal file
@ -0,0 +1,27 @@
|
||||
load_model='/home/rwkv/JL/model/rwkv-x060-7b-world-v2.1-36%trained-20240413-ctx4k.pth'
|
||||
proj_dir='/home/rwkv/JL/out_model/nf4'
|
||||
data_file='/home/rwkv/JL/data/roleplay'
|
||||
|
||||
QUANT='nf4' #4bit nf4 fp4 none
|
||||
svd_niter=4
|
||||
lora_r=64
|
||||
|
||||
n_layer=32
|
||||
n_embd=4096
|
||||
|
||||
micro_bsz=4
|
||||
epoch_save=1
|
||||
epoch_steps=1000
|
||||
ctx_len=1024
|
||||
|
||||
|
||||
python train.py --proj_dir $proj_dir --data_file $data_file \
|
||||
--data_type binidx --vocab_size 65536 \
|
||||
--ctx_len $ctx_len --epoch_steps $epoch_steps --epoch_count 20 --epoch_begin 0 --epoch_save $epoch_save --micro_bsz $micro_bsz \
|
||||
--n_layer $n_layer --n_embd $n_embd \
|
||||
--pre_ffn 0 --head_qk 0 --lr_init 5e-5 --lr_final 5e-5 --warmup_steps 0 --beta1 0.9 --beta2 0.99 --adam_eps 1e-8 \
|
||||
--accelerator gpu --devices 1 --precision bf16 --strategy deepspeed_stage_1 --grad_cp 1 \
|
||||
--my_testing "x060" \
|
||||
--lora_load rwkv-0 --lora --lora_r $lora_r --lora_alpha 128 --lora_dropout 0.01 --lora_parts=att,ffn,time,ln \
|
||||
--PISSA --svd_niter $svd_niter \
|
||||
--quant $QUANT
|
8
finetune/lora/v6/demo/demo-state-merge.sh
vendored
Normal file
8
finetune/lora/v6/demo/demo-state-merge.sh
vendored
Normal file
@ -0,0 +1,8 @@
|
||||
base_model='/home/rwkv/JL/model/RWKV-x060-World-3B-v2.1-20240417-ctx4096.pth'
|
||||
state_checkpoint='/home/rwkv/JL/out_model/state/rwkv-9.pth'
|
||||
output='/home/rwkv/JL/model/state-0.pth'
|
||||
|
||||
|
||||
python merge/merge_state.py --base_model $base_model \
|
||||
--state_checkpoint $state_checkpoint \
|
||||
--output $output
|
22
finetune/lora/v6/demo/demo-state-tuning.sh
vendored
Normal file
22
finetune/lora/v6/demo/demo-state-tuning.sh
vendored
Normal file
@ -0,0 +1,22 @@
|
||||
load_model='/home/rwkv/JL/model/RWKV-x060-World-1B6-v2.1-20240328-ctx4096.pth'
|
||||
proj_dir='/home/rwkv/JL/out_model/state'
|
||||
data_file='/home/rwkv/JL/data/end_text_document'
|
||||
|
||||
|
||||
n_layer=24
|
||||
n_embd=2048
|
||||
|
||||
micro_bsz=1
|
||||
epoch_save=1
|
||||
epoch_steps=1000
|
||||
ctx_len=1024
|
||||
|
||||
python train.py --load_model $load_model \
|
||||
--proj_dir $proj_dir --data_file $data_file \
|
||||
--data_type binidx --vocab_size 65536 \
|
||||
--ctx_len $ctx_len --epoch_steps $epoch_steps --epoch_count 1 --epoch_begin 0 --epoch_save $epoch_save --micro_bsz $micro_bsz \
|
||||
--n_layer $n_layer --n_embd $n_embd \
|
||||
--pre_ffn 0 --head_qk 0 --lr_init 1 --lr_final 1e-1 --warmup_steps 0 --beta1 0.9 --beta2 0.99 --adam_eps 1e-8 \
|
||||
--accelerator gpu --devices 1 --precision bf16 --strategy deepspeed_stage_1 --grad_cp 0 \
|
||||
--my_testing "x060" \
|
||||
--train_type "state" --dataload pad --wandb fla --fla
|
27
finetune/lora/v6/demo/demo-training-prepare.sh
vendored
Normal file
27
finetune/lora/v6/demo/demo-training-prepare.sh
vendored
Normal file
@ -0,0 +1,27 @@
|
||||
#!/bin/bash
|
||||
|
||||
# Create data directory
|
||||
|
||||
mkdir -p data
|
||||
|
||||
# Download minipile (1498226207 tokens, around 3GB)
|
||||
|
||||
wget --continue -O data/minipile.idx https://huggingface.co/datasets/BlinkDL/minipile-tokenized/resolve/main/rwkv_vocab_v20230424/minipile.idx
|
||||
wget --continue -O data/minipile.bin https://huggingface.co/datasets/BlinkDL/minipile-tokenized/resolve/main/rwkv_vocab_v20230424/minipile.bin
|
||||
|
||||
# Generate initial model (L12-D768 = 169M)
|
||||
|
||||
BASE_NAME="model/0.1-1"
|
||||
N_LAYER="12"
|
||||
N_EMBD="768"
|
||||
|
||||
# magic_prime = the largest 3n+2 prime smaller than datalen/ctxlen-1 (= 1498226207/512-1 = 2926222.06 in this case)
|
||||
# use https://www.dcode.fr/prime-numbers-search
|
||||
|
||||
python train.py --wandb "" --proj_dir $BASE_NAME \
|
||||
--data_file "data/minipile" --data_type "binidx" --vocab_size 65536 \
|
||||
--ctx_len 512 --my_pile_stage 1 --epoch_count 1 --epoch_begin 0 \
|
||||
--epoch_save 1 --weight_decay 0 --head_size_a 64 \
|
||||
--num_nodes 1 --micro_bsz 1 --n_layer $N_LAYER --n_embd $N_EMBD --pre_ffn 0 --head_qk 0 --my_exit_tokens 1498226207 --magic_prime 2926181 \
|
||||
--lr_init 1e-5 --lr_final 1e-5 --warmup_steps 10 --beta1 0.9 --beta2 0.99 --adam_eps 1e-8 --my_pile_edecay 0 \
|
||||
--accelerator cpu --devices 1 --precision bf16 --strategy deepspeed_stage_2 --grad_cp 0 --enable_progress_bar False --ds_bucket_mb 200
|
21
finetune/lora/v6/demo/demo-training-run.sh
vendored
Normal file
21
finetune/lora/v6/demo/demo-training-run.sh
vendored
Normal file
@ -0,0 +1,21 @@
|
||||
#!/bin/bash
|
||||
|
||||
BASE_NAME="model/0.1-1"
|
||||
N_LAYER="12"
|
||||
N_EMBD="768"
|
||||
M_BSZ="16" # takes 16G VRAM (reduce this to save VRAM)
|
||||
LR_INIT="6e-4"
|
||||
LR_FINAL="6e-5"
|
||||
GRAD_CP=0 # set to 1 to save VRAM (will be slower)
|
||||
EPOCH_SAVE=10
|
||||
|
||||
# magic_prime = the largest 3n+2 prime smaller than datalen/ctxlen-1 (= 1498226207/512-1 = 2926222.06 in this case)
|
||||
# use https://www.dcode.fr/prime-numbers-search
|
||||
|
||||
python train.py --load_model "0" --wandb "RWKV-5-Test" --proj_dir $BASE_NAME \
|
||||
--ctx_len 512 --my_pile_stage 3 --epoch_count 999999 --epoch_begin 0 \
|
||||
--data_file "data/minipile" --my_exit_tokens 1498226207 --magic_prime 2926181 \
|
||||
--num_nodes 1 --micro_bsz $M_BSZ --n_layer $N_LAYER --n_embd $N_EMBD --pre_ffn 0 --head_qk 0 \
|
||||
--lr_init $LR_INIT --lr_final $LR_FINAL --warmup_steps 10 --beta1 0.9 --beta2 0.99 --adam_eps 1e-8 --my_pile_edecay 0 --data_type "binidx" --vocab_size 65536 \
|
||||
--weight_decay 0.001 --epoch_save $EPOCH_SAVE --head_size_a 64 \
|
||||
--accelerator gpu --devices 1 --precision bf16 --strategy deepspeed_stage_2 --grad_cp $GRAD_CP --enable_progress_bar True --ds_bucket_mb 200
|
182
finetune/lora/v6/demo/demo.jsonl
vendored
Normal file
182
finetune/lora/v6/demo/demo.jsonl
vendored
Normal file
File diff suppressed because one or more lines are too long
25
finetune/lora/v6/demo/infctx.sh
vendored
Normal file
25
finetune/lora/v6/demo/infctx.sh
vendored
Normal file
@ -0,0 +1,25 @@
|
||||
load_model='/home/rwkv/JL/model/RWKV-x060-World-1B6-v2.1-20240328-ctx4096.pth'
|
||||
proj_dir='/home/rwkv/JL/out_model/infctx'
|
||||
data_file='/home/rwkv/JL/data/roleplay'
|
||||
|
||||
|
||||
n_layer=24
|
||||
n_embd=2048
|
||||
|
||||
micro_bsz=8
|
||||
epoch_save=5
|
||||
epoch_steps=1000
|
||||
ctx_len=16384
|
||||
chunk_ctx=2048
|
||||
|
||||
|
||||
python train.py --load_model $load_model \
|
||||
--proj_dir $proj_dir --data_file $data_file \
|
||||
--data_type binidx --vocab_size 65536 \
|
||||
--ctx_len $ctx_len --epoch_steps $epoch_steps --epoch_count 1 --epoch_begin 0 --epoch_save $epoch_save --micro_bsz $micro_bsz \
|
||||
--n_layer $n_layer --n_embd $n_embd \
|
||||
--pre_ffn 0 --head_qk 0 --lr_init 1e-4 --lr_final 1e-4 --warmup_steps 0 --beta1 0.9 --beta2 0.99 --adam_eps 1e-8 \
|
||||
--accelerator gpu --devices 1 --precision bf16 --strategy deepspeed_stage_1 --grad_cp 1 \
|
||||
--lora_load rwkv-0 --lora --lora_r 64 --lora_alpha 128 --lora_dropout 0.01 --lora_parts=att,ffn,time,ln \
|
||||
--my_testing "x060" --dataload pad \
|
||||
--train_type infctx --chunk_ctx $chunk_ctx --fla --wandb infctx
|
50
finetune/lora/v6/fla/__init__.py
vendored
Normal file
50
finetune/lora/v6/fla/__init__.py
vendored
Normal file
@ -0,0 +1,50 @@
|
||||
# -*- coding: utf-8 -*-
|
||||
|
||||
from fla.layers import (ABCAttention, BasedLinearAttention, DeltaNet,
|
||||
GatedLinearAttention, HGRN2Attention, LinearAttention,
|
||||
MultiScaleRetention, ReBasedLinearAttention)
|
||||
from fla.models import (ABCForCausalLM, ABCModel, DeltaNetForCausalLM,
|
||||
DeltaNetModel, GLAForCausalLM, GLAModel,
|
||||
HGRN2ForCausalLM, HGRN2Model, HGRNForCausalLM,
|
||||
HGRNModel, LinearAttentionForCausalLM,
|
||||
LinearAttentionModel, RetNetForCausalLM, RetNetModel,
|
||||
RWKV6ForCausalLM, RWKV6Model, TransformerForCausalLM,
|
||||
TransformerModel)
|
||||
from fla.ops import (chunk_gla, chunk_retention, fused_chunk_based,
|
||||
fused_chunk_gla, fused_chunk_retention)
|
||||
|
||||
__all__ = [
|
||||
'ABCAttention',
|
||||
'BasedLinearAttention',
|
||||
'DeltaNet',
|
||||
'HGRN2Attention',
|
||||
'GatedLinearAttention',
|
||||
'LinearAttention',
|
||||
'MultiScaleRetention',
|
||||
'ReBasedLinearAttention',
|
||||
'ABCForCausalLM',
|
||||
'ABCModel',
|
||||
'DeltaNetForCausalLM',
|
||||
'DeltaNetModel',
|
||||
'HGRNForCausalLM',
|
||||
'HGRNModel',
|
||||
'HGRN2ForCausalLM',
|
||||
'HGRN2Model',
|
||||
'GLAForCausalLM',
|
||||
'GLAModel',
|
||||
'LinearAttentionForCausalLM',
|
||||
'LinearAttentionModel',
|
||||
'RetNetForCausalLM',
|
||||
'RetNetModel',
|
||||
'RWKV6ForCausalLM',
|
||||
'RWKV6Model',
|
||||
'TransformerForCausalLM',
|
||||
'TransformerModel',
|
||||
'chunk_gla',
|
||||
'chunk_retention',
|
||||
'fused_chunk_based',
|
||||
'fused_chunk_gla',
|
||||
'fused_chunk_retention'
|
||||
]
|
||||
|
||||
__version__ = '0.1'
|
25
finetune/lora/v6/fla/layers/__init__.py
vendored
Normal file
25
finetune/lora/v6/fla/layers/__init__.py
vendored
Normal file
@ -0,0 +1,25 @@
|
||||
# -*- coding: utf-8 -*-
|
||||
|
||||
from .abc import ABCAttention
|
||||
from .based import BasedLinearAttention
|
||||
from .delta_net import DeltaNet
|
||||
from .gla import GatedLinearAttention
|
||||
from .hgrn import HGRNAttention
|
||||
from .hgrn2 import HGRN2Attention
|
||||
from .linear_attn import LinearAttention
|
||||
from .multiscale_retention import MultiScaleRetention
|
||||
from .rebased import ReBasedLinearAttention
|
||||
from .rwkv6 import RWKV6Attention
|
||||
|
||||
__all__ = [
|
||||
'ABCAttention',
|
||||
'BasedLinearAttention',
|
||||
'DeltaNet',
|
||||
'GatedLinearAttention',
|
||||
'HGRNAttention',
|
||||
'HGRN2Attention',
|
||||
'LinearAttention',
|
||||
'MultiScaleRetention',
|
||||
'ReBasedLinearAttention',
|
||||
'RWKV6Attention'
|
||||
]
|
Some files were not shown because too many files have changed in this diff Show More
Loading…
x
Reference in New Issue
Block a user